- お役立ち記事
- Big Data for Manufacturing: Building a Data Lake and Analytics Infrastructure
Big Data for Manufacturing: Building a Data Lake and Analytics Infrastructure
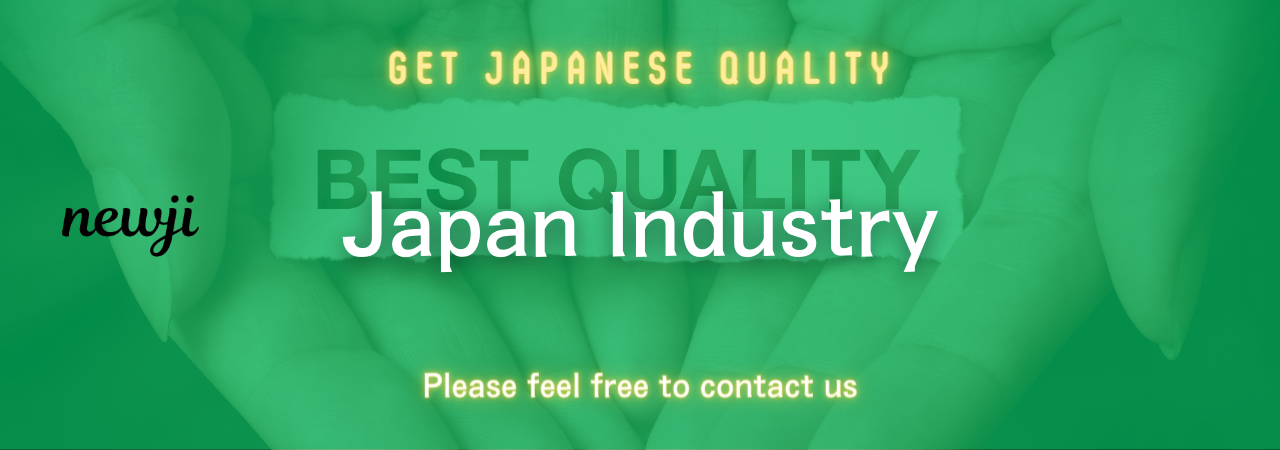
The world of manufacturing is constantly evolving, with advancements in technology playing a critical role in driving efficiency, reducing costs, and improving product quality.
One of the key technological innovations making waves in this sector is big data.
In particular, the creation of data lakes and analytics infrastructures are fundamental components that can help manufacturers harness the power of big data.
This article delves into big data for manufacturing, exploring the steps to build a data lake and effective analytics infrastructure.
目次
Understanding Big Data in Manufacturing
Big data refers to the vast and complex sets of data that can now be gathered, stored, and analyzed to reveal patterns, trends, and associations, particularly relating to human behavior and interactions.
In the manufacturing context, big data can come from a variety of sources including sensors on the production line, machinery analytics, supply chain data, and customer feedback.
These large sets of data, when analyzed, provide invaluable insights that can significantly enhance the manufacturing process.
The Importance of Big Data in Manufacturing
Big data in manufacturing helps in optimizing operations by predicting equipment failures, reducing downtime, and improving supply chain efficiency.
It also plays a crucial role in product development, quality control, and customer satisfaction.
For instance, predictive maintenance enabled by big data analytics can forecast machinery breakdowns before they occur, thereby saving costs on emergency repairs and reducing operational downtime.
Additionally, analyzing customer feedback helps in the continuous improvement of products to meet consumer needs better.
Building a Data Lake
A data lake is a centralized repository that allows you to store all your structured and unstructured data at any scale.
You can store your data as-is, without having to first structure the data, and run different types of analytics—from dashboards and visualizations to big data processing, real-time analytics, and machine learning—to guide better decisions.
Steps to Establish a Data Lake
1. Data Collection
The first step is accumulating data from various sources.
In a manufacturing context, this could include data from sensors, machinery, suppliers, and customers.
Ensure that you set up mechanisms to collect data in real-time to provide up-to-date information for analysis.
2. Storage Solutions
Next, determine the type of storage solution that best fits your needs.
Cloud storage options, like Amazon S3, are popular for their scalability and reliability.
On-premises servers are another option, although they can be more costly.
3. Data Ingestion
Data ingestion involves moving data from its original source to the data lake.
This can be achieved through batch processing or real-time streaming.
Tools like Apache Kafka or AWS Glue can facilitate this process.
4. Data Governance
Implement strong data governance measures to ensure data quality, security, and compliance.
This includes setting up policies for data access, ensuring data integrity, and adhering to industry regulations.
Setting Up Analytics Infrastructure
Once your data lake is set up, the next step is to create an analytics infrastructure.
This infrastructure will enable you to derive actionable insights from your data.
Steps to Develop an Effective Analytics Infrastructure
1. Data Processing
Begin with data processing, which involves cleaning, transforming, and organizing data for analysis.
Tools like Apache Spark or Hadoop can be incredibly efficient for these tasks.
2. Analytics Tools
Choose the right set of analytics tools depending on your needs.
For example, tools like Tableau or Power BI are excellent for visualization and reporting, while Python and R are powerful for more advanced analytics and machine learning.
3. Machine Learning Models
Implementing machine learning models can provide more advanced predictive capabilities.
These models can analyze historical data to make future predictions. For example, they can forecast demand, predict equipment failures, or optimize supply chains.
4. Real-Time Analytics
Real-time analytics can give you immediate insights into ongoing processes.
Technologies like Apache Flink can process data in real time, allowing you to make quick, informed decisions.
Integrating Insights into Operations
After setting up your data lake and analytics infrastructure, it’s essential to integrate these insights into your manufacturing operations.
1. Decision-Making
Use the insights derived from your analytics to inform strategic decision-making.
This could relate to production schedules, supply chain management, or product development.
2. Operational Efficiency
Implement changes based on predictive analytics to enhance operational efficiency.
For instance, maintenance schedules can be optimized based on predictive maintenance insights, minimizing unplanned downtime.
3. Quality Control
Use data analytics to monitor and improve product quality.
This can involve analyzing defect data and implementing corrective measures to prevent future occurrences.
4. Customer Feedback
Analyze customer feedback to understand product performance and areas for improvement.
This helps in ensuring that your products meet customer expectations and enhancing overall satisfaction.
Conclusion
Big data is revolutionizing the manufacturing sector by providing valuable insights that drive efficiency, reduce costs, and improve product quality.
Building a data lake and analytics infrastructure is crucial for harnessing the power of big data.
By following the steps outlined in this article, manufacturers can create a robust system that enhances their decision-making, operational efficiency, and customer satisfaction.
As technology continues to evolve, the importance of big data in manufacturing will only continue to grow, making it imperative for businesses to adopt these advanced data solutions.
資料ダウンロード
QCD調達購買管理クラウド「newji」は、調達購買部門で必要なQCD管理全てを備えた、現場特化型兼クラウド型の今世紀最高の購買管理システムとなります。
ユーザー登録
調達購買業務の効率化だけでなく、システムを導入することで、コスト削減や製品・資材のステータス可視化のほか、属人化していた購買情報の共有化による内部不正防止や統制にも役立ちます。
NEWJI DX
製造業に特化したデジタルトランスフォーメーション(DX)の実現を目指す請負開発型のコンサルティングサービスです。AI、iPaaS、および先端の技術を駆使して、製造プロセスの効率化、業務効率化、チームワーク強化、コスト削減、品質向上を実現します。このサービスは、製造業の課題を深く理解し、それに対する最適なデジタルソリューションを提供することで、企業が持続的な成長とイノベーションを達成できるようサポートします。
オンライン講座
製造業、主に購買・調達部門にお勤めの方々に向けた情報を配信しております。
新任の方やベテランの方、管理職を対象とした幅広いコンテンツをご用意しております。
お問い合わせ
コストダウンが利益に直結する術だと理解していても、なかなか前に進めることができない状況。そんな時は、newjiのコストダウン自動化機能で大きく利益貢献しよう!
(Β版非公開)