- お役立ち記事
- For new employees in the R&D department! Interpretation and application of experimental data using Bayesian statistics
For new employees in the R&D department! Interpretation and application of experimental data using Bayesian statistics
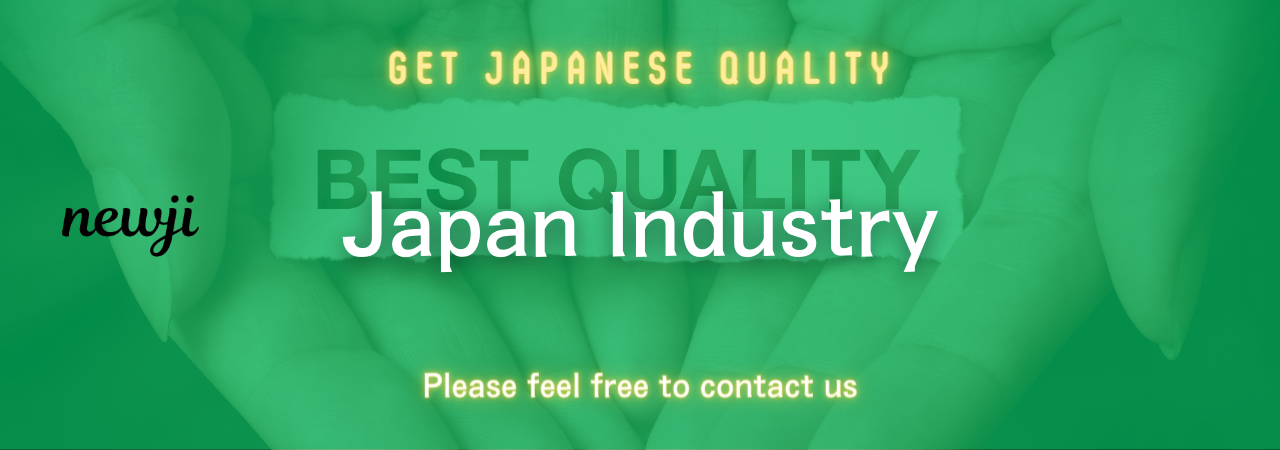
目次
Understanding Bayesian Statistics
When you first step into the world of research and development (R&D), understanding complex statistical methods can seem daunting.
One of the powerful tools you will encounter is Bayesian statistics.
This approach, named after Thomas Bayes, provides a robust framework for interpreting and applying experimental data.
Bayesian statistics is different from traditional, or frequentist, statistics in its approach.
While frequentist statistics looks at probability as a frequency or proportion of times an event occurs, Bayesian statistics views probability as a degree of belief or certainty about an event.
This means it not only considers the data you have, but also incorporates prior information or beliefs into the analysis.
As you get more data, Bayesian methods allow these beliefs to be updated, leading to better decision-making over time.
Why Use Bayesian Statistics in R&D?
Bayesian statistics is particularly useful in the R&D context for several reasons.
First, it allows for the integration of prior knowledge, which can be vital in research fields where existing information can enhance new insights.
Second, with new data, Bayesian analysis helps in continuously updating results – much like learning and refining hypotheses.
Another advantage is its flexibility and applicability to a wide range of models.
Whether the experiments involve drug effectiveness testing or engineering simulations, Bayesian methods can cater to diverse applications.
This makes it an invaluable tool for projects with complex data structures and hierarchical modeling requirements.
Moreover, Bayesian statistics offers intuitive and meaningful probability statements about parameters of interest, which can be directly communicated and understood by non-statistical stakeholders.
For new employees, this ability to convey results clearly can be crucial in cross-disciplinary teams.
Key Concepts in Bayesian Statistics
Before diving into data analysis using Bayesian methods, it’s essential to familiarize yourself with some key concepts:
Priors
Priors represent existing beliefs before considering the current data.
Choosing an appropriate prior is critical, as it influences the results and how you interpret them.
There are different types of priors, such as non-informative priors (which assume no prior knowledge) or informative priors (based on previous studies or expert opinion).
In R&D, understanding the context and industry standards can help you select suitable priors for effective data analysis.
Likelihood
The likelihood function describes how probable your data is, given certain parameters.
It combines with the prior to form the posterior distribution, the updated belief about parameters after considering the data when using Bayesian inference.
Working in R&D means you need to carefully assess experimental design and potential variations that can affect likelihood calculations.
Posterior
The posterior distribution delivers updated beliefs about parameters once both data and priors have been taken into account.
In essence, it is the crux of Bayesian inference, where all information blends to form the best possible understanding of the phenomenon being studied.
Throughout a project, posteriors support informed decision-making, whether assessing experimental results or making predictions.
Applications of Bayesian Statistics in Experimental Data Interpretation
Now that we have explored some basic concepts, let’s discuss how Bayesian analysis applies to the interpretation of experimental data in the R&D landscape.
Model Testing and Selection
Bayesian statistics can help evaluate competing hypotheses more effectively by analyzing how well different models explain the observed data.
It provides various criteria, such as the Bayes factor, to quantify the evidence supporting one hypothesis over another.
For R&D teams, this helps streamline the selection process and ensures resources are focused on promising research avenues.
Uncertainty Quantification
Understanding and quantifying uncertainty is a core part of R&D.
Bayesian statistics are particularly strong in this area, as they provide probability distributions that capture uncertainty about parameters and potential outcomes.
In practice, this means you can make more confident decisions on the reliability and robustness of results while considering possible sources of error.
Predictive Modeling
Bayesian methods are also vital in building predictive models based on experimental data.
They allow you to generate probabilistic predictions, which acknowledge uncertainties and give a comprehensive view of future events.
From drug development to environmental science, predictive modeling helps R&D teams make evidence-based projections crucial for strategic planning.
Best Practices for Implementing Bayesian Methods
To make the most of Bayesian statistics in your R&D role, here are some best practices:
Start with a Simple Model
When learning Bayesian methods, begin with simple models before moving to complex hierarchical structures.
As you build confidence, you can tackle models with multiple parameters and intricate dependencies without getting overwhelmed.
Use Computational Tools
Leverage computational software and tools designed for Bayesian analysis, such as R or Python with libraries like Stan, PyMC3, or JAGS.
These tools facilitate model fitting and posterior sampling, making it easier to apply Bayesian methods in real-world scenarios.
Communicate Results Effectively
Don’t just rely on mathematical descriptions; use visualizations like credible intervals, posterior distributions, and decision trees to communicate results clearly.
This enhances understanding across diverse R&D teams, accelerating collaboration and innovation.
By interpreting and applying experimental data using Bayesian statistics, new employees in the R&D department can drive more accurate and insightful research outcomes.
Embrace these techniques, and you will enhance your ability to contribute meaningfully to your team’s success.
資料ダウンロード
QCD調達購買管理クラウド「newji」は、調達購買部門で必要なQCD管理全てを備えた、現場特化型兼クラウド型の今世紀最高の購買管理システムとなります。
ユーザー登録
調達購買業務の効率化だけでなく、システムを導入することで、コスト削減や製品・資材のステータス可視化のほか、属人化していた購買情報の共有化による内部不正防止や統制にも役立ちます。
NEWJI DX
製造業に特化したデジタルトランスフォーメーション(DX)の実現を目指す請負開発型のコンサルティングサービスです。AI、iPaaS、および先端の技術を駆使して、製造プロセスの効率化、業務効率化、チームワーク強化、コスト削減、品質向上を実現します。このサービスは、製造業の課題を深く理解し、それに対する最適なデジタルソリューションを提供することで、企業が持続的な成長とイノベーションを達成できるようサポートします。
オンライン講座
製造業、主に購買・調達部門にお勤めの方々に向けた情報を配信しております。
新任の方やベテランの方、管理職を対象とした幅広いコンテンツをご用意しております。
お問い合わせ
コストダウンが利益に直結する術だと理解していても、なかなか前に進めることができない状況。そんな時は、newjiのコストダウン自動化機能で大きく利益貢献しよう!
(Β版非公開)