- お役立ち記事
- For new employees in the R&D department! Basics and practical methods of process optimization using response surface methodology
For new employees in the R&D department! Basics and practical methods of process optimization using response surface methodology
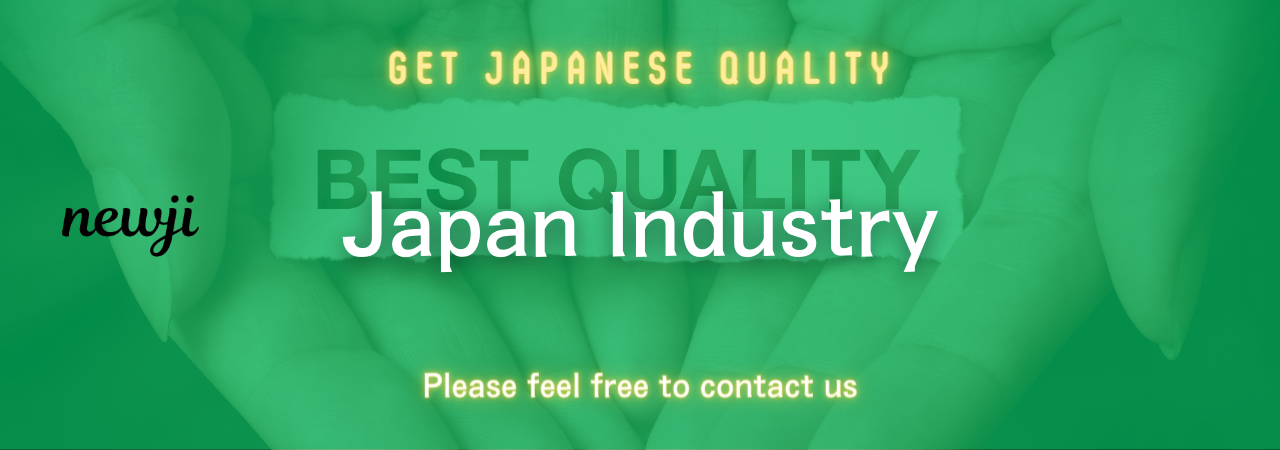
目次
Understanding Process Optimization
Joining the R&D department brings exciting opportunities to innovate and enhance processes, ensuring they operate at their fullest potential.
Process optimization is a systematic approach to improving efficiency and effectiveness, reducing costs, and maximizing desired outputs.
In the world of research and development, this means turning theoretical concepts into tangible results while maintaining quality and reliability.
Optimization involves fine-tuning variables, such as temperature, pressure, and time, to achieve optimal results.
Each process may have a different set of variables that need adjustment, requiring a strategy to identify and manipulate these factors.
Response Surface Methodology (RSM) is one such indispensable tool that helps navigate this complex landscape.
Introduction to Response Surface Methodology (RSM)
RSM is a collection of mathematical and statistical techniques useful for modeling and analyzing problems where several variables influence the output.
It helps in developing, improving, and optimizing processes.
This technique is particularly valuable when the relationship between the response variables and the independent variables is complex and nonlinear.
RSM involves designing experiments to acquire data, building models, evaluating the effect of variables, and exploring optimal conditions.
It provides a graphical representation of the response as a surface over a plane defined by the independent variables, facilitating better understanding and decision-making.
Components of RSM
1. **Design of Experiments (DOE):** The foundation of RSM is the design of experiments.
DOE involves planning and executing tests systematically to gather meaningful data.
Common designs used in RSM include Central Composite Design (CCD) and Box-Behnken Design (BBD).
2. **Building a Model:** After collecting data through experiments, a mathematical model is constructed.
This predictive model maps the relationship between variables and the response, often using polynomial equations.
3. **Analysis and Optimization:** The model allows us to predict and analyze the effects of varying inputs.
Optimization can then be performed using graphical methods like contour plots or numerical approaches such as gradient descent.
Practical Steps for RSM Implementation
Let’s explore practical steps to carry out RSM in your projects effectively.
Step 1: Define the Problem
Begin by understanding the process you want to optimize.
Identify response variables you wish to improve or control.
Clearly define the constraints and objectives.
An example could be improving yield in a chemical reaction while reducing energy consumption.
Step 2: Select Factors and Levels
Choose the factors (independent variables) that significantly affect the response.
For each factor, decide the levels you will test, typically aiming for a range that covers the operating conditions of interest.
A common approach is to use coded levels, such as -1 for low, 0 for medium, and +1 for high values.
Step 3: Design the Experiment
Select an experimental design, such as CCD or BBD, that suits your problem.
Plan a series of experiments that ensure comprehensive coverage of the variable space.
This includes conducting trials at different combinations of factor levels.
Step 4: Conduct Experiments
Perform the experiments diligently, following the designed plan.
Ensure consistency in experimental conditions and accurate data logging.
This step is crucial, as the quality of the results depends heavily on the precision and reliability of the data collected.
Step 5: Build the Model
With the experimental data in hand, use statistical software to fit a model.
Typically, response surface models use regression techniques to fit a polynomial equation.
Verify the model’s adequacy by checking statistical parameters such as R-squared and residual analysis.
Step 6: Analyze and Optimize
Use the model to explore the response surface and find optimal conditions.
Contour plots and 3D surface plots can help visualize the interactions between variables.
Engage in a sensitivity analysis to understand how tweaks to different factors impact the response.
Step 7: Validate the Model
Conduct confirmation experiments to validate the optimizations.
Ensure the predicted outcomes align with experimental results.
Adjust the model if necessary, based on these findings.
Practical Tips for New Employees
As a new employee in R&D, the following tips will help you successfully apply RSM for process optimization:
1. **Invest Time in Understanding Fundamentals:** Familiarize yourself with the basic concepts of statistical analysis and experimental design.
This foundational knowledge will empower you to make informed decisions throughout the optimization process.
2. **Leverage Software Tools:** Utilize software packages like Minitab or Design-Expert, which are designed to assist in DOE and RSM.
These tools provide user-friendly interfaces and automate many analysis steps, making your task more manageable.
3. **Collaborate with Experienced Team Members:** Engage with colleagues who have prior experience in process optimization.
Their insights can provide practical guidance and help you avoid common pitfalls.
4. **Focus on Precise Data Collection:** Data integrity is critical for reliable models.
Implement robust data collection practices and ensure experimental conditions are consistently controlled.
5. **Stay Curious and Iterative:** Be open to experimentation and learning.
Optimization is often an iterative process that requires continual refinement.
6. **Communicate Findings Clearly:** Articulate your process and results succinctly to stakeholders.
Use visuals and easy-to-understand language to present complex data.
By following these guidelines and utilizing Response Surface Methodology, you’ll be well-equipped to contribute to process improvements in the R&D department.
Your efforts will help in enhancing product quality, reducing costs, and ultimately driving innovation.
資料ダウンロード
QCD調達購買管理クラウド「newji」は、調達購買部門で必要なQCD管理全てを備えた、現場特化型兼クラウド型の今世紀最高の購買管理システムとなります。
ユーザー登録
調達購買業務の効率化だけでなく、システムを導入することで、コスト削減や製品・資材のステータス可視化のほか、属人化していた購買情報の共有化による内部不正防止や統制にも役立ちます。
NEWJI DX
製造業に特化したデジタルトランスフォーメーション(DX)の実現を目指す請負開発型のコンサルティングサービスです。AI、iPaaS、および先端の技術を駆使して、製造プロセスの効率化、業務効率化、チームワーク強化、コスト削減、品質向上を実現します。このサービスは、製造業の課題を深く理解し、それに対する最適なデジタルソリューションを提供することで、企業が持続的な成長とイノベーションを達成できるようサポートします。
オンライン講座
製造業、主に購買・調達部門にお勤めの方々に向けた情報を配信しております。
新任の方やベテランの方、管理職を対象とした幅広いコンテンツをご用意しております。
お問い合わせ
コストダウンが利益に直結する術だと理解していても、なかなか前に進めることができない状況。そんな時は、newjiのコストダウン自動化機能で大きく利益貢献しよう!
(Β版非公開)