- お役立ち記事
- Effective use of design of experiments using orthogonal arrays that mid-level employees in design departments should know
Effective use of design of experiments using orthogonal arrays that mid-level employees in design departments should know
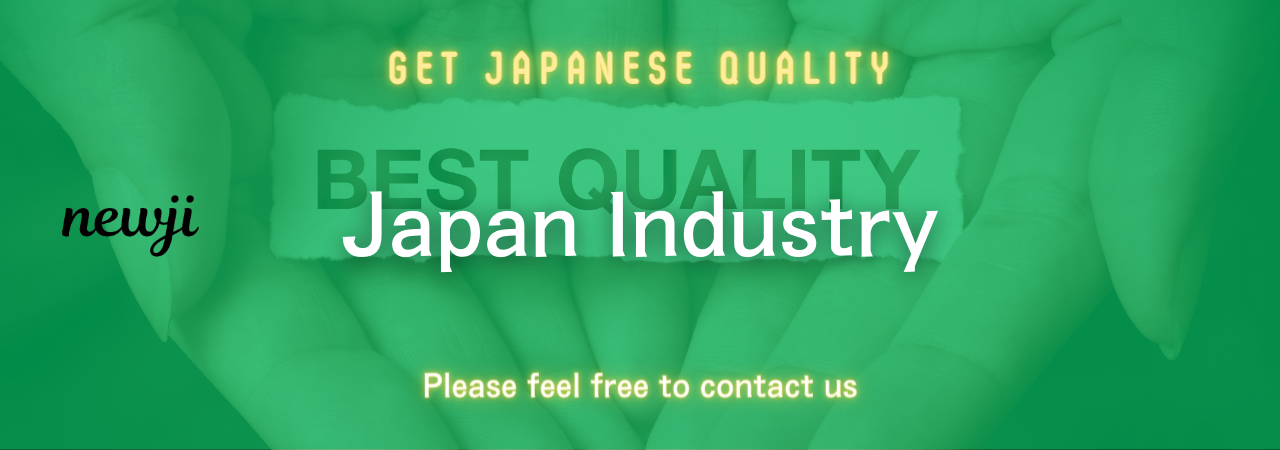
目次
Understanding Design of Experiments (DOE)
Design of Experiments (DOE) is a powerful statistical method used to determine the effect of multiple variables on a particular outcome.
It is widely applicable across various industries but is especially relevant in design departments where products and processes need to be optimized.
For mid-level employees, DOE can be an instrumental tool in refining designs and improving efficiencies.
What Are Orthogonal Arrays?
Orthogonal arrays are a specific type of experimental design that simplifies the testing process.
They allow you to study a large number of variables with a minimum number of experiments.
This is achieved by systematically varying each factor in your process, rather than testing them one at a time, which can be impractical or resource-intensive.
Orthogonal arrays help identify the most influential factors and their interactions, thereby guiding you towards improvements with fewer trials.
Benefits of Using Orthogonal Arrays
Orthogonal arrays offer several advantages in experimental design.
Firstly, they save time and resources by reducing the number of tests needed to understand the variables fully.
Secondly, they allow for a better understanding of interactions between different factors, which might be missed if variables were tested individually.
Additionally, orthogonal arrays promote efficiency by enabling the testing of complex systems in a simplified manner.
Steps to Implement Orthogonal Arrays in Experiments
Define Your Objective
The first step in using orthogonal arrays is defining the objective of your experiment.
What exactly do you want to learn or achieve through your experiment?
Whether it’s improving a product design or optimizing a manufacturing process, having a clear objective will guide your entire experiment.
Select Factors and Levels
Identify the factors that might influence the outcome.
These could be design parameters, environmental conditions, or material properties.
Next, determine the levels of each factor, which are the different values or settings you want to test.
For example, if you’re testing a new material, the factors might include temperature and pressure, with levels such as high, medium, and low.
Choose the Orthogonal Array
Choose the appropriate orthogonal array based on the number of factors and levels.
There are different types of arrays, like the L4, L8, or L18 arrays, often named based on the number of experiments conducted.
Software tools can help in selecting the right array, ensuring that your design is both effective and efficient.
Conduct the Experiment
With your orthogonal array selected, conduct the experiments as outlined.
Ensure that each test run is performed accurately and consistently to obtain reliable data.
It’s crucial to document each step and the corresponding results for thorough analysis later.
Analyze the Results
Once the experiments are complete, analyze the data using statistical software.
The goal is to determine which factors have the most significant impact on your target outcome.
Look for patterns and interactions in the data that provide insights into optimizing your process.
Examples of DOE in Action
Let’s consider a real-world case where orthogonal arrays have made a significant difference.
In an automotive design department, engineers used orthogonal arrays to improve fuel efficiency.
By testing various engine components and configurations, they identified the optimal combination, leading to a notable increase in efficiency with minimal performance tests.
This approach saved the company time, reduced costs, and improved product performance, showcasing the power of DOE.
Common Pitfalls and How to Avoid Them
While using orthogonal arrays, it is important to avoid certain common pitfalls.
Overlooking Factor Interactions
One major mistake is ignoring potential interactions between factors.
Interactions can significantly influence outcomes and might be more critical than individual factor effects.
Ensure that your analysis considers these interactions to draw accurate conclusions.
Inadequate Data Collection
Accurate data collection is vital in any experiment.
Inadequate or poor-quality data can lead to erroneous conclusions.
Always double-check your measurements and maintain consistency across all trial runs.
Improper Selection of Arrays
Selecting the wrong orthogonal array can compromise your results.
Make sure that the chosen array fits the number of factors and levels accurately.
Consult statistical references or software to ensure correct array selection.
Conclusion
The effective use of design of experiments with orthogonal arrays is crucial for optimizing processes and improving product designs.
For mid-level employees in design departments, mastering this technique can lead to significant enhancements in efficiency and performance.
By carefully defining objectives, selecting the right factors and arrays, and systematically analyzing the results, you can make informed decisions that drive success.
Understanding and applying DOE not only enhances your skill set but also contributes to the overall innovation and competitiveness of your organization.
資料ダウンロード
QCD調達購買管理クラウド「newji」は、調達購買部門で必要なQCD管理全てを備えた、現場特化型兼クラウド型の今世紀最高の購買管理システムとなります。
ユーザー登録
調達購買業務の効率化だけでなく、システムを導入することで、コスト削減や製品・資材のステータス可視化のほか、属人化していた購買情報の共有化による内部不正防止や統制にも役立ちます。
NEWJI DX
製造業に特化したデジタルトランスフォーメーション(DX)の実現を目指す請負開発型のコンサルティングサービスです。AI、iPaaS、および先端の技術を駆使して、製造プロセスの効率化、業務効率化、チームワーク強化、コスト削減、品質向上を実現します。このサービスは、製造業の課題を深く理解し、それに対する最適なデジタルソリューションを提供することで、企業が持続的な成長とイノベーションを達成できるようサポートします。
オンライン講座
製造業、主に購買・調達部門にお勤めの方々に向けた情報を配信しております。
新任の方やベテランの方、管理職を対象とした幅広いコンテンツをご用意しております。
お問い合わせ
コストダウンが利益に直結する術だと理解していても、なかなか前に進めることができない状況。そんな時は、newjiのコストダウン自動化機能で大きく利益貢献しよう!
(Β版非公開)