- お役立ち記事
- Practical method of demand forecasting and order optimization in purchasing department using AI
Practical method of demand forecasting and order optimization in purchasing department using AI
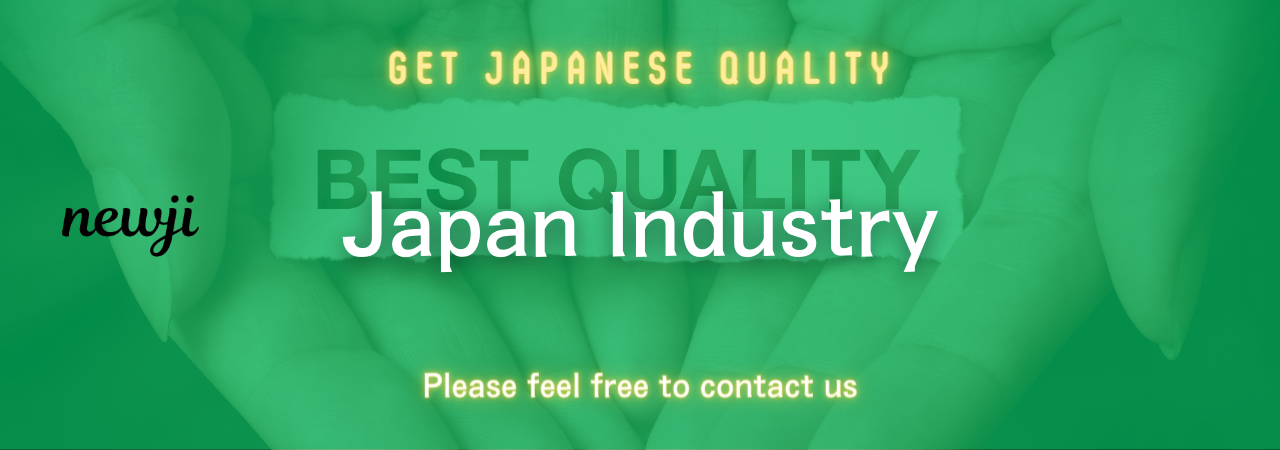
目次
Understanding Demand Forecasting
Demand forecasting is a critical process in the purchasing department of any organization.
It involves predicting future customer demand to optimize purchasing, inventory management, and production schedules.
Accurate demand forecasting ensures that companies can meet customer needs without overstocking or understocking products.
The advent of artificial intelligence (AI) has revolutionized this process, offering more precision and efficiency.
AI-driven methods leverage large datasets and advanced algorithms to provide accurate predictions, ultimately helping businesses make informed decisions.
The Role of AI in Demand Forecasting
Artificial intelligence plays a pivotal role in enhancing demand forecasting through its ability to process vast amounts of data quickly and accurately.
AI algorithms analyze historical sales data, market trends, consumer behavior, seasonal patterns, and even external factors like economic indicators and weather forecasts.
By identifying patterns in this data, AI can make accurate predictions about future demand.
This predictive power allows businesses to anticipate changes in demand and adjust their purchasing and inventory control strategies accordingly.
AI’s ability to learn and adapt over time further improves the accuracy of demand forecasts, as it can continually refine its models based on new data.
Steps to Implement AI in Demand Forecasting
Implementing AI in demand forecasting requires a systematic approach to ensure it aligns with business objectives and processes.
Here are the essential steps:
1. **Data Collection**: Gather comprehensive historical sales data along with relevant external data sources.
This may include market trends, social media insights, customer feedback, and competitor analysis.
2. **Data Cleaning and Preparation**: Ensure data quality by cleaning and pre-processing.
This involves handling missing values, eliminating duplicates, and normalizing data formats.
3. **Model Selection**: Choose appropriate AI algorithms based on business requirements.
Commonly used models include machine learning algorithms such as regression models, time-series analysis, and neural networks.
4. **Training and Testing**: Train the selected model using historical data and validate its accuracy through testing.
This step involves fine-tuning parameters and optimizing model performance.
5. **Integration and Implementation**: Integrate the AI model into existing systems and processes.
Ensure seamless communication between the forecasting model and other business functions like procurement, production, and sales.
6. **Continuous Monitoring and Improvement**: Continuously monitor the performance of AI models and update them with new data.
Regularly review forecasting accuracy and refine models as necessary.
Order Optimization Strategies
Order optimization involves determining the right quantity and timing of orders to match forecasted demand while minimizing costs.
AI-driven demand forecasting provides the insights needed to achieve efficient order optimization.
By accurately predicting demand fluctuations, businesses can optimize their order quantities, reduce carrying costs, and avoid stockouts or excess inventory.
Key Techniques for Order Optimization
There are several effective techniques for order optimization using AI-driven insights:
1. **Just-In-Time (JIT) Inventory**: JIT is an inventory management approach that aims to minimize inventory levels by aligning orders with demand forecasts.
With AI-driven forecasting, businesses can refine their JIT strategies, ensuring that inventory levels are sufficient to meet demand without overstocking.
2. **Safety Stock Calculation**: AI models can help determine optimal safety stock levels by considering demand variability and lead time variability.
This ensures that inventory is available to cover unforeseen demand spikes or supply chain disruptions.
3. **Dynamic Reordering**: Utilize AI to create a dynamic reordering system that adjusts order quantities and timing based on real-time demand forecasts.
This flexibility allows businesses to respond quickly to changes in demand while minimizing carrying costs.
4. **Supplier Collaboration**: AI-powered demand forecasting facilitates better collaboration with suppliers.
By sharing accurate demand forecasts, suppliers can adjust their production schedules and inventory levels, reducing lead times and improving supply chain efficiency.
Benefits of AI-Driven Demand Forecasting and Order Optimization
Implementing AI for demand forecasting and order optimization offers numerous benefits for purchasing departments:
– **Improved Accuracy**: AI models provide higher forecasting accuracy by analyzing a broad range of data inputs and identifying patterns that may not be apparent with traditional methods.
– **Cost Reduction**: By optimizing orders and reducing inventory carrying costs, businesses can achieve significant cost savings.
– **Enhanced Customer Satisfaction**: Ensuring product availability while avoiding excess inventory leads to improved customer satisfaction and loyalty.
– **Increased Agility**: AI allows companies to respond swiftly to changes in market conditions, ensuring they remain competitive and agile in dynamic environments.
– **Better Resource Allocation**: With accurate demand forecasts, businesses can allocate resources more efficiently, focusing on areas that deliver the most value.
Challenges and Considerations
While the benefits of AI-driven demand forecasting and order optimization are substantial, there are also challenges to consider:
– **Data Quality**: The accuracy of AI forecasts relies heavily on data quality.
Ensuring high-quality, comprehensive datasets is crucial for reliable predictions.
– **Integration Complexity**: Integrating AI systems with existing business processes can be complex, requiring careful planning and change management.
– **Initial Investment**: Implementing AI solutions may require an upfront investment in technology and training.
However, the long-term benefits often outweigh initial costs.
– **Continuous Improvement**: AI models require ongoing monitoring and adaptation to remain effective.
Businesses must be committed to continual improvement to maximize AI’s potential.
In summary, AI-driven demand forecasting and order optimization are transformative practices for purchasing departments.
By leveraging AI technologies, organizations can enhance their forecasting accuracy, optimize orders, and achieve greater operational efficiency.
These capabilities ultimately lead to cost savings, improved customer satisfaction, and increased competitiveness in today’s dynamic market landscape.
資料ダウンロード
QCD調達購買管理クラウド「newji」は、調達購買部門で必要なQCD管理全てを備えた、現場特化型兼クラウド型の今世紀最高の購買管理システムとなります。
ユーザー登録
調達購買業務の効率化だけでなく、システムを導入することで、コスト削減や製品・資材のステータス可視化のほか、属人化していた購買情報の共有化による内部不正防止や統制にも役立ちます。
NEWJI DX
製造業に特化したデジタルトランスフォーメーション(DX)の実現を目指す請負開発型のコンサルティングサービスです。AI、iPaaS、および先端の技術を駆使して、製造プロセスの効率化、業務効率化、チームワーク強化、コスト削減、品質向上を実現します。このサービスは、製造業の課題を深く理解し、それに対する最適なデジタルソリューションを提供することで、企業が持続的な成長とイノベーションを達成できるようサポートします。
オンライン講座
製造業、主に購買・調達部門にお勤めの方々に向けた情報を配信しております。
新任の方やベテランの方、管理職を対象とした幅広いコンテンツをご用意しております。
お問い合わせ
コストダウンが利益に直結する術だと理解していても、なかなか前に進めることができない状況。そんな時は、newjiのコストダウン自動化機能で大きく利益貢献しよう!
(Β版非公開)