- お役立ち記事
- Basics of price prediction algorithms useful for purchasing strategies in the manufacturing industry
Basics of price prediction algorithms useful for purchasing strategies in the manufacturing industry
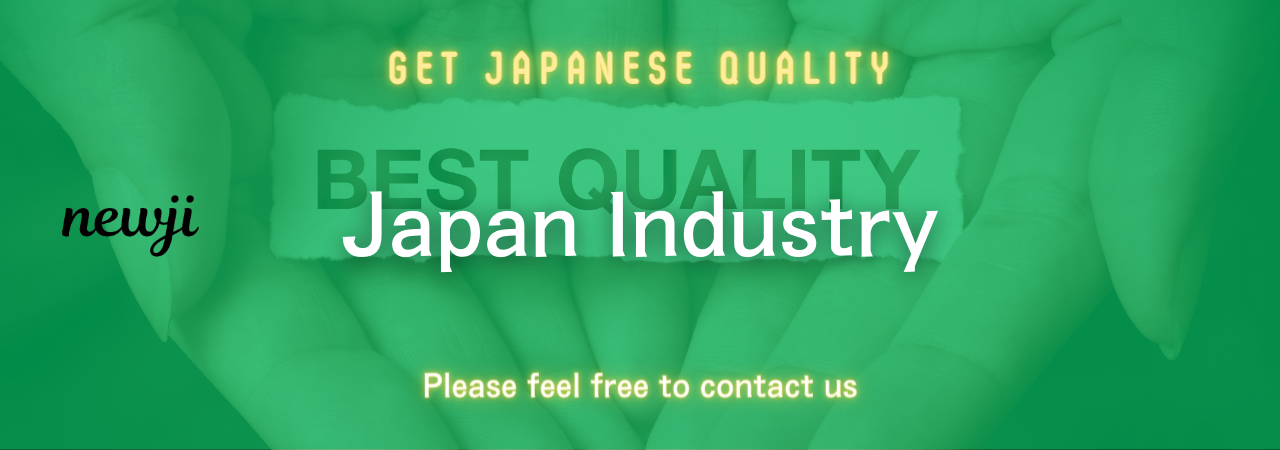
目次
Introduction to Price Prediction Algorithms
Price prediction algorithms have become essential tools in various industries, especially in manufacturing, where purchasing strategies significantly impact profitability.
These algorithms leverage complex mathematical models and data analysis to forecast future prices based on historical and real-time data.
By effectively utilizing price prediction algorithms, manufacturing companies can make informed purchasing decisions, reduce costs, and gain a competitive edge in the market.
How Price Prediction Algorithms Work
Price prediction algorithms use a combination of statistical techniques, machine learning, and artificial intelligence to forecast future prices.
The process begins with the collection of relevant data, which can include past prices, supply and demand information, production costs, and external factors like economic indicators and geopolitical events.
Data Collection and Preprocessing
In the initial stage, data collection is paramount.
Manufacturers need to gather comprehensive datasets from various sources, including their own records, online databases, and market research firms.
Once the data is collected, it must undergo preprocessing to remove any noise or errors.
This step is crucial for ensuring the quality of data fed into the algorithm, as inaccuracies can lead to incorrect predictions.
Model Selection and Training
Choosing the right model is vital for accurate price predictions.
Common models include linear regression, neural networks, decision trees, and support vector machines.
Each model has its strengths and weaknesses, and the selection depends on the nature of the data and the specific requirements of the manufacturer.
The chosen model is then trained using historical data.
During training, the algorithm learns the patterns and relationships in the data, fine-tuning its parameters to predict future prices accurately.
Forecasting and Analysis
Once trained, the algorithm can forecast future prices based on new data inputs.
These predictions are often accompanied by confidence intervals, providing a range of possible outcomes and their probabilities.
Manufacturers can then analyze these forecasts to develop purchasing strategies that optimize costs and minimize risks.
Benefits of Using Price Prediction Algorithms in Manufacturing
Cost Reduction
One of the primary advantages of using price prediction algorithms is the potential for significant cost savings.
By anticipating future price fluctuations, manufacturers can time their purchases to take advantage of lower prices or avoid periods of high costs.
This proactive approach to purchasing reduces the overall cost of raw materials and components, directly impacting the bottom line.
Improved Decision-Making
Price prediction algorithms enhance decision-making by providing data-driven insights.
Manufacturers can rely on these insights to make informed choices regarding inventory management, production planning, and budgeting.
By aligning purchasing strategies with predicted price trends, companies can optimize their resource allocation and operational efficiency.
Competitive Advantage
In the highly competitive manufacturing industry, having access to reliable price predictions is a significant advantage.
Companies that use these algorithms can respond more quickly to market changes, ensuring they remain competitive and resilient.
This agility enables manufacturers to adjust their strategies in real-time, seizing opportunities and mitigating risks.
Challenges and Limitations
Data Quality and Availability
The effectiveness of price prediction algorithms heavily depends on the quality and availability of data.
Incomplete or inaccurate data can lead to incorrect predictions, potentially causing financial losses for manufacturers.
Ensuring access to reliable data sources and maintaining rigorous data quality standards are ongoing challenges.
Complexity and Technical Expertise
Developing and implementing price prediction algorithms require specialized knowledge in data science and machine learning.
Manufacturers may face challenges in recruiting or training personnel with the necessary expertise to design and maintain these systems.
Adaptability to Market Changes
The manufacturing industry is subject to rapid and unpredictable changes driven by factors such as technological advancements, regulatory changes, and shifts in consumer demand.
Price prediction algorithms must be continuously updated and validated to remain effective in this dynamic environment.
Future Trends in Price Prediction Algorithms
The future of price prediction algorithms in the manufacturing industry holds promising advancements that will further improve accuracy and usability.
Integration with Artificial Intelligence
Artificial intelligence offers significant potential for enhancing price prediction algorithms.
Advanced AI models can process vast amounts of data more efficiently, uncovering patterns that traditional methods might miss.
As AI technology evolves, manufacturers can expect more sophisticated and precise price forecasts.
Real-Time Data Analysis
The shift towards real-time data analysis will revolutionize price prediction in manufacturing.
Instead of relying solely on historical data, future algorithms will continuously update predictions based on the latest market developments.
This capability will allow manufacturers to react almost instantaneously to market changes, optimizing their purchasing strategies on the fly.
Collaborative Platforms
As the industry moves towards increased collaboration and data sharing, price prediction algorithms can benefit from access to more comprehensive datasets.
These collaborative platforms can facilitate information exchange between manufacturers, suppliers, and industry partners, enriching the data pool for more accurate predictions.
Conclusion
Price prediction algorithms have become indispensable tools in the manufacturing industry, offering substantial benefits such as cost reduction, improved decision-making, and a competitive edge.
Despite challenges like data quality and technical complexity, ongoing advancements in AI, real-time data analysis, and collaboration promise a bright future for these algorithms.
By embracing these technologies, manufacturers can adapt to the evolving market landscape, maximizing efficiency and profitability in their purchasing strategies.
資料ダウンロード
QCD調達購買管理クラウド「newji」は、調達購買部門で必要なQCD管理全てを備えた、現場特化型兼クラウド型の今世紀最高の購買管理システムとなります。
ユーザー登録
調達購買業務の効率化だけでなく、システムを導入することで、コスト削減や製品・資材のステータス可視化のほか、属人化していた購買情報の共有化による内部不正防止や統制にも役立ちます。
NEWJI DX
製造業に特化したデジタルトランスフォーメーション(DX)の実現を目指す請負開発型のコンサルティングサービスです。AI、iPaaS、および先端の技術を駆使して、製造プロセスの効率化、業務効率化、チームワーク強化、コスト削減、品質向上を実現します。このサービスは、製造業の課題を深く理解し、それに対する最適なデジタルソリューションを提供することで、企業が持続的な成長とイノベーションを達成できるようサポートします。
オンライン講座
製造業、主に購買・調達部門にお勤めの方々に向けた情報を配信しております。
新任の方やベテランの方、管理職を対象とした幅広いコンテンツをご用意しております。
お問い合わせ
コストダウンが利益に直結する術だと理解していても、なかなか前に進めることができない状況。そんな時は、newjiのコストダウン自動化機能で大きく利益貢献しよう!
(Β版非公開)