- お役立ち記事
- Deep Learning for Optimizing Optical Measurement Data Analysis
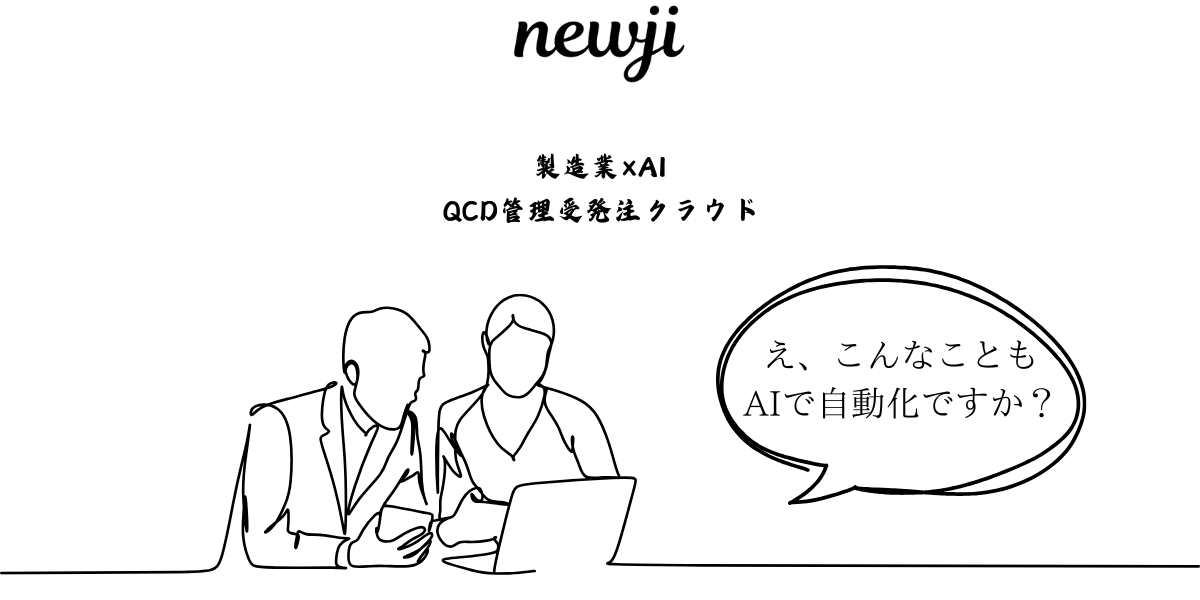
Deep Learning for Optimizing Optical Measurement Data Analysis
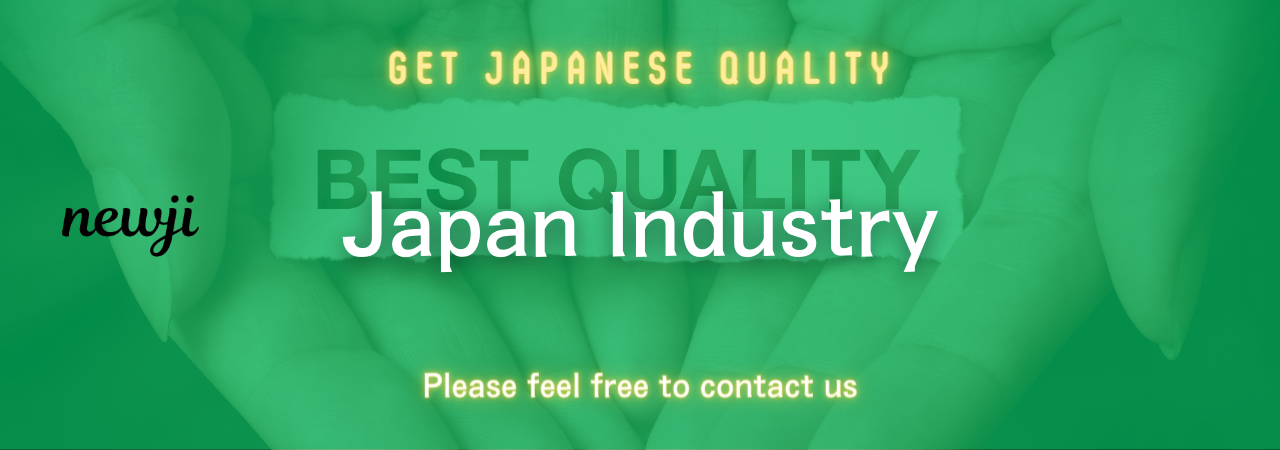
目次
Introduction to Deep Learning
Deep learning, a subset of machine learning, is revolutionizing various fields by enabling systems to learn from data patterns.
It mimics the human brain, using layers of artificial neural networks to process information.
Each layer identifies different features, gradually improving overall analysis and decision-making capabilities.
The Importance of Optical Measurement Data
Optical measurement is widely used in industries to provide precise data on physical quantities.
These measurements are crucial for quality control, product development, and compliance with standards.
Accurate data analysis is essential to derive meaningful insights and make informed decisions.
Challenges in Optical Data Analysis
However, analyzing optical measurement data comes with its challenges.
The sheer volume of data can be overwhelming, and traditional methods often struggle with processing speed and accuracy.
Additionally, measurement errors, noise, and variable conditions can complicate the interpretation of data.
Deep Learning to the Rescue
Deep learning offers a promising solution to these challenges by enhancing the accuracy and efficiency of data analysis.
Its ability to process large volumes of data at high speed makes it ideal for handling complex datasets.
Moreover, deep learning models are adaptive, continuously improving as they process more data, which allows them to cope with the variability inherent in optical measurements.
Preprocessing Optical Data
Before applying deep learning, it is crucial to preprocess the optical measurement data.
This step involves cleaning the data, removing noise, and normalizing it to enhance the performance of the neural networks.
Preprocessed data ensures that deep learning models have accurate input for analysis, leading to more reliable outcomes.
Feature Extraction with Deep Learning
One of the main advantages of deep learning in optical data analysis is its ability to automatically extract relevant features from the data.
This automatic feature extraction reduces the need for manual intervention and expertise, saving time and resources.
Deep learning models can identify intricate patterns that would be difficult, if not impossible, for human analysts to detect.
Applications of Deep Learning in Optical Measurement
Deep learning techniques are being successfully applied across different applications within optical measurement.
Quality Control in Manufacturing
In manufacturing, ensuring product quality is paramount.
Deep learning models can analyze optical data to detect defects and anomalies with high precision, ensuring stringent quality standards are met.
This capability reduces waste and enhances the overall efficiency of production lines.
Medical Imaging and Diagnostics
In the medical field, optical measurements are critical in diagnostics and imaging.
Deep learning algorithms assist in interpreting complex imaging data, facilitating early diagnosis and treatment planning.
These models improve accuracy, reduce interpretation time, and enable healthcare professionals to focus on patient care.
Environmental Monitoring
Deep learning aids in analyzing optical data from environmental sensors, helping monitor pollution levels, weather patterns, and other ecological factors.
The ability to process large datasets quickly allows for real-time monitoring and prompt intervention when necessary.
Advantages of Using Deep Learning
The use of deep learning in optical measurement data analysis offers numerous benefits.
Improved Accuracy and Precision
Deep learning models are designed to handle vast amounts of data and complex patterns, leading to more accurate and precise analyses than traditional methods.
Faster Processing Time
Deep learning algorithms can rapidly process data, significantly reducing the time required for analysis.
This speed is particularly beneficial in situations that require real-time decision-making.
Scalability
These models are scalable, meaning they can be trained on large datasets without a significant increase in computational requirements.
This scalability ensures that as more data becomes available, the system continues to improve its performance.
Conclusion
Deep learning is transforming the world of optical measurement data analysis by enhancing precision, speed, and scalability.
Its ability to automatically extract features and handle large datasets makes it an invaluable tool for various applications, from manufacturing to environmental monitoring.
As technology continues to advance, we can expect deep learning to play an increasingly crucial role in optimizing optical measurement data analysis.
資料ダウンロード
QCD調達購買管理クラウド「newji」は、調達購買部門で必要なQCD管理全てを備えた、現場特化型兼クラウド型の今世紀最高の購買管理システムとなります。
ユーザー登録
調達購買業務の効率化だけでなく、システムを導入することで、コスト削減や製品・資材のステータス可視化のほか、属人化していた購買情報の共有化による内部不正防止や統制にも役立ちます。
NEWJI DX
製造業に特化したデジタルトランスフォーメーション(DX)の実現を目指す請負開発型のコンサルティングサービスです。AI、iPaaS、および先端の技術を駆使して、製造プロセスの効率化、業務効率化、チームワーク強化、コスト削減、品質向上を実現します。このサービスは、製造業の課題を深く理解し、それに対する最適なデジタルソリューションを提供することで、企業が持続的な成長とイノベーションを達成できるようサポートします。
オンライン講座
製造業、主に購買・調達部門にお勤めの方々に向けた情報を配信しております。
新任の方やベテランの方、管理職を対象とした幅広いコンテンツをご用意しております。
お問い合わせ
コストダウンが利益に直結する術だと理解していても、なかなか前に進めることができない状況。そんな時は、newjiのコストダウン自動化機能で大きく利益貢献しよう!
(Β版非公開)