- お役立ち記事
- Practice of fluid prediction and optimal control using fluid dynamics and reinforcement learning
月間76,176名の
製造業ご担当者様が閲覧しています*
*2025年3月31日現在のGoogle Analyticsのデータより
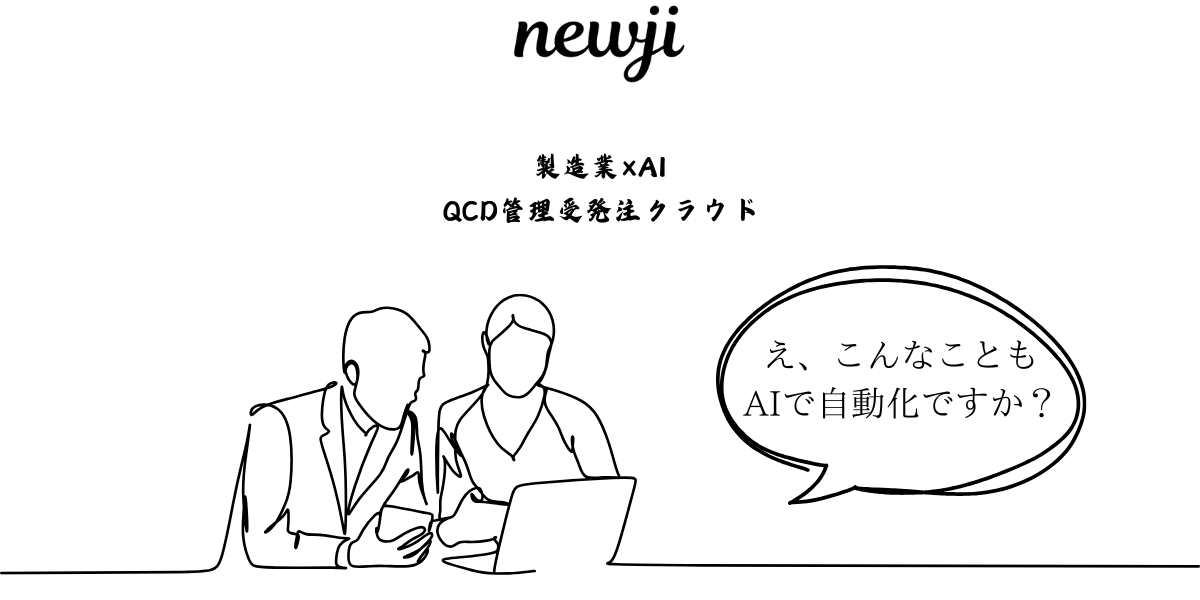
Practice of fluid prediction and optimal control using fluid dynamics and reinforcement learning
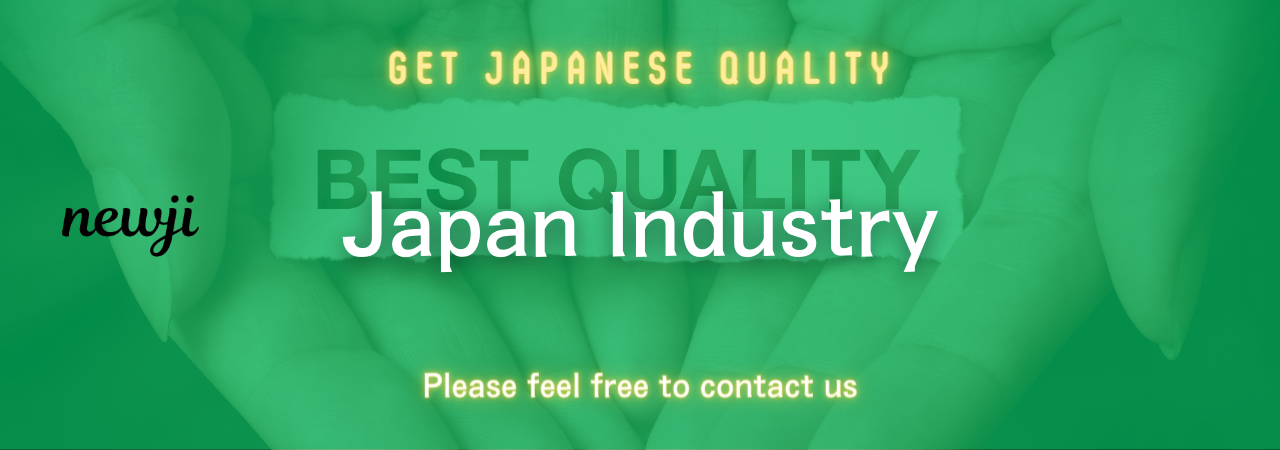
目次
Introduction to Fluid Dynamics
Fluid dynamics is a branch of physics concerned with the study of fluids (liquids and gases) in motion.
It plays a crucial role in various fields, from understanding natural phenomena like ocean currents and weather patterns to engineering applications such as designing aircraft, automobiles, and pipelines.
Fluid dynamics helps predict how fluids behave, allowing scientists and engineers to optimize systems for efficiency and effectiveness.
Predicting fluid behavior and controlling it optimally has traditionally been a complex task due to the chaotic and nonlinear nature of fluid flow.
Importance of Predicting Fluid Behavior
Predicting fluid behavior has significant implications in numerous industries.
In the aviation industry, for instance, the ability to forecast how air flows over wing surfaces can dramatically enhance aircraft design, reducing drag and improving fuel efficiency.
Similarly, in the automotive sector, understanding fluid flow under and around vehicles can lead to more aerodynamic car designs that consume less fuel.
In the chemical and process industries, ensuring fluids mix optimally can improve product quality and energy efficiency.
Accurate fluid dynamics predictions also bolster our ability to respond to natural disasters such as hurricanes by improving weather models.
Challenges in Fluid Dynamics Prediction
Predicting fluid dynamics is filled with challenges.
Fluids are governed by the Navier-Stokes equations, which are notoriously difficult to solve due to their nonlinear nature.
Even simplified models often lead to complex equations that require significant computational resources.
Moreover, real-world conditions include various external factors and unpredictable forces, making accurate predictions even harder.
Capturing turbulence, a common aspect of fluid dynamics, adds another layer of difficulty.
Turbulence is characterized by chaotic changes in pressure and flow velocity, which complicates predictions.
Despite advances in computational power and numerical methods, achieving highly accurate predictions remains a hurdle.
Reinforcement Learning in Fluid Dynamics
Recent advances in artificial intelligence, particularly reinforcement learning (RL), have opened new avenues in fluid dynamics research.
Reinforcement learning is a type of machine learning where an agent learns to make decisions by interacting with an environment in order to achieve a goal.
Unlike traditional machine learning, RL doesn’t require a fixed dataset; instead, it learns from trial and error, making it suitable for dynamic and complex systems like fluid flows.
Incorporating RL into fluid dynamics allows for adaptive learning and optimization.
For instance, RL algorithms can be used to find the optimal shape of an object to minimize drag in various flow conditions.
This approach allows for continuous learning and adaptation, leading to improvements over existing static models.
Optimal Control Using Reinforcement Learning
Optimal control in fluid dynamics involves tweaking parameters and conditions in a fluid system to achieve desired outcomes, such as minimizing energy consumption or maximizing throughput.
With reinforcement learning, it’s possible to automate and refine these control strategies more efficiently than traditional methods.
Advantages of RL in Optimal Control
One of the primary advantages of using RL for optimal control is its ability to handle high-dimensional and complex control spaces.
Traditional optimization algorithms might struggle with the vast array of variables and outcomes in fluid systems.
In contrast, RL can explore these spaces more effectively, learning from the outcomes of different actions.
Moreover, RL can better handle nonlinearity, uncertainty, and noise, which are inherent in fluid dynamics, providing more robust solutions.
Applications of RL in Fluid Control
RL has been successfully applied to various fluid control scenarios.
In HVAC systems, for example, RL algorithms have been used to dynamically adjust the flow and temperature of air to optimize energy usage while maintaining comfort.
In biotechnology, RL has been applied to optimize the mixing of fluids in reactors, improving yields and efficiency.
In the context of aerospace, RL can aid in the development of adaptable control surfaces for aircraft, leading to more efficient flight operations.
The versatility and adaptive nature of RL make it a powerful tool in optimizing fluid dynamic systems.
The Future of Fluid Dynamics with AI
The integration of AI and machine learning with fluid dynamics is poised to revolutionize both research and practical applications.
RL’s ability to learn from interactions and adapt to evolving conditions offers potential breakthroughs in understanding and optimizing fluid behavior.
As computational power and algorithm efficiency continue to improve, RL could become a cornerstone technology in fluid dynamics research.
Challenges and Considerations
Despite its potential, there are challenges in implementing RL in fluid dynamics.
Training RL models can be computationally intensive and require significant time and resources.
Ensuring that RL models generalize well beyond their training conditions is also crucial to prevent overfitting.
Collaboration between experts in fluid dynamics and computer science is essential to overcome these hurdles and enhance the effectiveness of RL in this domain.
Conclusion
The practice of predicting and optimizing fluid dynamics using reinforcement learning marks a significant step forward in leveraging machine learning for complex physical systems.
By embracing the capabilities of RL, we unlock new possibilities for innovation in industries reliant on fluid dynamics.
The potential benefits include heightened efficiencies, reduced costs, and innovative strategies for managing fluid flows.
The marriage of AI with fluid dynamics heralds a promising future, where adaptable and intelligent systems are at the forefront of advancements.
資料ダウンロード
QCD管理受発注クラウド「newji」は、受発注部門で必要なQCD管理全てを備えた、現場特化型兼クラウド型の今世紀最高の受発注管理システムとなります。
ユーザー登録
受発注業務の効率化だけでなく、システムを導入することで、コスト削減や製品・資材のステータス可視化のほか、属人化していた受発注情報の共有化による内部不正防止や統制にも役立ちます。
NEWJI DX
製造業に特化したデジタルトランスフォーメーション(DX)の実現を目指す請負開発型のコンサルティングサービスです。AI、iPaaS、および先端の技術を駆使して、製造プロセスの効率化、業務効率化、チームワーク強化、コスト削減、品質向上を実現します。このサービスは、製造業の課題を深く理解し、それに対する最適なデジタルソリューションを提供することで、企業が持続的な成長とイノベーションを達成できるようサポートします。
製造業ニュース解説
製造業、主に購買・調達部門にお勤めの方々に向けた情報を配信しております。
新任の方やベテランの方、管理職を対象とした幅広いコンテンツをご用意しております。
お問い合わせ
コストダウンが利益に直結する術だと理解していても、なかなか前に進めることができない状況。そんな時は、newjiのコストダウン自動化機能で大きく利益貢献しよう!
(β版非公開)