- お役立ち記事
- Multi-Agent System Control Basics and Python-Based Implementation
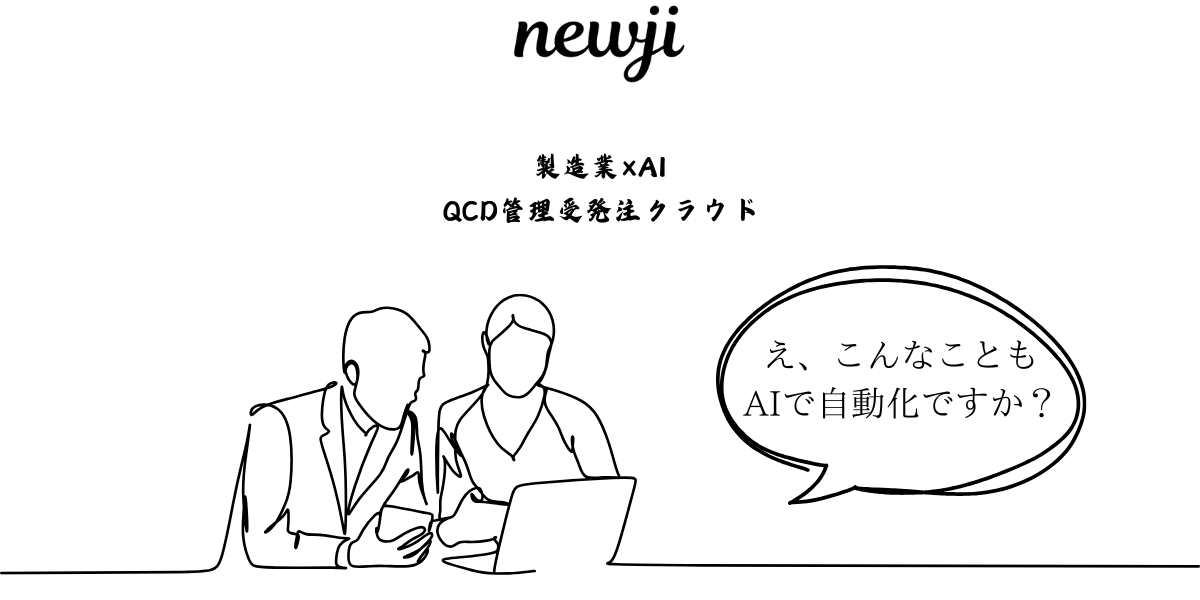
Multi-Agent System Control Basics and Python-Based Implementation
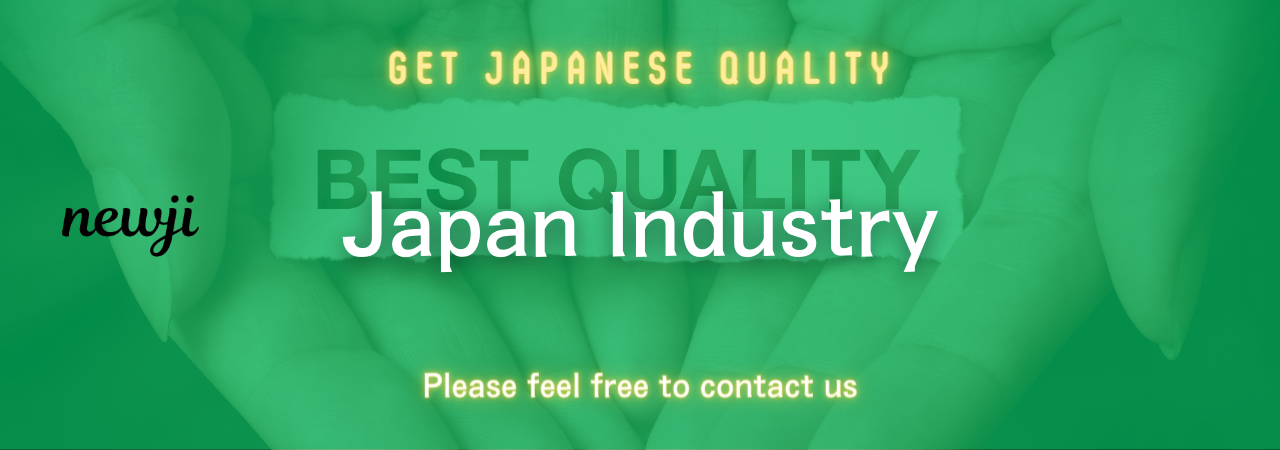
目次
Introduction to Multi-Agent Systems
Multi-agent systems (MAS) are a fascinating and essential area of study in the field of computer science and artificial intelligence.
They consist of multiple interacting agents, each capable of making individual decisions to achieve specific goals.
MAS can be applied in various domains such as robotics, economics, and traffic management.
Understanding how these systems work begins with recognizing the role of agents.
An agent is an autonomous entity that perceives its environment, makes decisions, and acts upon them.
These agents interact with each other to solve complex problems collaboratively or competitively.
The Basics of Multi-Agent System Control
MAS control involves managing the actions and interactions of agents to achieve desired outcomes.
Control strategies can be centralized or decentralized.
Centralized control systems rely on a central unit that supervises and manages all agents.
This approach simplifies coordination but can become a bottleneck and a single point of failure.
On the other hand, decentralized control distributes decision-making across individual agents.
This method enhances the system’s robustness and scalability but may require complex coordination protocols to ensure agents work efficiently together.
Coordination and Communication
Coordination is a cornerstone of efficient MAS.
It ensures agents work together without conflicts and inefficiencies.
Techniques such as negotiation, auctions, and consensus are employed to achieve coordination.
Communication between agents is equally vital.
Agents exchange information about their goals, constraints, and environment.
This exchange helps agents make informed decisions and adapt to changes swiftly.
Applications of Multi-Agent Systems
MAS are increasingly utilized across various sectors due to their versatile nature.
Robotics
Robotic systems often employ MAS to manage fleets of robots working in tandem.
Each robot acts autonomously, making decisions based on local information and collaborating to complete tasks like assembly or exploration.
Traffic and Transportation
In traffic management, MAS can optimize the flow of vehicles by adjusting traffic light patterns or suggesting alternate routes.
Agents represent individual vehicles, collecting and sharing information to improve overall traffic efficiency.
Economics and Finance
MAS play a significant role in modeling market dynamics.
Agents can represent buyers, sellers, or financial entities in simulations that help analyze market behavior and test economic theories.
Implementing Multi-Agent Systems with Python
Python is an excellent language for developing MAS due to its simplicity and rich ecosystem of libraries.
Choosing the Right Framework
Several frameworks aid in building MAS, including:
– **JADE:** Although primarily for Java, you can integrate it with Python.
– **SPADE:** A multi-agent system platform developed in Python.
– **PADE:** Another Python-based framework suitable for developing and deploying MAS.
Selecting the right framework depends on your project requirements and familiarity with the language.
Setting Up a Basic Agent
Creating an agent in Python involves defining its properties and behavior.
Here is a simple example using a basic Python class:
“`python
class Agent:
def __init__(self, name):
self.name = name
def perceive(self, environment):
# Code to perceive the environment
pass
def decide(self):
# Code to make decisions
pass
def act(self):
# Code to act upon decisions
pass
“`
This template forms the basis of an agent’s structure.
You can expand it by adding methods for communication, coordination, and learning.
Testing and Debugging
Once your agents are created, you must test them in simulated environments.
Python offers multiple libraries for this purpose.
Using tools like **unittest** for testing and **logging** for debugging can streamline the development process.
Challenges and Future Directions
While MAS offer numerous advantages, they also present unique challenges.
Scalability
Scaling MAS to handle many agents without degrading performance can be challenging.
Efficient communication protocols and robust coordination strategies become paramount as the system grows.
Security and Privacy
In systems where agents exchange sensitive information, ensuring security and privacy is critical.
Techniques like encryption and anonymization are integral to maintaining trust in MAS.
Adapting to Dynamic Environments
Dynamic environments require agents to be highly adaptable.
Incorporating machine learning techniques enables agents to learn from experience and adapt to changing conditions effectively.
Conclusion
Multi-agent systems are a powerful concept in artificial intelligence with a wide range of applications.
Understanding MAS control basics and applying them using Python can lead to innovative solutions across various domains.
As technology advances, MAS will continue to evolve, presenting exciting opportunities and challenges for researchers and practitioners alike.
資料ダウンロード
QCD調達購買管理クラウド「newji」は、調達購買部門で必要なQCD管理全てを備えた、現場特化型兼クラウド型の今世紀最高の購買管理システムとなります。
ユーザー登録
調達購買業務の効率化だけでなく、システムを導入することで、コスト削減や製品・資材のステータス可視化のほか、属人化していた購買情報の共有化による内部不正防止や統制にも役立ちます。
NEWJI DX
製造業に特化したデジタルトランスフォーメーション(DX)の実現を目指す請負開発型のコンサルティングサービスです。AI、iPaaS、および先端の技術を駆使して、製造プロセスの効率化、業務効率化、チームワーク強化、コスト削減、品質向上を実現します。このサービスは、製造業の課題を深く理解し、それに対する最適なデジタルソリューションを提供することで、企業が持続的な成長とイノベーションを達成できるようサポートします。
オンライン講座
製造業、主に購買・調達部門にお勤めの方々に向けた情報を配信しております。
新任の方やベテランの方、管理職を対象とした幅広いコンテンツをご用意しております。
お問い合わせ
コストダウンが利益に直結する術だと理解していても、なかなか前に進めることができない状況。そんな時は、newjiのコストダウン自動化機能で大きく利益貢献しよう!
(Β版非公開)