- お役立ち記事
- Basics of Time Series Data Analysis and Predictive Modeling Techniques
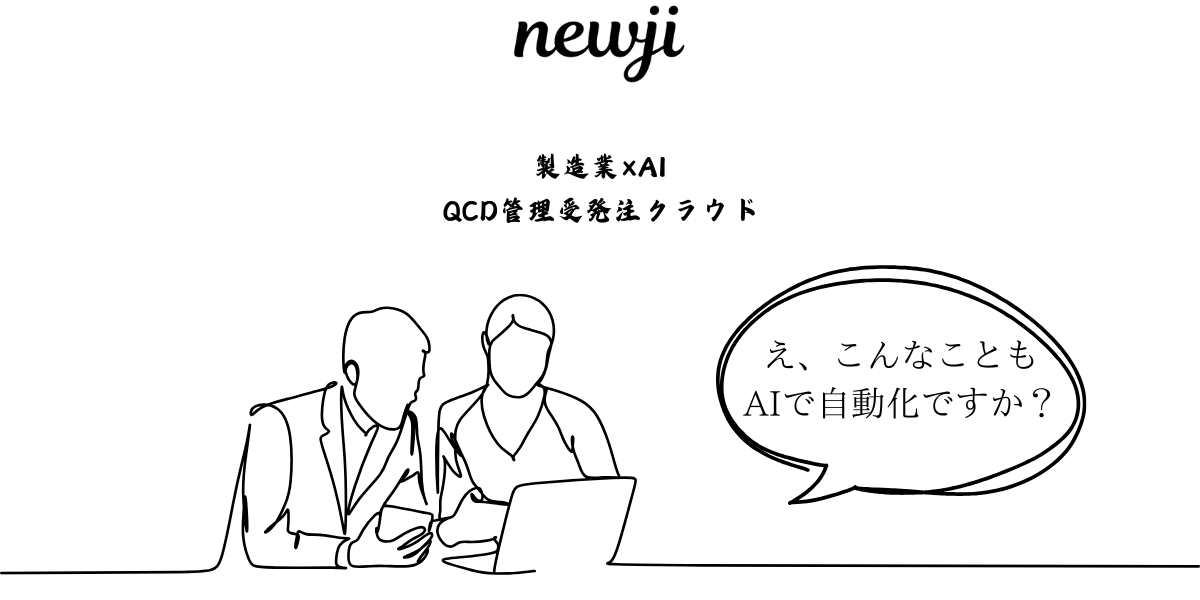
Basics of Time Series Data Analysis and Predictive Modeling Techniques
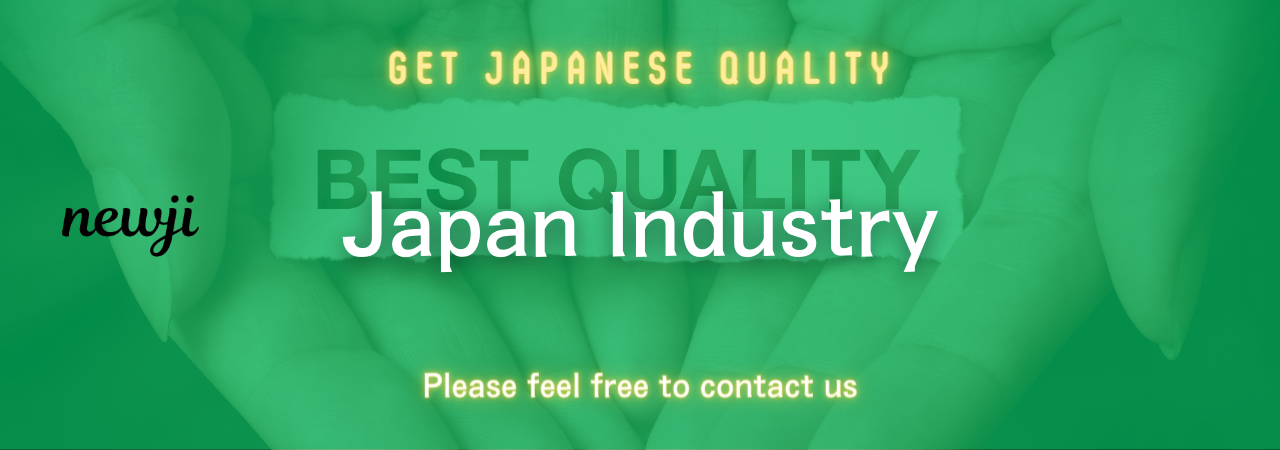
目次
Understanding Time Series Data
Time series data refers to a sequence of data points collected or recorded at specific time intervals.
This type of data is unique because it captures changes over time, allowing us to observe patterns, trends, and seasonal variations.
Common examples include daily stock prices, monthly sales figures, or annual weather records.
Components of Time Series Data
There are several key components in time series data that analysts need to consider:
1. **Trend**: This is the long-term movement or direction in the data.
It can be upward, downward, or stationary.
2. **Seasonality**: These are regular, predictable fluctuations that occur over specific periods such as days, months, or years.
For instance, retail sales might increase during the holiday season.
3. **Cyclical Patterns**: Unlike seasonality, cycles are not of a fixed period and can be influenced by economic conditions.
Cycles can last months or even years.
4. **Irregular Variations**: These are sudden changes in the time series that do not follow a pattern.
Examples include data spikes due to unforeseen events like natural disasters.
Why Time Series Analysis is Important
Time series analysis is crucial for various reasons:
– **Forecasting**: Accurate predictions can be made about future points using historical data.
This is particularly valuable in finance, weather forecasting, and inventory management.
– **Understanding Past Behavior**: By analyzing patterns, businesses can understand what has happened in the past and the reasons behind it.
– **Improving Decision Making**: Insights from time series data can lead to better strategic planning and operational efficiency.
Key Techniques for Time Series Analysis
Several methods are used in time series analysis, each with its own strengths.
1. Moving Averages
This technique involves averaging data over a specific period to smooth out short-term fluctuations and highlight longer-term trends.
It’s simple to implement and widely used in finance to see stock price trends.
2. Exponential Smoothing
This technique assigns exponentially decreasing weights to past observations, giving more importance to recent data.
It is particularly useful for short-term forecasting where the past is a good indicator of the future.
3. Autoregressive Integrated Moving Average (ARIMA)
ARIMA is a comprehensive modeling technique that combines AutoRegressive (AR) models, Integrated models (I), and Moving Averages (MA).
This method is useful for non-seasonal forecasting when trend and cyclic variations need to be captured.
4. Seasonal Decomposition of Time Series (STL)
STL is a method used to separate a time series into seasonal, trend, and residual components.
This decomposition helps analysts understand the underlying patterns in data, particularly those with clear seasonal effects.
5. Prophet Forecasting
Developed by Facebook, Prophet is particularly useful for data with strong seasonal effects and missing data points.
It is robust and suitable for automatically detecting trends and outliers.
Predictive Modeling with Time Series Data
Predictive modeling plays a critical role in time series analysis.
It allows businesses to anticipate future trends and make informed decisions.
Importance of Data Preparation
Before building predictive models, proper data preparation is crucial.
This includes:
– **Data Cleaning**: Removing outliers and handling missing data ensures the accuracy of your model.
– **Feature Engineering**: Creating relevant features such as lag variables or rolling statistical measures can improve model performance.
– **Data Splitting**: Dividing data into training and validation sets helps to assess the model’s effectiveness.
Choosing the Right Model
The choice of the model depends on various factors including data characteristics, desired accuracy, and complexity.
Common models include:
– **Linear Regression Models**: Useful for understanding the linear relationship between time series variables.
– **Machine Learning Models**: Methods like Random Forest or XGBoost can handle non-linear relationships and interactions effectively.
– **Deep Learning Models**: For complex datasets, frameworks like LSTM (Long Short-Term Memory) networks capture temporal patterns in sequential data.
Evaluating Model Performance
After building your predictive model, evaluating its performance is essential to ensure reliability.
Key Evaluation Metrics
– **Mean Absolute Error (MAE)**: Measures the average magnitude of errors in a set of predictions, without considering direction.
– **Root Mean Square Error (RMSE)**: Provides a measure of how much the predictions deviate from the actual values.
– **Mean Absolute Percentage Error (MAPE)**: Expresses prediction accuracy as a percentage, offering a better understanding of errors relative to actual values.
Continuous Improvement
Predictive modeling is not a one-time effort.
As new data becomes available, models should be regularly updated and refined to maintain their accuracy and relevance.
In conclusion, understanding the basics of time series data analysis and predictive modeling is crucial for anyone looking to leverage data for forecasting future trends.
By mastering these techniques and continuously refining your approach, you can make more informed decisions and gain a competitive edge in various domains.
資料ダウンロード
QCD調達購買管理クラウド「newji」は、調達購買部門で必要なQCD管理全てを備えた、現場特化型兼クラウド型の今世紀最高の購買管理システムとなります。
ユーザー登録
調達購買業務の効率化だけでなく、システムを導入することで、コスト削減や製品・資材のステータス可視化のほか、属人化していた購買情報の共有化による内部不正防止や統制にも役立ちます。
NEWJI DX
製造業に特化したデジタルトランスフォーメーション(DX)の実現を目指す請負開発型のコンサルティングサービスです。AI、iPaaS、および先端の技術を駆使して、製造プロセスの効率化、業務効率化、チームワーク強化、コスト削減、品質向上を実現します。このサービスは、製造業の課題を深く理解し、それに対する最適なデジタルソリューションを提供することで、企業が持続的な成長とイノベーションを達成できるようサポートします。
オンライン講座
製造業、主に購買・調達部門にお勤めの方々に向けた情報を配信しております。
新任の方やベテランの方、管理職を対象とした幅広いコンテンツをご用意しております。
お問い合わせ
コストダウンが利益に直結する術だと理解していても、なかなか前に進めることができない状況。そんな時は、newjiのコストダウン自動化機能で大きく利益貢献しよう!
(Β版非公開)