- お役立ち記事
- Data Analysis and Predictive Modeling for Researchers
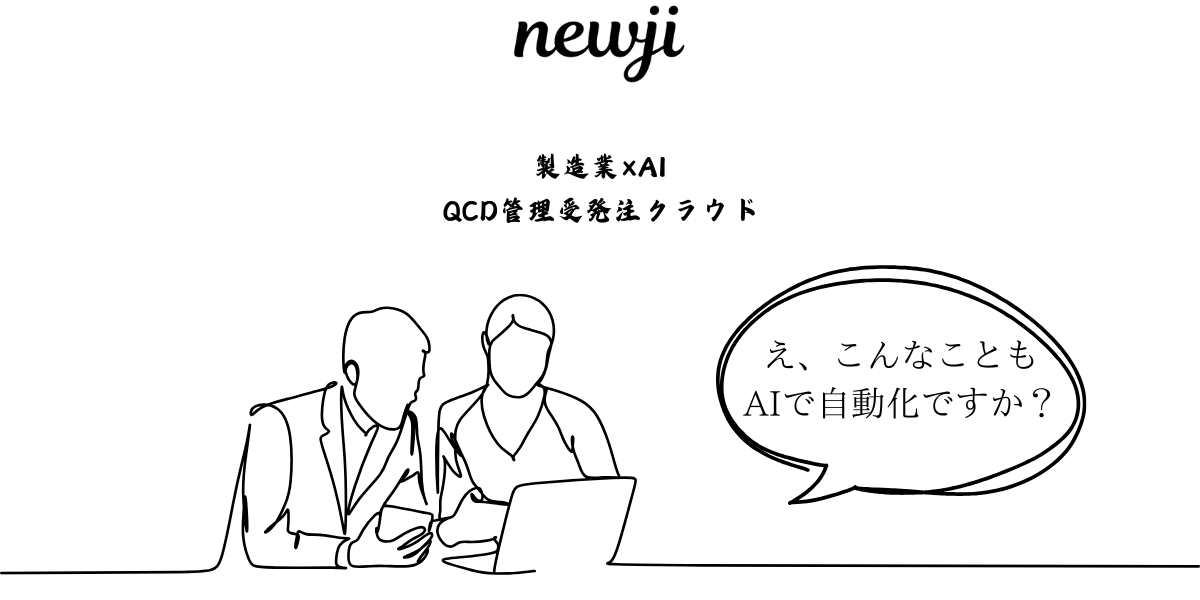
Data Analysis and Predictive Modeling for Researchers
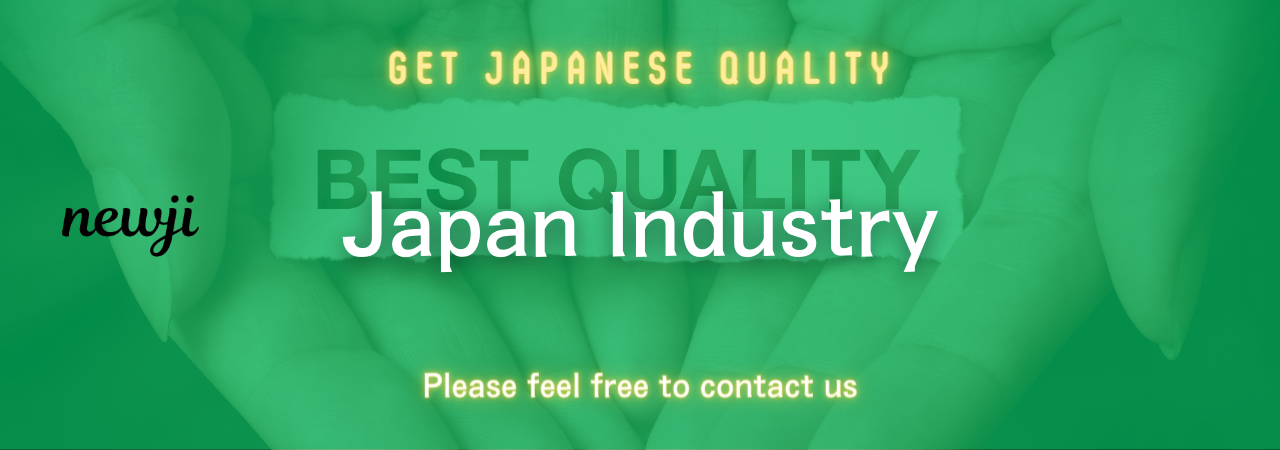
目次
Understanding Data Analysis
Data analysis is the process of inspecting, cleansing, transforming, and modeling data to discover useful information, draw conclusions, and support decision-making.
For researchers, data analysis is a fundamental aspect of their work as it helps them make sense of the various types of data they collect.
In essence, data analysis answers specific questions or tests hypotheses using statistical tools and techniques.
The initial stage of data analysis involves collecting relevant data from multiple sources.
This data can be quantitative, qualitative, or a combination of both.
Once the data is gathered, it needs to be cleaned and prepared for analysis.
Cleaning involves removing or correcting any inaccurate records from the dataset.
This ensures that the analysis is based on accurate and meaningful data.
Once the data is clean, it’s time to explore and understand it by summarizing its main characteristics.
Types of Data Analysis
There are several types of data analysis, each with specific purposes and outcomes.
Below are some common types:
1. **Descriptive Analysis:** This type of analysis helps describe the main features of a dataset.
Through descriptive statistics, such as mean, median, mode, and standard deviation, researchers can quickly summarize and understand the data.
Descriptive analysis provides a solid foundation for further analysis.
2. **Inferential Analysis:** Researchers use inferential analysis to make generalizations from a sample to a population.
By applying probability theory, inferential analysis allows researchers to estimate population parameters and test hypotheses.
Common techniques include regression analysis, t-tests, and chi-square tests.
3. **Exploratory Analysis:** Exploratory analysis is used to discover patterns, trends, and relationships in data.
It employs data visualization and other techniques to uncover insights that were not initially apparent.
This type of analysis is crucial for forming hypotheses and deciding on the directions of more formal analysis.
4. **Diagnostic Analysis:** Diagnostic analysis explores the underlying causes of trends and patterns identified in the data.
It aims to uncover the ‘why’ behind a phenomenon, offering a deeper understanding of the causes of specific changes in data.
5. **Predictive Analysis:** This type of analysis uses historical data to make predictions about future outcomes.
It often involves statistical modeling, machine learning, and artificial intelligence to forecast trends.
Predictive analysis is widely used in various fields, from finance to healthcare.
Predictive Modeling Explained
Predictive modeling is a crucial part of predictive analysis.
It involves creating, testing, and validating a model to predict future outcomes based on historical data.
Predictive models can provide valuable insights and forecasts, assisting researchers and organizations in planning and decision-making.
Components of Predictive Modeling
Predictive modeling involves several key components and steps, including:
1. **Problem Definition:** Clearly defining what you are trying to predict and the questions you hope to answer through the model.
This step sets the direction for your modeling efforts.
2. **Data Collection and Preparation:** Gathering relevant data that will be used to inform the model.
This step includes cleaning the data, handling missing values, and preparing it for analysis.
3. **Model Selection:** Choosing the appropriate modeling technique to suit the data and problem at hand.
Common models include linear regression, decision trees, neural networks, and support vector machines.
4. **Training the Model:** Using a subset of the data (training set) to build the model, helping it learn patterns and relationships from the data.
5. **Validation and Testing:** Evaluating the model’s performance using a different subset of data (validation and test sets) to ensure it makes accurate predictions.
This step may also involve tuning model parameters to improve its performance.
6. **Deployment and Monitoring:** Implementing the model in real-world scenarios and continuously monitoring its performance.
Over time, models may require updates and re-training with new data to maintain accuracy.
Applications of Predictive Modeling
Predictive modeling is versatile and has applications across various research fields:
1. **Healthcare:** Predictive models in healthcare can predict patient outcomes, identify at-risk patients, optimize treatment plans, and improve overall care quality.
With accurate predictions, healthcare providers can make proactive decisions that enhance patient care and reduce costs.
2. **Finance:** In finance, predictive models are used to forecast market trends, assess credit risk, and develop investment strategies.
These models help financial institutions make informed decisions, manage risks, and maximize profits.
3. **Marketing:** Marketers use predictive modeling to analyze consumer behavior, target specific audiences, and personalize marketing campaigns.
This leads to more effective marketing strategies and higher return on investment.
4. **Retail:** Retailers use predictive models to forecast demand, optimize inventory, and improve supply chain management.
By anticipating customer needs, businesses can enhance customer satisfaction and increase efficiency.
5. **Environmental Science:** Researchers in environmental science employ predictive modeling to predict climate change impacts, assess air and water quality, and develop conservation strategies.
These models provide valuable insights for sustainable environmental management.
Challenges in Data Analysis and Predictive Modeling
While data analysis and predictive modeling offer powerful insights, researchers face several challenges:
1. **Data Quality:** The accuracy of analysis and predictions heavily depends on data quality.
Inaccurate data can lead to misleading results and incorrect conclusions.
2. **Complexity:** Advanced statistical and machine learning techniques in predictive modeling can be complex and require a deep understanding of both the data and the tools used.
Researchers may need expertise to build and interpret these models effectively.
3. **Data Privacy:** Handling sensitive or personal data requires careful attention to privacy and ethical considerations.
Ensuring data protection is critical when collecting and analyzing data.
4. **Model Interpretability:** Often, complex models like deep learning or ensemble methods lack interpretability, making it difficult to understand how predictions are made.
Striking a balance between accuracy and transparency is crucial.
Conclusion
Data analysis and predictive modeling are invaluable tools for researchers across disciplines.
They offer profound insights by transforming raw data into actionable knowledge, enabling informed decision-making.
As data continues to grow in volume and complexity, mastering these techniques becomes essential for researchers.
While challenges exist, advancements in technology and statistical methods provide researchers with ever-improving resources for analysis and modeling.
Those who adeptly handle these processes stand to gain a competitive edge in their respective fields, unearthing new insights and driving innovation.
資料ダウンロード
QCD調達購買管理クラウド「newji」は、調達購買部門で必要なQCD管理全てを備えた、現場特化型兼クラウド型の今世紀最高の購買管理システムとなります。
ユーザー登録
調達購買業務の効率化だけでなく、システムを導入することで、コスト削減や製品・資材のステータス可視化のほか、属人化していた購買情報の共有化による内部不正防止や統制にも役立ちます。
NEWJI DX
製造業に特化したデジタルトランスフォーメーション(DX)の実現を目指す請負開発型のコンサルティングサービスです。AI、iPaaS、および先端の技術を駆使して、製造プロセスの効率化、業務効率化、チームワーク強化、コスト削減、品質向上を実現します。このサービスは、製造業の課題を深く理解し、それに対する最適なデジタルソリューションを提供することで、企業が持続的な成長とイノベーションを達成できるようサポートします。
オンライン講座
製造業、主に購買・調達部門にお勤めの方々に向けた情報を配信しております。
新任の方やベテランの方、管理職を対象とした幅広いコンテンツをご用意しております。
お問い合わせ
コストダウンが利益に直結する術だと理解していても、なかなか前に進めることができない状況。そんな時は、newjiのコストダウン自動化機能で大きく利益貢献しよう!
(Β版非公開)