- お役立ち記事
- Surrogate Modeling and CAE Design for Analysis Applications
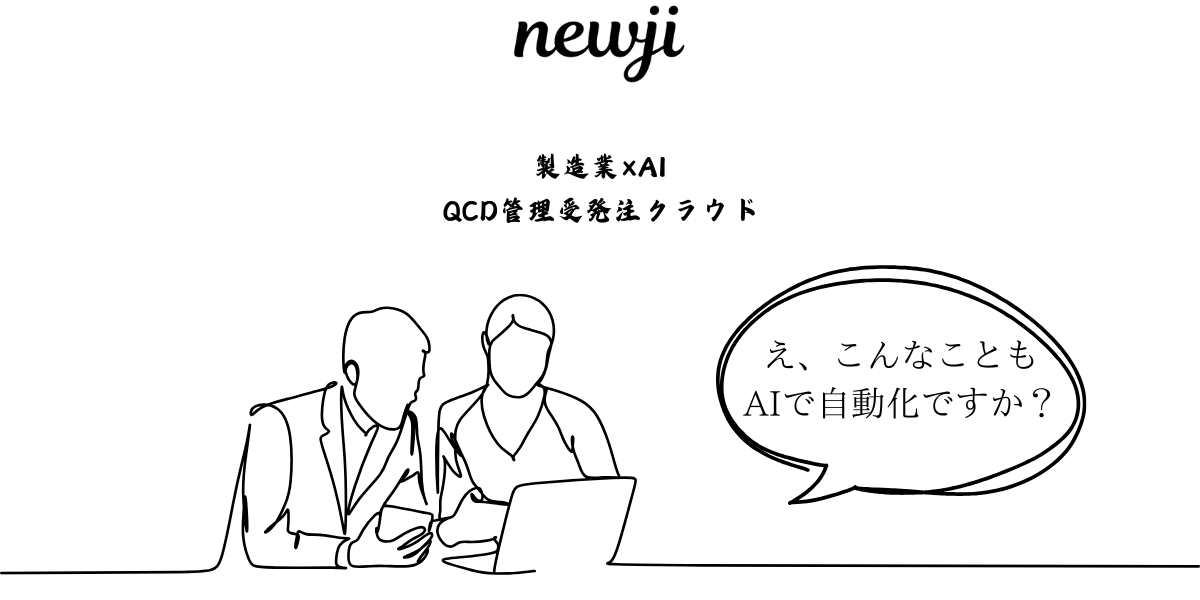
Surrogate Modeling and CAE Design for Analysis Applications
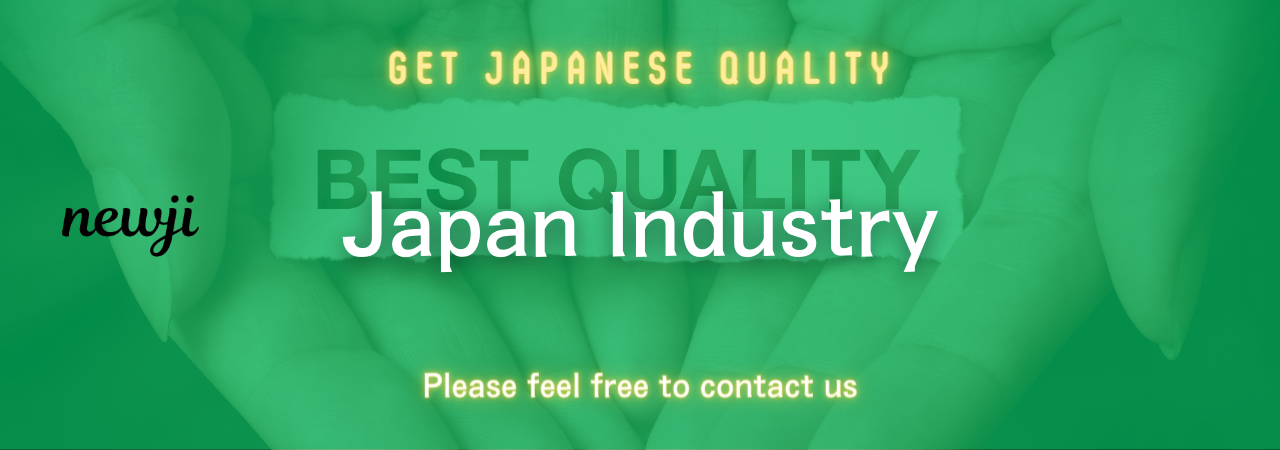
目次
Introduction to Surrogate Modeling
Surrogate modeling has become a vital tool in engineering and design applications, particularly in computer-aided engineering (CAE) design.
Surrogate models, sometimes called metamodels, are used to approximate complex simulations or processes that are otherwise computationally expensive.
These models are crafted to predict outcomes effectively, making them invaluable in design optimization, uncertainty quantification, and sensitivity analysis.
The need for surrogate modeling arises from the demand for rapid prototyping and evaluation, allowing engineers to explore a broad design space efficiently.
By using these models, designers can achieve accurate results without bearing the computational burden of high-fidelity simulations.
Understanding CAE Design
CAE Design, short for Computer-Aided Engineering Design, is the process of using computer software to aid in engineering tasks.
These tasks include simulation, validation, and optimization of products and processes.
CAE tools often involve solutions for fluid dynamics, structural analysis, and thermal management.
With the ongoing evolution of technology, CAE design has become integral to the engineering design process.
It allows for faster development, reduced costs, and improved product designs, all while ensuring a robust and safe final product.
By integrating surrogate modeling with CAE design, engineers can simulate and iterate designs more effectively, optimizing their processes and ensuring design integrity.
Importance of Surrogate Modeling in CAE
Surrogate models play a critical role in the CAE environment by offering approximations that are much faster to compute.
This speed is crucial when it comes to design iterations and optimizations.
When engineers face complex systems that require numerous simulations over vast design spaces, surrogate models provide quick, reliable insights.
This capability reduces the number of full-scale simulations needed, saving both time and resources.
Additionally, surrogate modeling facilitates understanding of how different parameters impact system performance, leading to optimized designs that meet specified criteria.
Through efficient approximations, engineers can confidently make decisions, knowing that the surrogate models reflect the underlying systems accurately.
Types of Surrogate Models
There are several types of surrogate models, each serving specific purposes based on the complexity and nature of the problem at hand.
A few common types include polynomial regression, kriging models, radial basis functions, and neural networks.
Polynomial regression is often used for simpler models due to its straightforward implementation and interpretation.
Kriging models, popular in geostatistics, offer flexibility and adaptability in modeling complex surfaces.
Radial basis functions, on the other hand, are useful for interpolating data and capturing non-linear relationships.
Neural networks, inspired by the human brain, can model highly non-linear and complex systems but require substantial data for training.
Each type provides unique advantages and trade-offs, making it essential for designers to choose the right surrogate model for their specific application needs.
Integrating Surrogate Models in CAE Workflows
To leverage the power of surrogate models in CAE workflows, it’s crucial to integrate them seamlessly into the design process.
This integration often begins with selecting the appropriate surrogate model type based on the specific simulations and problems.
Data collection follows, ensuring that sufficient and representative data informs the model training.
Once the model is developed, it’s validated against actual simulation results to ensure accuracy and reliability.
This validation step is critical, as it establishes confidence in the surrogate’s ability to predict new scenarios.
After validation, the surrogate model becomes a tool for optimization, testing different design variables and conditions efficiently.
Engineers can then use the rapid predictions to iterate designs, meet constraints, and improve performance.
Such integration helps streamline the entire CAE process, enhancing productivity and innovation.
Challenges in Surrogate Modeling
Though surrogate modeling presents clear advantages, several challenges must be navigated.
One primary challenge is ensuring model accuracy, especially when limited data is available.
Insufficient training data can lead to poor model performance, rendering it unreliable.
Moreover, capturing the true physics of complex systems can be difficult, often requiring sophisticated models that are computationally expensive to train.
Another challenge is the selection of an appropriate surrogate model type, as each type has its strengths and weaknesses.
Incorrect choice can lead to suboptimal approximations, reducing the effectiveness of simulations.
Lastly, incorporating surrogate models into existing workflows may require substantial changes in how simulations and designs are approached, posing a barrier for organizations reluctant to adapt.
The Future of Surrogate Modeling in CAE
The field of surrogate modeling continues to evolve, driven by advances in machine learning and artificial intelligence.
These technologies promise to bolster the capabilities of surrogate models, allowing them to handle more complex simulations with greater accuracy.
As computational power increases and data availability grows, surrogate models will become even more integrated into CAE processes.
Future developments may include automated model selection, where algorithms choose the optimal surrogate model based on the problem and available data.
Furthermore, real-time applications of surrogate modeling are likely to emerge, enabling on-the-fly design optimization and decision-making.
As engineers face increasingly complex design challenges, surrogate modeling will be essential in delivering efficient, cost-effective solutions.
By embracing these advancements, the engineering field will sustain its trajectory towards innovation and excellence.
In conclusion, surrogate modeling offers substantial opportunities in CAE design, enabling efficient exploration of design spaces and optimization.
Despite challenges, the integration of these models holds promise for future advancements and streamlined engineering processes.
資料ダウンロード
QCD調達購買管理クラウド「newji」は、調達購買部門で必要なQCD管理全てを備えた、現場特化型兼クラウド型の今世紀最高の購買管理システムとなります。
ユーザー登録
調達購買業務の効率化だけでなく、システムを導入することで、コスト削減や製品・資材のステータス可視化のほか、属人化していた購買情報の共有化による内部不正防止や統制にも役立ちます。
NEWJI DX
製造業に特化したデジタルトランスフォーメーション(DX)の実現を目指す請負開発型のコンサルティングサービスです。AI、iPaaS、および先端の技術を駆使して、製造プロセスの効率化、業務効率化、チームワーク強化、コスト削減、品質向上を実現します。このサービスは、製造業の課題を深く理解し、それに対する最適なデジタルソリューションを提供することで、企業が持続的な成長とイノベーションを達成できるようサポートします。
オンライン講座
製造業、主に購買・調達部門にお勤めの方々に向けた情報を配信しております。
新任の方やベテランの方、管理職を対象とした幅広いコンテンツをご用意しております。
お問い合わせ
コストダウンが利益に直結する術だと理解していても、なかなか前に進めることができない状況。そんな時は、newjiのコストダウン自動化機能で大きく利益貢献しよう!
(Β版非公開)