- お役立ち記事
- Basics of Dispersion and Aggregation Techniques: Selection and Troubleshooting
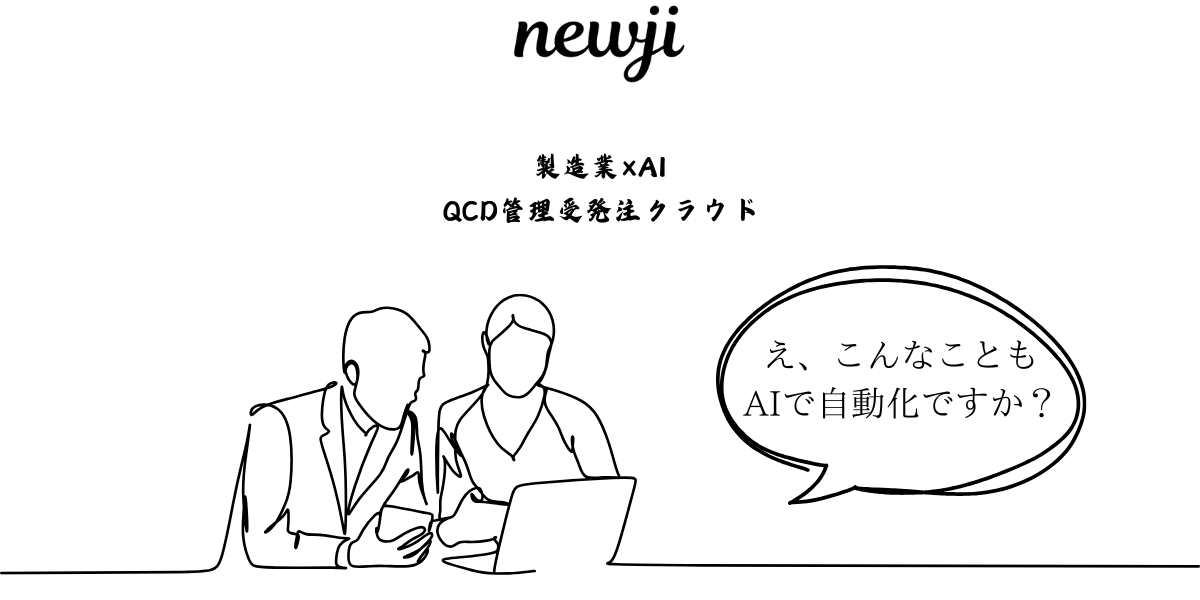
Basics of Dispersion and Aggregation Techniques: Selection and Troubleshooting
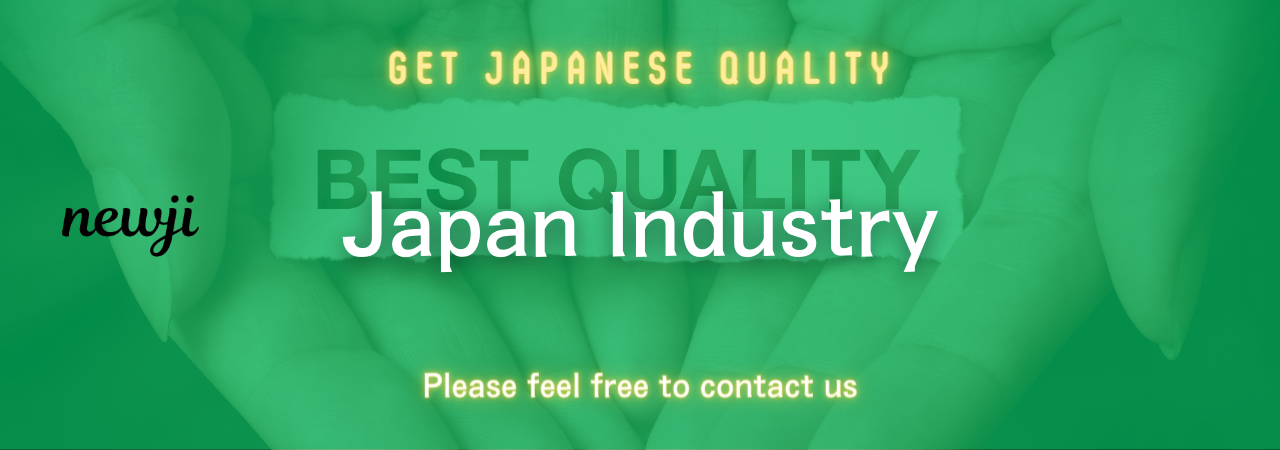
目次
Understanding Dispersion and Aggregation Techniques
Dispersion and aggregation are fundamental concepts in data analysis and processing.
They play a critical role in how data is manipulated, interpreted, and presented.
In simple terms, dispersion refers to the spread of data points across a dataset, while aggregation is the process of combining or summarizing this data into a more comprehensive form.
Understanding these techniques is crucial for anyone working with data, as they directly impact the quality of insights that can be drawn.
What is Dispersion?
Dispersion measures how much data points differ from each other and from the central value, such as the mean.
It provides insights into the variability or diversity within a dataset.
There are several methods to measure dispersion, including:
1. **Range**: This is the simplest form of dispersion which is calculated as the difference between the maximum and minimum values in a dataset.
Though easy to compute, the range only considers two points and can be affected by outliers.
2. **Variance**: This metric signifies the average squared deviation of each data point from the mean.
Variance gives a more accurate picture of dispersion as it uses all data points in its calculation.
3. **Standard Deviation**: This is the square root of the variance and is in the same unit as the original data.
It offers a more intuitive understanding of dispersion by showing the average distance from the mean.
What is Aggregation?
Aggregation summarizes multiple data points and is crucial in data simplification and analysis.
It helps in understanding large volumes of data by considering key metrics.
Common forms of aggregation include:
1. **Sum**: Adding all data points together provides a total that can be essential in financial analyses and other applications.
2. **Mean**: As the average of all data points, the mean gives a central tendency that is significant in various statistical analyses.
3. **Median**: By arranging data points in ascending order and identifying the midpoint, the median provides a measure of central tendency that is resistant to outliers.
4. **Mode**: This is the most frequently occurring value in a dataset and is useful when dealing with categorical data.
Choosing the Right Technique
Selecting the appropriate dispersion and aggregation techniques depends on your specific data analysis needs and the nature of your data.
Here are some factors to consider:
Data Type
The choice of technique should align with the data type you are working with.
For example, if you are working with numerical data, you might choose variance or standard deviation to understand dispersion.
If dealing with categorical data, mode might be more meaningful than mean or median.
Data Distribution
If your data is normally distributed, using the mean and standard deviation is typically appropriate.
However, in the presence of outliers or skewed data, the median might provide a better measure of central tendency due to its robustness to such anomalies.
Objective of Analysis
Your analysis objective also dictates the technique choice.
If you need to understand variability for risk assessment, measuring dispersion through variance or standard deviation might be crucial.
On the other hand, if you need an overall summary of data, aggregation techniques like sum or mean are more relevant.
Troubleshooting Common Issues
Working with dispersion and aggregation techniques might present some challenges.
Here’s how to troubleshoot common issues:
Handling Outliers
Outliers can significantly distort your data analysis, especially when calculating the mean or variance.
Consider using the median for central tendency, which is less affected by outliers, or apply data-cleaning techniques to remove them.
Skewed Data Distributions
Skewed distributions can make standard deviation an unreliable measure of spread.
It might be more appropriate to use the interquartile range, which provides insight into the central 50% of your data.
Data with Missing Values
Missing data can impact the accuracy of your analysis.
Ensure to handle missing values by using methods such as data imputation or removing incomplete records, depending on the dataset’s nature and analysis requirements.
Avoiding Misinterpretation
When reporting processed data, ensure accuracy by clearly explaining the methods and measures used.
This transparency helps in avoiding misinterpretations and provides a basis for sound decision-making.
Conclusion
Understanding dispersion and aggregation techniques is essential for effectively tackling data analysis tasks.
By choosing the right methods based on your data characteristics and analysis goals, you can extract meaningful insights from datasets.
Being aware of potential issues and taking proactive measures to address them will further enhance the quality and reliability of your analysis.
資料ダウンロード
QCD調達購買管理クラウド「newji」は、調達購買部門で必要なQCD管理全てを備えた、現場特化型兼クラウド型の今世紀最高の購買管理システムとなります。
ユーザー登録
調達購買業務の効率化だけでなく、システムを導入することで、コスト削減や製品・資材のステータス可視化のほか、属人化していた購買情報の共有化による内部不正防止や統制にも役立ちます。
NEWJI DX
製造業に特化したデジタルトランスフォーメーション(DX)の実現を目指す請負開発型のコンサルティングサービスです。AI、iPaaS、および先端の技術を駆使して、製造プロセスの効率化、業務効率化、チームワーク強化、コスト削減、品質向上を実現します。このサービスは、製造業の課題を深く理解し、それに対する最適なデジタルソリューションを提供することで、企業が持続的な成長とイノベーションを達成できるようサポートします。
オンライン講座
製造業、主に購買・調達部門にお勤めの方々に向けた情報を配信しております。
新任の方やベテランの方、管理職を対象とした幅広いコンテンツをご用意しております。
お問い合わせ
コストダウンが利益に直結する術だと理解していても、なかなか前に進めることができない状況。そんな時は、newjiのコストダウン自動化機能で大きく利益貢献しよう!
(Β版非公開)