- お役立ち記事
- A practical guide to improving manufacturing reliability with AI quality control
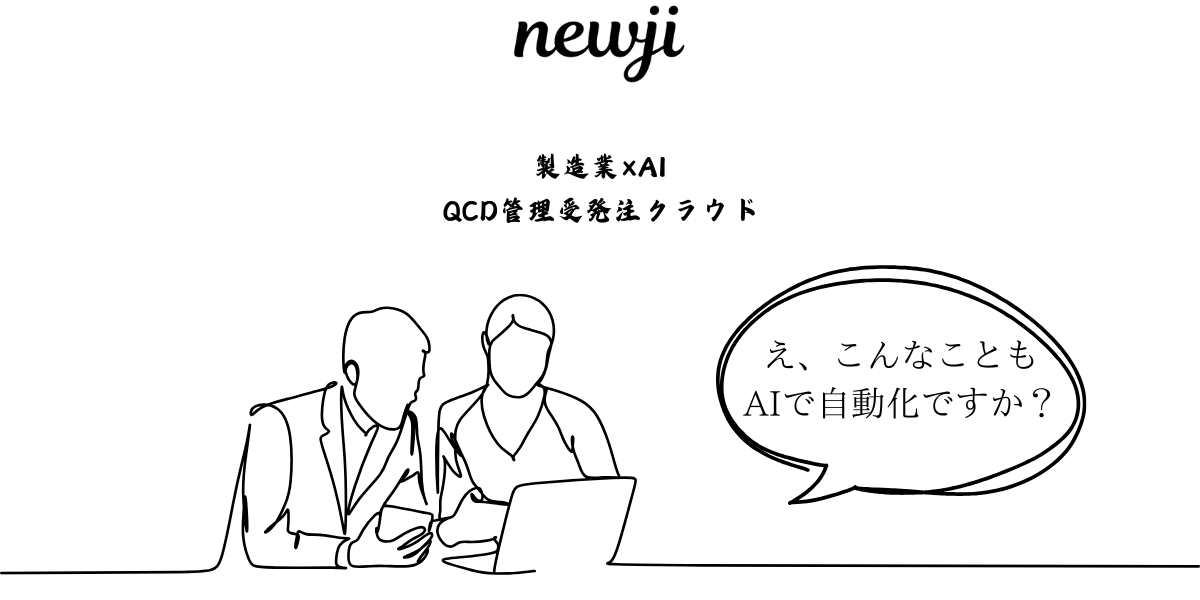
A practical guide to improving manufacturing reliability with AI quality control
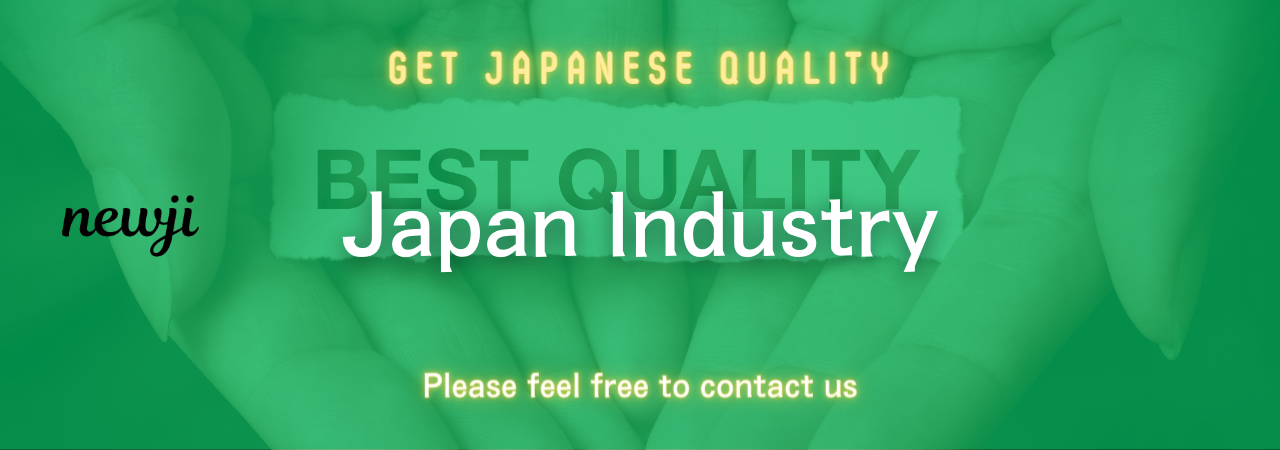
目次
Understanding AI Quality Control
Manufacturing is the backbone of industry, providing the goods and services we rely on daily.
The process demands precision, efficiency, and reliability, making quality control crucial.
The advent of Artificial Intelligence (AI) in quality control has transformed the way manufacturers approach these methods, bringing more precision and efficiency to the system.
AI quality control refers to the use of algorithms and machine learning models to monitor and improve the quality of products throughout the manufacturing process.
This innovative technology can analyze data from various sources such as sensors, cameras, and other quality inspection devices, to locate defects and anomalies in real time.
The Role of AI in Quality Control
AI plays several significant roles in improving manufacturing reliability.
Its capacity for vast data processing and pattern recognition means AI can detect inconsistencies that may escape human observation.
AI algorithms can be trained to identify specific product flaws by learning from historical data of defects.
These machine learning models develop an understanding of ‘normal’ versus ‘defective’ product patterns, thereby efficiently identifying issues on the manufacturing line.
The speed at which AI processes information means that problems can be detected and rectified swiftly, reducing downtime and maintaining the smooth running of operations.
Enhanced accuracy in detecting errors ensures fewer defective products reach the consumer, safeguarding brand reputation and reducing costs associated with returns and repairs.
Benefits of AI Quality Control
The integration of AI in quality control offers numerous benefits.
Firstly, it enhances precision by minimizing human error.
Humans are prone to fatigue and oversight, particularly in repetitive tasks, whereas AI systems consistently apply rigorous standards without being subject to these limitations.
Secondly, AI brings a significant improvement in the speed of quality inspections.
Traditional methods might take considerable time, but AI enables near-instantaneous analysis.
This rapid processing not only accelerates production lines but also allows for the immediate correction of mistakes, minimizing waste and inefficiencies.
Moreover, AI quality control systems can predict defects before they occur.
Using predictive analytics, these systems can foresee areas of potential failure within a process, allowing for proactive measures to address these issues.
This anticipatory approach not only prevents defects but also maintains a high level of product consistency and reliability.
Implementing AI in Manufacturing
Implementing AI in quality control involves several steps.
To start, manufacturers need to identify the processes or areas where quality control improvements are most needed.
This may involve assessing where manual inspections frequently fail or where the most significant defects occur.
Next, collecting comprehensive data is crucial.
Quality data serves as the foundation upon which AI models are built.
This information can be sourced from physical inspections, sensor outputs, camera feeds, and historical logs.
Ensuring data quality and preparing it for initial analysis is essential to train the AI system effectively.
Following data collection, the appropriate AI system and tools must be selected.
This could range from third-party software solutions to customized AI models developed in-house.
The choice will depend on the manufacturer’s specific needs, scale of operations, and existing technological infrastructure.
Training the AI system comes next, involving inputting data and teaching the model to recognize defects.
Ongoing iteration, testing, and validation are crucial at this stage to ensure the AI operates accurately and efficiently.
Lastly, integration into existing systems and infrastructure should be seamless, allowing AI quality control to work alongside human workers for optimal results.
Ongoing support and updates are also necessary to adjust to evolving production requirements and technological advancements.
Challenges of AI in Quality Control
Despite its advantages, integrating AI into manufacturing does pose some challenges.
Foremost is the initial investment cost.
AI systems require substantial investment in both software and hardware.
However, these costs are often offset by long-term savings and efficiency improvements.
Additionally, the transition to AI may face resistance from employees accustomed to conventional methods.
Training programs and demonstrations of the benefits AI can offer are essential to gaining worker buy-in.
Another challenge is ensuring data accuracy.
AI systems are heavily reliant on data, and any inaccuracies in the initial data sets can lead to incorrect analyses and defect identification.
Continuous monitoring and cleaning of data are necessary to maintain high operational standards.
Finally, cybersecurity risks must be managed vigilantly.
As manufacturing processes become more digital and interconnected, ensuring the protection of data and systems from potential breaches or cyberattacks is vital.
Success Stories and Future Prospects
Numerous companies have successfully implemented AI in quality control, seeing tangible improvements in reliability and efficiency.
For instance, large automobile manufacturers have adopted AI systems to reduce defect rates, thus enhancing the longevity and performance of their vehicles.
Similarly, electronics manufacturers use AI to assess and improve circuitry, ensuring product robustness and durability.
Looking to the future, AI quality control will likely expand with advancements in machine learning, computer vision, and data analytics.
Integration with the Internet of Things (IoT) will enable even more data-driven decision-making, further refining manufacturing processes and outcomes.
AI’s role in manufacturing is poised only to grow, promising improved product reliability, enhanced consumer satisfaction, and a significant competitive edge in the market.
Embracing AI quality control is not just a trend but a necessity for manufacturers aiming to thrive in an increasingly complex and demanding industry landscape.
資料ダウンロード
QCD調達購買管理クラウド「newji」は、調達購買部門で必要なQCD管理全てを備えた、現場特化型兼クラウド型の今世紀最高の購買管理システムとなります。
ユーザー登録
調達購買業務の効率化だけでなく、システムを導入することで、コスト削減や製品・資材のステータス可視化のほか、属人化していた購買情報の共有化による内部不正防止や統制にも役立ちます。
NEWJI DX
製造業に特化したデジタルトランスフォーメーション(DX)の実現を目指す請負開発型のコンサルティングサービスです。AI、iPaaS、および先端の技術を駆使して、製造プロセスの効率化、業務効率化、チームワーク強化、コスト削減、品質向上を実現します。このサービスは、製造業の課題を深く理解し、それに対する最適なデジタルソリューションを提供することで、企業が持続的な成長とイノベーションを達成できるようサポートします。
オンライン講座
製造業、主に購買・調達部門にお勤めの方々に向けた情報を配信しております。
新任の方やベテランの方、管理職を対象とした幅広いコンテンツをご用意しております。
お問い合わせ
コストダウンが利益に直結する術だと理解していても、なかなか前に進めることができない状況。そんな時は、newjiのコストダウン自動化機能で大きく利益貢献しよう!
(Β版非公開)