- お役立ち記事
- How to use AI predictive models to support process improvement in the manufacturing industry
How to use AI predictive models to support process improvement in the manufacturing industry
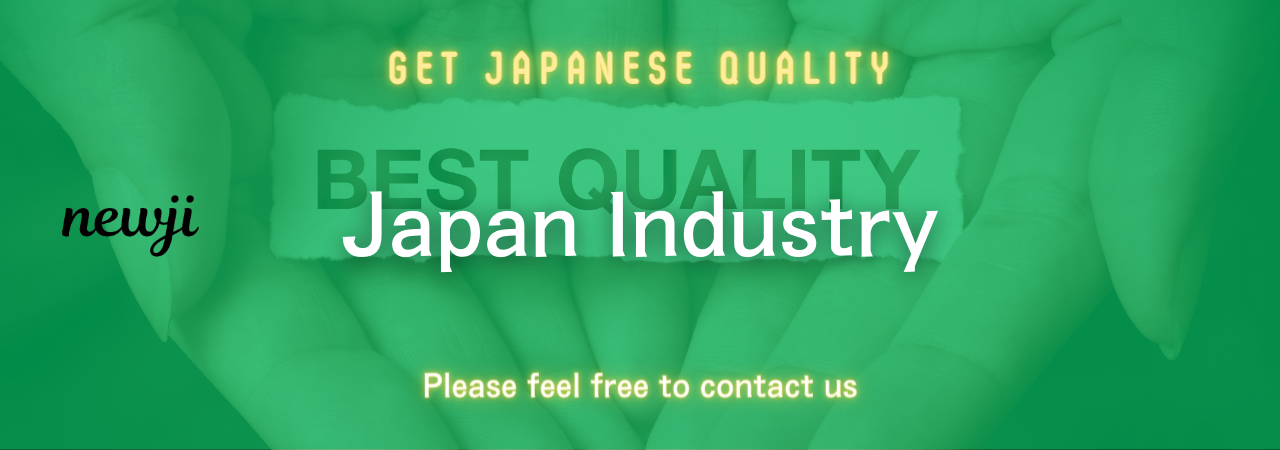
目次
Understanding AI Predictive Models
AI predictive models are powerful tools engineered to analyze patterns and forecast potential outcomes based on existing data.
These models are developed using various machine learning algorithms that study historical data to predict future trends and behaviors.
In the manufacturing industry, AI predictive models can transform operations by offering data-driven insights that support process improvement.
Manufacturing involves numerous processes and stages, from production to quality assurance.
Traditional methods of monitoring these processes can be time-consuming and prone to errors.
AI predictive models, however, provide an efficient alternative by predicting potential bottlenecks and suggesting improvements.
The Role of AI in Process Improvement
Process improvement in manufacturing is critical for enhancing productivity, reducing costs, and maintaining product quality.
AI predictive models support these goals in several ways.
Predictive Maintenance
One of the primary applications of AI in manufacturing is predictive maintenance.
AI models analyze data from machinery to predict when maintenance is needed, preventing unexpected breakdowns.
This proactive approach not only prevents costly downtime but also extends the lifespan of equipment.
By forecasting potential failures, manufacturers can schedule maintenance during planned shutdowns, thus minimizing disruption to production.
Supply Chain Optimization
AI predictive models can analyze supply chain data to enhance efficiency.
Models can forecast demand, anticipate supply chain disruptions, and optimize inventory levels.
This leads to a smoother supply chain operation, reduced waste, and ultimately, cost savings.
Quality Control
Maintaining high product quality is paramount in manufacturing.
AI predictive models help identify factors that could lead to quality issues by analyzing production line data.
The models can recognize patterns that indicate defects, allowing for early intervention before poor-quality products reach the market.
Implementing AI in quality control leads to fewer product recalls, happier customers, and a stronger brand reputation.
Steps to Implement AI Predictive Models in Manufacturing
Adopting AI predictive models in your manufacturing processes requires several steps.
Identify Opportunities
Start by identifying areas within your manufacturing processes that could benefit from AI predictive models.
This could include production, maintenance schedules, supply chain logistics, or quality control.
Understanding the specific challenges you face will help tailor the AI solution to meet your needs.
Data Collection and Preprocessing
AI models rely on high-quality data.
Ensure your data collection systems are robust and gather comprehensive data from relevant processes.
Once collected, data needs to be preprocessed, which involves cleaning, organizing, and formatting it for use in AI models.
Choosing the Right AI Model
There are various machine learning algorithms, from simple linear regression to complex neural networks.
The choice of model depends on the complexity of the task and the nature of the data.
Expert guidance can help select the most suitable model for your needs.
Model Training and Validation
Once selected, the model must be trained using historical data.
During training, the model learns to recognize patterns and make predictions.
After training, validate the model’s performance to ensure its accuracy before full implementation.
Integration and Monitoring
Integrate the AI predictive model into your existing systems.
Continuous monitoring is essential; it ensures the model remains accurate over time and adapts to changing conditions.
Regularly update the model with new data to refine its predictive capability.
Overcoming Challenges in AI Implementation
Implementing AI predictive models in manufacturing poses several challenges.
Data Quality
Poor data quality can lead to inaccurate predictions.
It’s vital to invest in high-quality data collection methods and systems for optimal results.
Technology Infrastructure
Modern IT infrastructure is essential for running AI models efficiently.
Manufacturers must ensure their systems are equipped to handle the computational demands of AI.
Change Management
Introducing AI requires a shift in operations and possibly corporate culture.
Effective change management strategies should be in place to ensure a smooth transition and staff buy-in.
The Future of AI in Manufacturing
The integration of AI in manufacturing is set to revolutionize the industry.
AI predictive models will continue to improve, offering even greater accuracy and insights.
Manufacturers that adopt these models will be positioned to gain a competitive edge, able to offer higher quality products at lower costs.
AI holds the promise of not only optimizing current operations but also leading to innovative manufacturing processes through continuous improvement and adaptation.
In summary, leveraging AI predictive models is a strategic decision that can transform the manufacturing landscape.
With careful planning and execution, these models can lead to significant process improvements, resulting in enhanced efficiency and sustained business growth.
資料ダウンロード
QCD調達購買管理クラウド「newji」は、調達購買部門で必要なQCD管理全てを備えた、現場特化型兼クラウド型の今世紀最高の購買管理システムとなります。
ユーザー登録
調達購買業務の効率化だけでなく、システムを導入することで、コスト削減や製品・資材のステータス可視化のほか、属人化していた購買情報の共有化による内部不正防止や統制にも役立ちます。
NEWJI DX
製造業に特化したデジタルトランスフォーメーション(DX)の実現を目指す請負開発型のコンサルティングサービスです。AI、iPaaS、および先端の技術を駆使して、製造プロセスの効率化、業務効率化、チームワーク強化、コスト削減、品質向上を実現します。このサービスは、製造業の課題を深く理解し、それに対する最適なデジタルソリューションを提供することで、企業が持続的な成長とイノベーションを達成できるようサポートします。
オンライン講座
製造業、主に購買・調達部門にお勤めの方々に向けた情報を配信しております。
新任の方やベテランの方、管理職を対象とした幅広いコンテンツをご用意しております。
お問い合わせ
コストダウンが利益に直結する術だと理解していても、なかなか前に進めることができない状況。そんな時は、newjiのコストダウン自動化機能で大きく利益貢献しよう!
(Β版非公開)