- お役立ち記事
- Small data analysis in machine learning, its key points, and application to solving real problems
月間77,185名の
製造業ご担当者様が閲覧しています*
*2025年2月28日現在のGoogle Analyticsのデータより
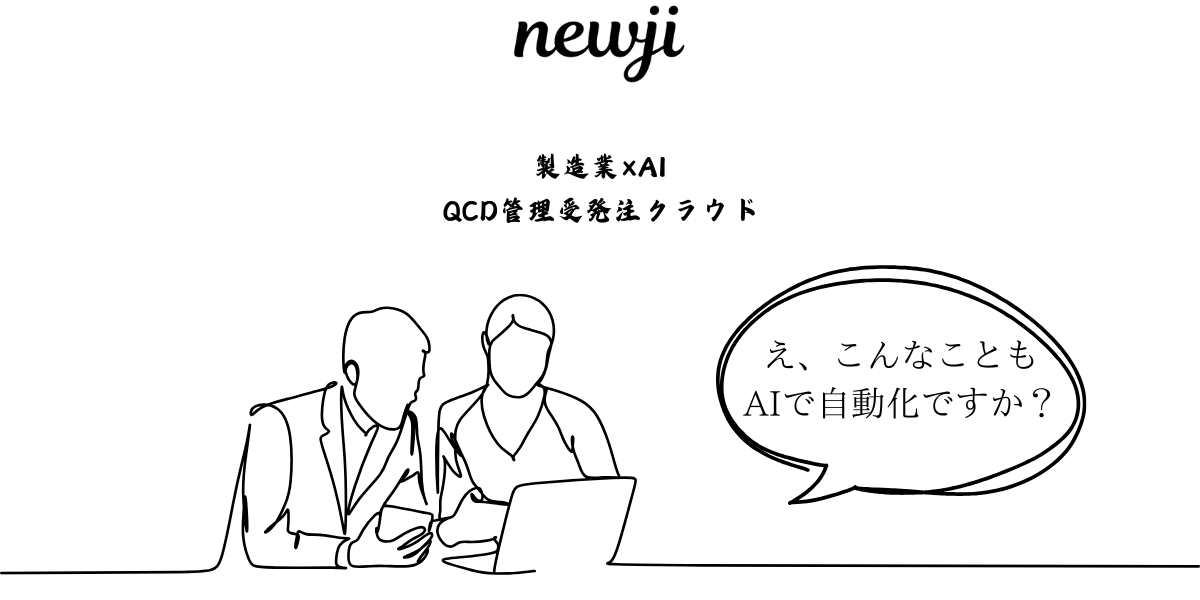
Small data analysis in machine learning, its key points, and application to solving real problems
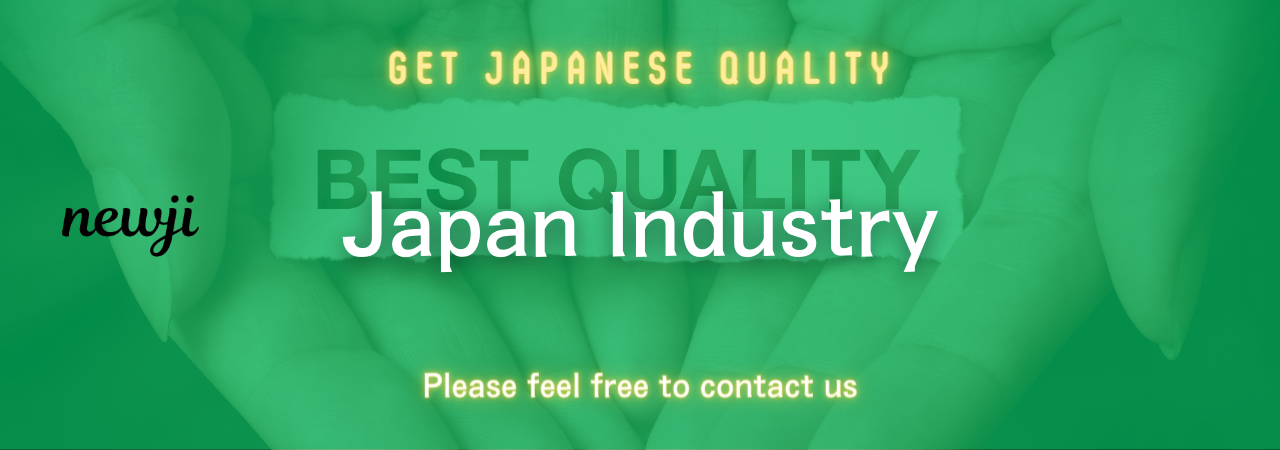
目次
Introduction to Small Data Analysis
In the realm of machine learning, data is key.
While large datasets often grab the spotlight, small data analysis plays an equally vital role, especially when resources are limited or the data is inherently sparse.
In this article, we will explore the key points of small data analysis and its application to solving real-world problems.
Understanding how to effectively analyze and utilize small datasets can lead to innovative solutions and insights.
What is Small Data?
Small data refers to datasets that are limited in size.
Unlike big data, which involves massive volumes of structured or unstructured data, small data focuses on sifted, precise, and manageable data.
This type of data is often easier to analyze and use for decision-making as it provides clear insights that can be acted upon.
Small data sets are common in scenarios where collecting data can be challenging, such as in medical research with rare diseases, niche market analysis, or when operational constraints restrict data collection.
Challenges of Small Data in Machine Learning
Machine learning models typically perform better with large amounts of data; however, this is not always feasible.
Here are some challenges that arise when dealing with small data:
Data Sparsity
With small datasets, data sparsity is a significant issue.
This refers to the lack of data points across many possible input spaces, which can impede the learning process of algorithms.
Overfitting
Overfitting occurs when a model learns the details and noise in the training data to an extent that it negatively impacts the performance on new data.
This risk is heightened with small datasets because the model can misinterpret nuances as genuine patterns, leading to poor generalization on unseen data.
Model Selection
Choosing the right model is critical when dealing with small datasets.
Complex models like deep neural networks may not be suitable due to their high data requirement; instead, simpler models like linear regression or decision trees might be more appropriate.
Strategies for Small Data Analysis
While small data presents challenges, several strategies can be employed to maximize its utility:
Data Augmentation
Data augmentation involves creating new data points from the existing data through transformations such as rotation, scaling, translation, or by adding noise.
This approach helps to enlarge the dataset without collecting more data.
Transfer Learning
Transfer learning leverages the knowledge from models trained on large datasets to improve performance on small data tasks.
By fine-tuning a pre-trained model on a smaller related dataset, transfer learning allows for efficient use of limited data.
Cross-Validation
Cross-validation is essential in small data analysis as it allows all the available data to be used for training and testing, providing a reliable estimate of the model’s performance.
K-fold and leave-one-out cross-validation are common techniques used to mitigate overfitting risks in small datasets.
Regularization Techniques
Regularization techniques, such as L1 and L2 regularization, are designed to prevent overfitting by adding a penalty to the loss function.
These techniques can enhance model generalization on unseen data.
Applications of Small Data Analysis
Small data analysis has numerous practical applications in various fields:
Healthcare
In healthcare, small data can be used to improve decision-making processes in scenarios where large datasets are unavailable.
For instance, analyzing patient data from rare diseases can help in diagnostics and forming personalized treatment plans.
Finance
In finance, small data can be instrumental in assessing credit risk for niche borrower segments or developing algorithms for trading low-frequency data.
Marketing
Small data analysis aids marketers in understanding consumer behavior in niche markets.
By analyzing detailed customer interaction data, businesses can create personalized marketing strategies that better engage their audience.
Industrial Applications
In industries, small data is often used in predictive maintenance.
Machines and sensors may generate limited data, yet this information is crucial for predicting equipment failures and scheduling timely maintenance.
Conclusion
While big data has its advantages, small data analysis should not be overlooked in machine learning.
With the right strategies and tools, small data can provide significant insights and solutions to real-world problems.
Embracing small data analysis opens new avenues for innovation, especially in fields where large amounts of data are not feasible to acquire.
Regardless of the scale of your dataset, focusing on accurate data representation and model performance will ensure that valuable insights are extracted and utilized effectively.
資料ダウンロード
QCD管理受発注クラウド「newji」は、受発注部門で必要なQCD管理全てを備えた、現場特化型兼クラウド型の今世紀最高の受発注管理システムとなります。
ユーザー登録
受発注業務の効率化だけでなく、システムを導入することで、コスト削減や製品・資材のステータス可視化のほか、属人化していた受発注情報の共有化による内部不正防止や統制にも役立ちます。
NEWJI DX
製造業に特化したデジタルトランスフォーメーション(DX)の実現を目指す請負開発型のコンサルティングサービスです。AI、iPaaS、および先端の技術を駆使して、製造プロセスの効率化、業務効率化、チームワーク強化、コスト削減、品質向上を実現します。このサービスは、製造業の課題を深く理解し、それに対する最適なデジタルソリューションを提供することで、企業が持続的な成長とイノベーションを達成できるようサポートします。
製造業ニュース解説
製造業、主に購買・調達部門にお勤めの方々に向けた情報を配信しております。
新任の方やベテランの方、管理職を対象とした幅広いコンテンツをご用意しております。
お問い合わせ
コストダウンが利益に直結する術だと理解していても、なかなか前に進めることができない状況。そんな時は、newjiのコストダウン自動化機能で大きく利益貢献しよう!
(β版非公開)