- お役立ち記事
- Fundamentals of time series data analysis and application to prediction through modeling
Fundamentals of time series data analysis and application to prediction through modeling
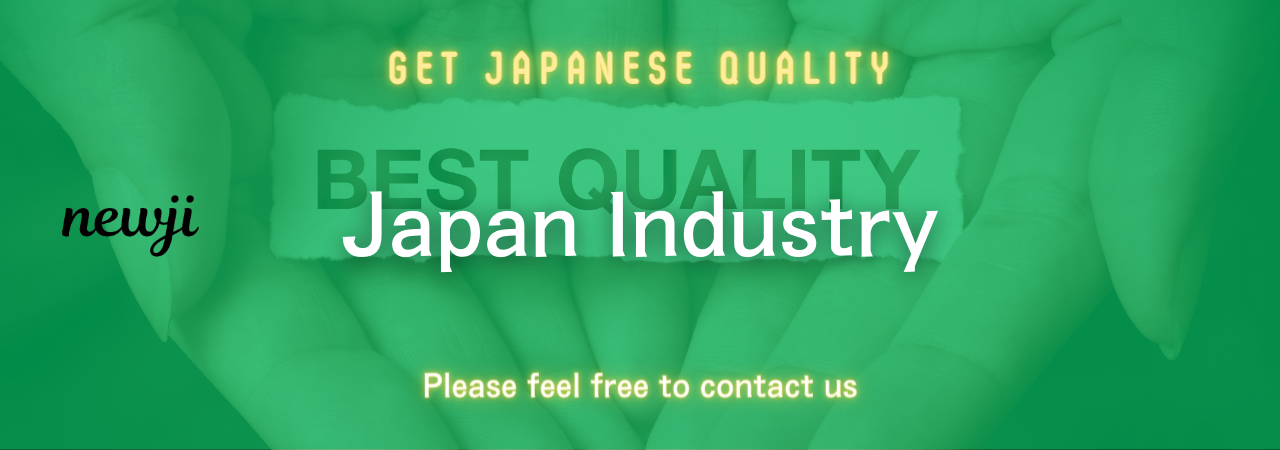
目次
Understanding Time Series Data
Time series data is a sequence of data points collected or recorded at specific time intervals.
These data points are typically arranged chronologically and can include anything from daily stock prices to monthly sales figures.
One of the primary characteristics of time series data is that it shows how the data evolves over time, which makes it unique and useful for predictive analysis.
A crucial aspect of time series is the time interval, which can be anything from seconds to years.
The choice of interval depends on the nature of the data and the objectives of the analysis.
Time series data is often used in fields such as finance, economics, environmental studies, and many more to forecast trends, seasonality, and other patterns.
Components of Time Series Data
Time series data can be broken down into various components, each contributing to the overall structure and pattern of the data.
Understanding these components is essential for effective analysis and modeling.
Trend
The trend is the long-term movement or direction in the data.
It indicates whether the data is increasing, decreasing, or remaining constant over time.
Identifying the trend is crucial for making accurate predictions and understanding the underlying patterns.
Seasonality
Seasonality refers to the regular, predictable fluctuations that recur at specific intervals due to seasonal factors.
For example, retail sales often increase during the holiday season.
Recognizing these patterns can help businesses plan and optimize their strategies according to expected changes.
Cyclical Patterns
Cyclical patterns are similar to seasonal effects but occur over longer periods.
These patterns are often influenced by economic cycles or other external factors.
While they are less predictable than seasonal patterns, identifying cycles can provide valuable insights into the data’s behavior over time.
Irregular Variations
Irregular variations are random or unforeseen changes in the data that do not follow a specific pattern.
These variations could be caused by events such as natural disasters, political events, or other unpredictable occurrences.
While it’s challenging to predict these variations, analyzing past events can help mitigate their impact.
Methods of Time Series Analysis
Several methods are employed to analyze time series data, each with its advantages and applications.
Selecting the right method depends on the dataset, analysis objectives, and the specific patterns present.
Decomposition
Decomposition is a technique used to separate a time series into its components, such as trend, seasonality, and irregular variations.
This method helps analysts understand the underlying factors influencing the series and is a foundational step for further analysis and modeling.
Smoothing
Smoothing techniques aim to remove noise and irregular variations from time series data to highlight underlying patterns.
Two common smoothing methods are moving averages and exponential smoothing.
These methods help analysts forecast future trends by focusing on the more stable components of the data.
Autoregressive Integrated Moving Average (ARIMA)
ARIMA is a widely used statistical modeling technique for time series forecasting.
It combines autoregressive (AR) and moving average (MA) components to model the time series.
ARIMA can effectively handle data with trends and seasonality but requires proper parameter selection, which can be complex.
Exponential Smoothing State Space Model (ETS)
The ETS method is another popular approach for forecasting time series data.
It models the data using an exponential smoothing technique and captures the error, trend, and seasonality components.
ETS models are relatively easy to use and can provide reliable forecasts, making them suitable for various time series datasets.
Applications of Time Series Analysis
Time series analysis is crucial across many industries to enhance decision-making and strategic planning.
Here are some common applications:
Finance and Economics
In finance, time series analysis is used to forecast stock prices, exchange rates, and financial indicators.
Economists also rely on time series data to study economic growth, inflation, and employment trends.
Accurate forecasts allow policy makers and investors to make informed decisions.
Retail and Marketing
Retailers use time series data to analyze sales patterns and inventory levels.
By identifying trends and seasonal effects, businesses can optimize their marketing campaigns, manage stock more efficiently, and improve customer satisfaction.
Supply Chain Management
Time series analysis plays a critical role in supply chain management by predicting demand and inventory requirements.
Accurate forecasts help companies maintain optimal stock levels, reduce costs, and improve delivery performance.
Environmental Science
Environmental scientists analyze time series data to monitor climate changes, weather patterns, and natural resource utilization.
Such analysis aids in identifying trends, predicting future changes, and implementing effective conservation measures.
Steps to Implement Time Series Prediction Models
Developing a time series prediction model involves several steps to ensure accuracy and reliability.
Data Collection and Preprocessing
Before modeling, it’s essential to collect high-quality data and preprocess it.
This includes handling missing values, smoothening noisy data, and converting the data into a consistent time format.
Exploratory Data Analysis
Performing exploratory analysis helps to uncover the underlying patterns and characteristics of the data.
This step involves visualizing the data and identifying trends, seasonality, and potential outliers.
Model Selection and Training
Selecting the appropriate model involves comparing different methods based on the dataset and analysis objectives.
Once selected, the model needs to be trained using a portion of the data to learn the underlying patterns.
Model Validation and Testing
Before deployment, it’s crucial to validate the model’s accuracy and reliability.
This process involves testing the model on unseen data and fine-tuning parameters to optimize performance.
Conclusion
Time series data analysis and modeling are fundamental techniques for making informed predictions across various fields.
By understanding the core components, methods, and applications, organizations can leverage time series analysis to make data-driven decisions and anticipate future trends effectively.
With continuous advancements in technology and data analysis techniques, the potential for time series analysis will only continue to grow, providing even more accurate and insightful predictions.
資料ダウンロード
QCD調達購買管理クラウド「newji」は、調達購買部門で必要なQCD管理全てを備えた、現場特化型兼クラウド型の今世紀最高の購買管理システムとなります。
ユーザー登録
調達購買業務の効率化だけでなく、システムを導入することで、コスト削減や製品・資材のステータス可視化のほか、属人化していた購買情報の共有化による内部不正防止や統制にも役立ちます。
NEWJI DX
製造業に特化したデジタルトランスフォーメーション(DX)の実現を目指す請負開発型のコンサルティングサービスです。AI、iPaaS、および先端の技術を駆使して、製造プロセスの効率化、業務効率化、チームワーク強化、コスト削減、品質向上を実現します。このサービスは、製造業の課題を深く理解し、それに対する最適なデジタルソリューションを提供することで、企業が持続的な成長とイノベーションを達成できるようサポートします。
オンライン講座
製造業、主に購買・調達部門にお勤めの方々に向けた情報を配信しております。
新任の方やベテランの方、管理職を対象とした幅広いコンテンツをご用意しております。
お問い合わせ
コストダウンが利益に直結する術だと理解していても、なかなか前に進めることができない状況。そんな時は、newjiのコストダウン自動化機能で大きく利益貢献しよう!
(Β版非公開)