- お役立ち記事
- Data utilization techniques to streamline maintenance planning in the manufacturing industry
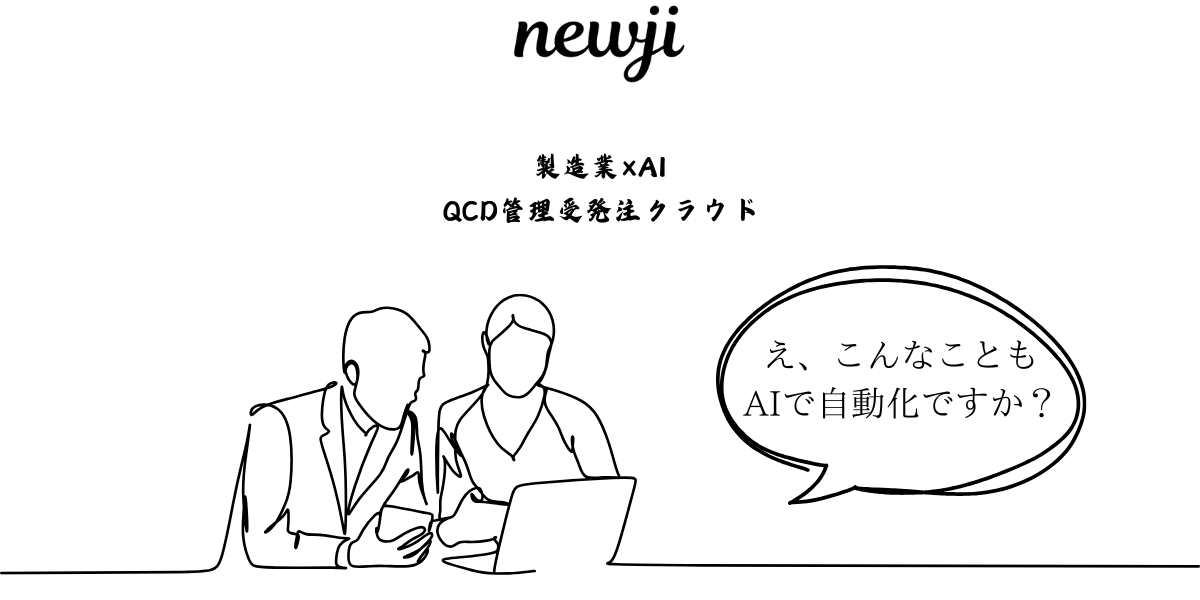
Data utilization techniques to streamline maintenance planning in the manufacturing industry
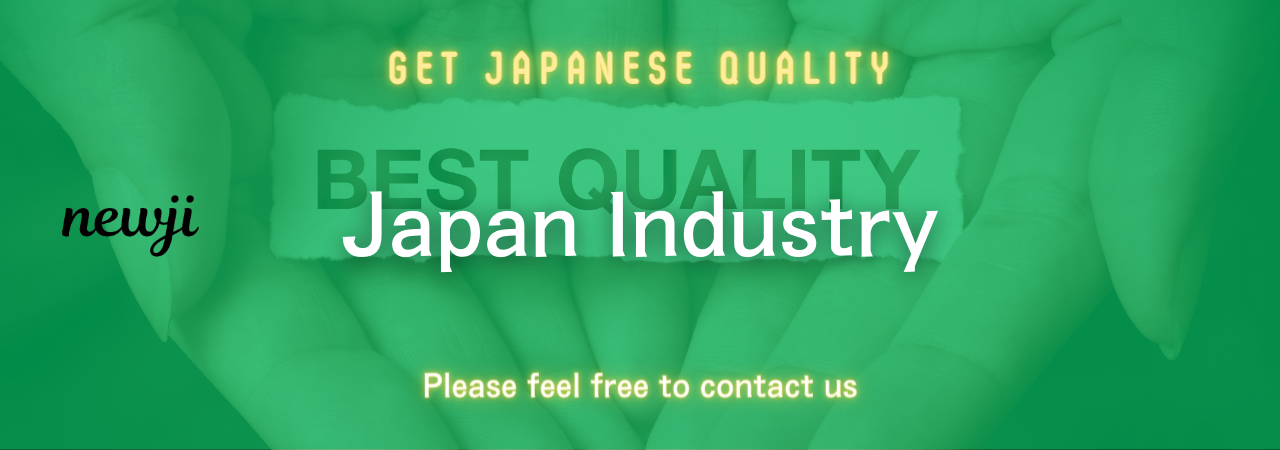
目次
Understanding the Importance of Data in Maintenance Planning
In the manufacturing industry, maintenance planning plays a crucial role in ensuring that all machinery and equipment run smoothly and efficiently.
Unexpected breakdowns can lead to significant downtime, resulting in productivity losses and increased operational costs.
Therefore, utilizing data effectively can transform maintenance planning from a reactive approach to a more proactive one.
By leveraging data, manufacturers can streamline their maintenance strategies, anticipating potential issues before they become serious problems.
The Shift from Reactive to Predictive Maintenance
Traditionally, maintenance in the manufacturing industry was primarily reactive.
This means that companies would address issues and equipment failures as they occurred.
While this approach works to some extent, it often results in unplanned downtime and repairs.
To mitigate these challenges, many manufacturers are now shifting toward predictive maintenance, a strategy heavily reliant on data utilization.
Predictive maintenance uses data analytics and predictive models to monitor equipment health and predict failures.
By analyzing historical data, machine learning algorithms can identify patterns and provide insights into when a machine is likely to require maintenance.
This approach not only reduces unexpected breakdowns but also optimizes maintenance scheduling, which ultimately extends the lifespan of the equipment.
Key Data Utilization Techniques
There are several data utilization techniques that manufacturers can implement to enhance maintenance planning.
These techniques help in gathering, analyzing, and interpreting data to make informed decisions.
1. Implementing IoT Sensors
The Internet of Things (IoT) has revolutionized the way data is collected in the manufacturing industry.
By implementing IoT sensors on machinery, manufacturers can collect real-time data on equipment performance.
These sensors provide continuous monitoring, tracking parameters such as temperature, vibration, and energy consumption.
This data can then be analyzed to identify irregular patterns that may indicate potential failures.
2. Utilizing Big Data Analytics
Big data analytics plays a vital role in processing large volumes of data collected from various sources.
With advanced analytics tools, manufacturers can extract meaningful insights from complex datasets.
This involves using algorithms to discover trends, correlations, and patterns that inform maintenance decisions.
Manufacturers can now predict equipment failures with greater accuracy and plan maintenance activities accordingly.
3. Employing Predictive Modeling
Predictive modeling is a powerful technique used to forecast future events based on historical data.
For maintenance planning, predictive models can predict equipment failure, enabling manufacturers to schedule maintenance activities proactively.
These models use machine learning algorithms to analyze past equipment performance and identify patterns leading to failures.
By implementing predictive models, manufacturers can significantly reduce the chances of unexpected breakdowns.
4. Integrating Machine Learning
Machine learning is a subset of artificial intelligence that enables systems to learn and improve from experience automatically.
In the context of maintenance planning, machine learning algorithms can analyze historical and real-time data to identify warning signs of potential equipment failures.
As these algorithms grow more sophisticated, they can enhance predictive maintenance strategies, further reducing costs and downtime.
Benefits of Streamlined Maintenance Planning
Streamlining maintenance planning through data utilization offers numerous benefits to manufacturers.
These advantages translate into improved efficiency, cost savings, and longer equipment lifespan.
1. Minimized Downtime
By predicting when equipment is likely to fail, manufacturers can schedule maintenance activities at convenient times.
This approach reduces unexpected downtime and ensures that machinery operates efficiently, maximizing productivity.
2. Cost Savings
Proactive maintenance allows manufacturers to address potential issues before they escalate into costly repairs.
This not only saves on repair costs but also prevents losses incurred due to unplanned downtime.
3. Enhanced Equipment Lifespan
Regular maintenance helps in extending the lifespan of machinery.
With predictive maintenance enabled by data, manufacturers can conduct maintenance tasks that effectively preserve equipment health.
4. Improved Safety
Predictive maintenance ensures that equipment is maintained in optimal condition, reducing the risk of accidents caused by malfunctions.
By keeping machinery in good shape, manufacturers foster a safer working environment for their employees.
5. Better Resource Allocation
With a streamlined maintenance strategy, manufacturers can allocate resources more efficiently.
Staff and tools can be assigned based on predicted needs, ensuring that maintenance is carried out without straining resources.
Challenges in Implementing Data Utilization Techniques
While data utilization offers significant advantages, manufacturers may face challenges in implementing these techniques effectively.
1. Data Silos
One major challenge is data silos, where data is stored in isolated systems that don’t communicate with each other.
This hampers the ability to gather comprehensive insights needed for effective maintenance planning.
2. Data Quality
The accuracy of predictive maintenance relies heavily on the quality of data.
Inaccurate or incomplete data can lead to false predictions, causing inefficiencies in maintenance scheduling.
3. Technology Integration
Integrating new technologies, like IoT sensors and machine learning tools, with existing systems can be daunting for manufacturers.
The costs and complexity of integrating these technologies can be prohibitive.
4. Skill Gaps
Implementing advanced data utilization techniques may require specialized skills that manufacturers may lack in their workforce.
Training or hiring staff with the right expertise can be necessary, adding to costs and time.
Conclusion
Data utilization techniques are pivotal in transforming maintenance planning in the manufacturing industry.
By moving from reactive to predictive maintenance, manufacturers can anticipate failures and schedule maintenance strategically.
This transition not only minimizes downtime and reduces costs but also enhances equipment lifespan and workplace safety.
While challenges exist in implementing these techniques, the benefits they offer make them a worthwhile investment for manufacturers aiming to stay competitive in an ever-evolving industry.
資料ダウンロード
QCD調達購買管理クラウド「newji」は、調達購買部門で必要なQCD管理全てを備えた、現場特化型兼クラウド型の今世紀最高の購買管理システムとなります。
ユーザー登録
調達購買業務の効率化だけでなく、システムを導入することで、コスト削減や製品・資材のステータス可視化のほか、属人化していた購買情報の共有化による内部不正防止や統制にも役立ちます。
NEWJI DX
製造業に特化したデジタルトランスフォーメーション(DX)の実現を目指す請負開発型のコンサルティングサービスです。AI、iPaaS、および先端の技術を駆使して、製造プロセスの効率化、業務効率化、チームワーク強化、コスト削減、品質向上を実現します。このサービスは、製造業の課題を深く理解し、それに対する最適なデジタルソリューションを提供することで、企業が持続的な成長とイノベーションを達成できるようサポートします。
オンライン講座
製造業、主に購買・調達部門にお勤めの方々に向けた情報を配信しております。
新任の方やベテランの方、管理職を対象とした幅広いコンテンツをご用意しております。
お問い合わせ
コストダウンが利益に直結する術だと理解していても、なかなか前に進めることができない状況。そんな時は、newjiのコストダウン自動化機能で大きく利益貢献しよう!
(Β版非公開)