- お役立ち記事
- Fundamentals of acoustic signal processing, sound source separation technology using deep learning, and its applications
Fundamentals of acoustic signal processing, sound source separation technology using deep learning, and its applications
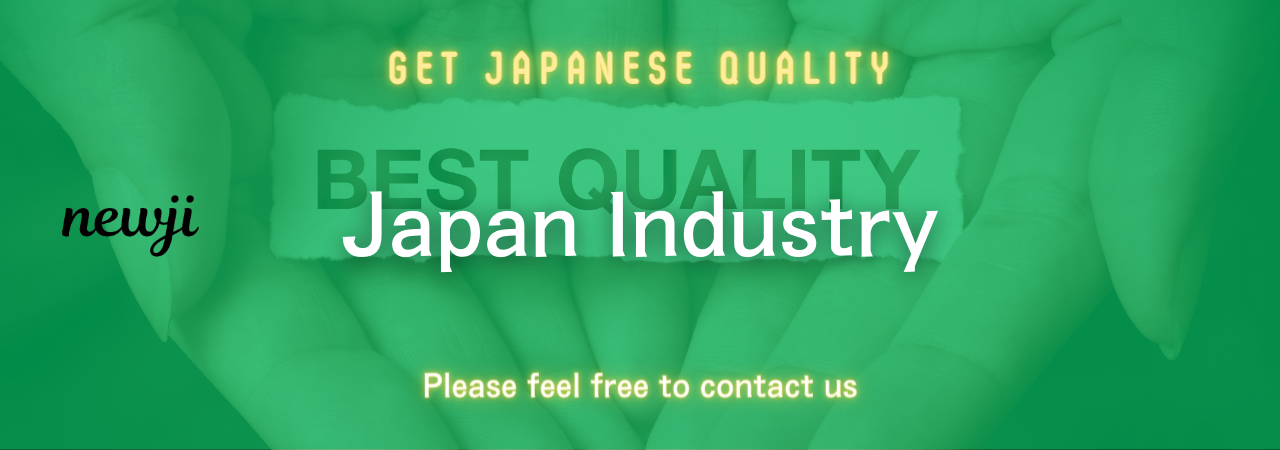
目次
Introduction to Acoustic Signal Processing
Acoustic signal processing is a fundamental aspect of how we interact with sound in various technologies and daily life applications.
It involves the analysis, transformation, and synthesis of audio signals.
These processes allow us to enhance, interpret, and manipulate sound to meet specific requirements.
Acoustic signal processing is used in diverse fields such as telecommunications, audio engineering, and hearing aids.
The goal is to effectively capture, process, and transmit sound waves to improve the clarity and quality of audio signals.
Understanding Sound Source Separation
Sound source separation is a key area within acoustic signal processing.
It refers to the technique of isolating individual sound sources from a mixture of sounds.
Imagine being in a crowded room where multiple conversations are happening simultaneously.
Sound source separation allows us to focus on a single conversation while filtering out other noises.
This is achieved by using various algorithms and technologies to decompose the mixed audio signal into its individual components.
Deep Learning and its Role in Sound Source Separation
Deep learning has revolutionized the field of acoustic signal processing, particularly in sound source separation.
Deep learning algorithms can learn complex patterns and structures within audio data, making them highly effective for separating sound sources.
These algorithms are designed to mimic the human brain, allowing machines to understand and process sound in a sophisticated manner.
By training on large datasets, deep learning models can identify and separate individual audio components with remarkable accuracy.
Methods of Sound Source Separation Using Deep Learning
There are several approaches to employing deep learning for sound source separation.
One common method is using neural networks, specifically convolutional neural networks (CNNs) and recurrent neural networks (RNNs).
Convolutional Neural Networks (CNNs)
CNNs are designed to process data with a grid-like structure, making them ideal for image and audio data.
In sound source separation, CNNs can analyze spectrograms (visual representations of audio signals) and identify distinct sound patterns.
By focusing on specific areas of the spectrogram, CNNs can separate different sound sources accurately.
Recurrent Neural Networks (RNNs)
RNNs are well-suited for sequential data like audio signals, as they can capture temporal dependencies within the data.
They are effective in sound source separation by recognizing the evolving patterns in audio sequences over time.
RNNs excel in handling time series data, making them valuable for real-time applications where sound needs to be continuously processed and separated.
Applications of Deep Learning in Sound Source Separation
The advancements in deep learning and sound source separation hold immense potential across various industries.
Enhancing Communication Systems
In telecommunications, sound source separation improves the quality of calls by isolating speaker voices from background noise.
This technology enables clearer conversations and can be especially beneficial in noisy environments, enhancing the overall user experience.
Advancing Hearing Aids
For individuals with hearing impairments, sound source separation can significantly enhance hearing aids.
By focusing on desired sounds and suppressing background noise, users can experience improved sound clarity, making it easier to understand conversations and enjoy various auditory experiences.
Transforming Audio Production
In the audio production industry, sound source separation enables producers and engineers to manipulate audio tracks more precisely.
It allows them to extract specific instruments or vocals from a music track, offering greater flexibility and creativity in the mixing and mastering process.
Improving Voice Assistants
Voice assistants, such as Siri and Alexa, benefit from sound source separation by accurately interpreting user commands even in noisy environments.
By isolating speech from background noise, these assistants can respond more accurately and efficiently, enhancing their usability and reliability.
Facilitating Forensic Audio Analysis
In forensic investigations, sound source separation can be used to analyze audio recordings and isolate specific voices or sounds of interest.
This technology aids in criminal investigations, providing valuable insights and evidence for law enforcement agencies.
Challenges and Future Prospects
Despite its promising applications, sound source separation using deep learning faces challenges.
One significant challenge is the complexity of real-world audio environments, where multiple sound sources interact dynamically.
Developing models that can handle various noise types, reverberations, and overlapping sounds remains a research focus.
The future of sound source separation is promising, with ongoing advancements in deep learning algorithms and computational power.
Researchers are working to improve the accuracy and efficiency of these models, making them more robust and adaptable to different acoustic scenarios.
As technology continues to evolve, we can expect even more sophisticated applications in areas like virtual reality, autonomous vehicles, and beyond.
Conclusion
The integration of deep learning in acoustic signal processing, particularly in sound source separation, is transforming the way we perceive and interact with sound.
The ability to isolate individual audio components has far-reaching implications across various industries, improving communication, enhancing audio experiences, and opening up new possibilities for innovation.
As research progresses, we can anticipate even greater advancements, leading to a future where sound is processed with unparalleled precision and clarity.
資料ダウンロード
QCD調達購買管理クラウド「newji」は、調達購買部門で必要なQCD管理全てを備えた、現場特化型兼クラウド型の今世紀最高の購買管理システムとなります。
ユーザー登録
調達購買業務の効率化だけでなく、システムを導入することで、コスト削減や製品・資材のステータス可視化のほか、属人化していた購買情報の共有化による内部不正防止や統制にも役立ちます。
NEWJI DX
製造業に特化したデジタルトランスフォーメーション(DX)の実現を目指す請負開発型のコンサルティングサービスです。AI、iPaaS、および先端の技術を駆使して、製造プロセスの効率化、業務効率化、チームワーク強化、コスト削減、品質向上を実現します。このサービスは、製造業の課題を深く理解し、それに対する最適なデジタルソリューションを提供することで、企業が持続的な成長とイノベーションを達成できるようサポートします。
オンライン講座
製造業、主に購買・調達部門にお勤めの方々に向けた情報を配信しております。
新任の方やベテランの方、管理職を対象とした幅広いコンテンツをご用意しております。
お問い合わせ
コストダウンが利益に直結する術だと理解していても、なかなか前に進めることができない状況。そんな時は、newjiのコストダウン自動化機能で大きく利益貢献しよう!
(Β版非公開)