- お役立ち記事
- Fundamentals of data assimilation and key points for building and utilizing digital twins
Fundamentals of data assimilation and key points for building and utilizing digital twins
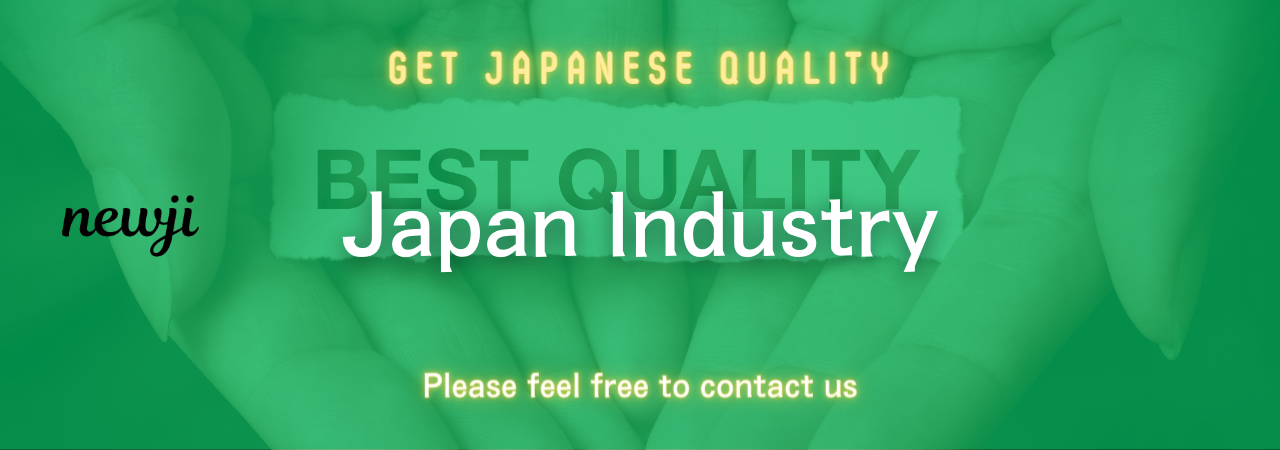
目次
Understanding Data Assimilation
Data assimilation is a critical process in the realm of data science that combines real-world data with model predictions to produce accurate representations of systems.
This methodology is essential for various fields such as meteorology, oceanography, and environmental sciences, where predicting and understanding complex systems is necessary.
In essence, data assimilation helps bridge the gap between observational data and theoretical models to enhance the forecasting and decision-making processes.
At its core, data assimilation involves integrating observations into models at regular intervals to update the state of a system.
By doing so, it enables scientists and analysts to refine their models continuously, yielding more reliable and up-to-date information.
This iterative process is especially valuable in dynamic systems where conditions change rapidly, and timely adjustments are crucial for accuracy.
Key Techniques in Data Assimilation
Several techniques are employed in data assimilation, each with its unique advantages and challenges.
One of the most well-known methods is the Kalman Filter, which is widely used in systems that can be modeled linearly.
This algorithm works by adjusting model predictions based on new measurements, thereby reducing uncertainties over time.
For non-linear models, the Ensemble Kalman Filter (EnKF) offers a practical solution.
This approach uses a series of model state estimates to account for potential variable changes, making it suitable for complex systems with non-linear behaviors.
Its ability to handle large datasets and highly non-linear applications makes EnKF a popular choice in various scientific fields.
Another significant technique is 4D-VAR, or Four-Dimensional Variational Assimilation.
This method optimizes the fit between model forecasts and observations over a given period, taking into account both spatial and temporal dimensions.
By doing so, it addresses discrepancies in model accuracy and helps reconcile differences between observational data and predictive models.
The Rise of Digital Twins
A digital twin is a virtual representation of a physical system, process, or product.
It serves as a digital counterpart that can be used to simulate, analyze, and predict the behaviors and performance of its real-world analog.
In recent years, digital twins have gained prominence across industries such as manufacturing, healthcare, and urban planning, where the capability to test and optimize systems in a virtual environment offers significant advantages.
The concept of digital twins hinges on the integration of multiple technologies, including the Internet of Things (IoT), artificial intelligence (AI), and machine learning.
By leveraging real-time data and advanced analytics, digital twins enable organizations to monitor and manage systems with unprecedented precision.
This approach not only aids in understanding current operations but also supports predictive maintenance and strategic planning.
Applications of Digital Twins
Digital twins are being utilized in a wide range of applications, each leveraging the technology’s unique capabilities.
In manufacturing, digital twins are used to optimize production lines by modeling operations and identifying inefficiencies.
They can predict machine failures before they occur, allowing for proactive maintenance and minimizing downtime.
In healthcare, digital twins provide a virtual model of a patient’s anatomy, assisting in personalized treatment plans and surgical simulations.
This technology empowers healthcare providers to anticipate patient needs and improve clinical outcomes.
Urban planners employ digital twins to design smarter cities by creating dynamic models of urban environments.
These models enable planners to simulate traffic patterns, optimize energy usage, and enhance overall city resilience.
Building and Utilizing Digital Twins
The construction of a digital twin involves several key steps that facilitate its effective implementation and utilization.
The first step is data collection, which requires gathering high-quality and comprehensive data from the physical system or process being modeled.
Sensors and IoT devices play a crucial role here, providing real-time information that drives the digital twin’s accuracy.
Next, the integration of modeling and simulation tools is necessary to create the virtual replica of the physical system.
These tools should account for the complexities of the system and incorporate various data sources for a holistic view.
Once the digital twin is established, continuous monitoring and data assimilation are essential for maintaining its relevance.
This involves updating the digital model with new data to reflect changes and improve predictive capabilities.
Finally, analysis and decision-making are central to utilizing digital twins effectively.
By interpreting the insights derived from the digital model, organizations can make informed decisions that enhance performance and efficiency.
Key Points for Effective Digital Twin Implementation
Successful digital twin implementation requires careful consideration of several factors.
First, defining clear objectives is imperative, as it guides the design and functionality of the digital twin.
This ensures that the virtual model aligns with the organization’s goals and delivers tangible benefits.
Integration of robust data management strategies is also crucial.
Without effective data handling, inconsistencies can arise, compromising the digital twin’s accuracy and reliability.
Collaboration across disciplines enhances the development of digital twins, combining expertise from various fields such as data science, engineering, and business.
Such cooperation fosters innovation and optimizes the digital twin’s potential.
Finally, organizations must commit to continuous evolution, as technology and system requirements change over time.
Adapting to these changes ensures that digital twins remain a valuable asset in the organization’s strategic toolkit.
Conclusion
Data assimilation and digital twins represent pivotal advancements in the pursuit of accurate system modeling and informed decision-making.
By understanding the fundamentals of data assimilation and the strategic application of digital twins, organizations can achieve a competitive edge in their respective fields.
As technology continues to evolve, the importance of these methodologies will only grow, offering unprecedented opportunities to optimize operations and anticipate future challenges.
Through thoughtful implementation and consistent refinement, the fusion of real-world data with digital insights can unlock significant value for businesses and society alike.
資料ダウンロード
QCD調達購買管理クラウド「newji」は、調達購買部門で必要なQCD管理全てを備えた、現場特化型兼クラウド型の今世紀最高の購買管理システムとなります。
ユーザー登録
調達購買業務の効率化だけでなく、システムを導入することで、コスト削減や製品・資材のステータス可視化のほか、属人化していた購買情報の共有化による内部不正防止や統制にも役立ちます。
NEWJI DX
製造業に特化したデジタルトランスフォーメーション(DX)の実現を目指す請負開発型のコンサルティングサービスです。AI、iPaaS、および先端の技術を駆使して、製造プロセスの効率化、業務効率化、チームワーク強化、コスト削減、品質向上を実現します。このサービスは、製造業の課題を深く理解し、それに対する最適なデジタルソリューションを提供することで、企業が持続的な成長とイノベーションを達成できるようサポートします。
オンライン講座
製造業、主に購買・調達部門にお勤めの方々に向けた情報を配信しております。
新任の方やベテランの方、管理職を対象とした幅広いコンテンツをご用意しております。
お問い合わせ
コストダウンが利益に直結する術だと理解していても、なかなか前に進めることができない状況。そんな時は、newjiのコストダウン自動化機能で大きく利益貢献しよう!
(Β版非公開)