- お役立ち記事
- Fundamentals of power grid/system control and application to load prediction and energy management using machine learning
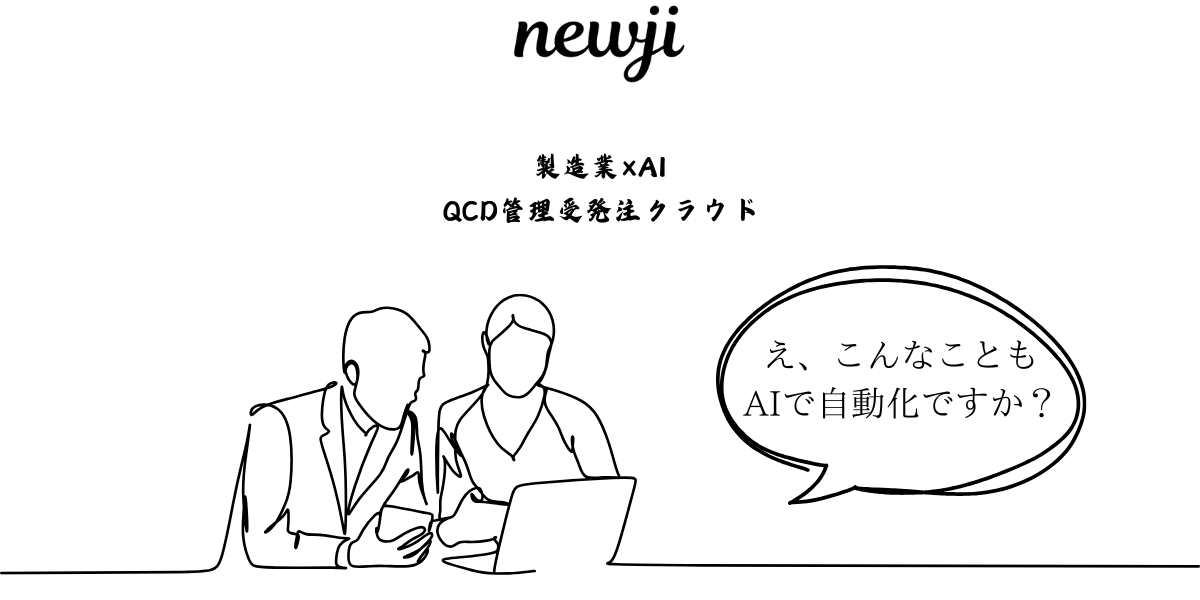
Fundamentals of power grid/system control and application to load prediction and energy management using machine learning
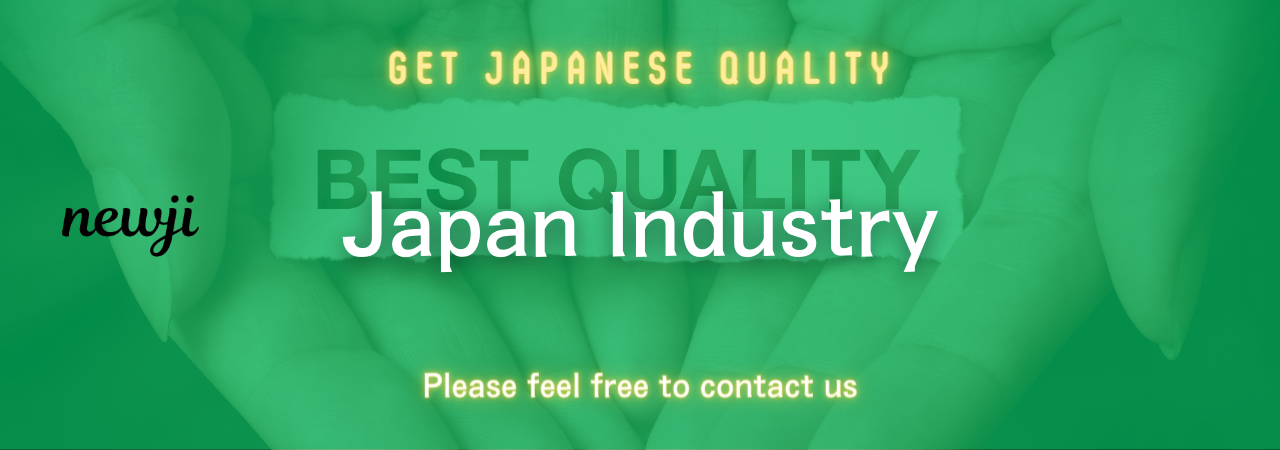
目次
Introduction to Power Grid/System Control
Power grids are complex networks that distribute electricity from power plants to consumers.
To ensure a reliable supply of electricity, power grid systems require meticulous control and management.
This involves balancing the production and consumption of electricity, maintaining voltage levels, and ensuring the stability of the entire system.
System operators use various techniques and technologies to manage these tasks efficiently.
The Role of Control in Power Grids
Control systems in power grids are essential for managing the flow of electricity.
They help to regulate the generation and consumption of power, thereby maintaining the balance between supply and demand.
This is achieved through the use of advanced technologies such as sensors, automated switching devices, and communication systems.
These tools enable operators to monitor the grid in real-time and respond to any disruptions or inefficiencies.
Understanding Load Prediction
One crucial aspect of power grid management is load prediction.
Load prediction involves forecasting the amount of electricity that consumers will require in the future.
Accurate load predictions are essential for planning and managing the production and distribution of electricity.
Without reliable forecasts, power grids can either overproduce or underproduce electricity, leading to wasted resources or power shortages.
Traditional Methods of Load Prediction
Traditionally, load prediction has relied on statistical techniques and historical data analysis.
These methods involve examining past consumption patterns and using that information to predict future demand.
While these approaches can be effective, they are relatively limited in their capability to adapt to changes in consumer behavior or unexpected events.
Machine Learning in Power Systems
In recent years, machine learning (ML) has emerged as a powerful tool for enhancing power grid management and load prediction.
With its ability to learn from large datasets and identify complex patterns, machine learning can significantly improve the accuracy of predictions and control mechanisms.
How Machine Learning Works
Machine learning algorithms analyze vast amounts of data to identify trends and relationships that can inform decision-making processes.
In the context of power grids, this means examining data from sensors, historical consumption records, and other relevant sources.
By recognizing patterns within this data, machine learning models can make more accurate predictions about future load requirements.
Application of Machine Learning in Load Prediction
Machine learning can revolutionize load prediction by incorporating various data points, such as weather conditions, economic factors, and social trends, into its models.
This allows for a more comprehensive understanding of factors affecting energy demand.
Benefits of Machine Learning in Load Prediction
1. **Increased Accuracy:** Machine learning models can improve the accuracy of load predictions by considering multiple variables and recognizing patterns that traditional methods might miss.
2. **Adaptability:** These models can quickly adapt to changes in data and remain relevant in varying circumstances, such as seasonal variations or unexpected events.
3. **Efficiency:** By providing more accurate forecasts, machine learning helps operators optimize power generation and distribution, reducing waste and enhancing energy efficiency.
Energy Management Using Machine Learning
Beyond load prediction, machine learning can optimize energy management strategies.
Energy management refers to the monitoring, controlling, and conserving of energy in a power grid.
Use Cases in Energy Management
1. **Demand Response:** Machine learning can improve demand response strategies by predicting times of peak demand and recommending the best actions to balance the load.
2. **Energy Efficiency:** By analyzing consumption data, machine learning can identify inefficiencies and suggest improvements, such as upgrading equipment or adjusting usage patterns.
3. **Integration of Renewable Energy:** Machine learning can help integrate renewable energy sources into power grids by predicting their output and ensuring a balanced power supply.
Challenges and Considerations
While machine learning offers significant benefits for power grid management, it also presents certain challenges.
Data Quality and Availability
The effectiveness of machine learning models largely depends on the quality and availability of data.
Without accurate and comprehensive data, predictions and decisions may be flawed.
Technical Expertise
Developing and maintaining machine learning models require significant technical expertise.
Operators must have the skills to build, test, and refine these models to ensure they function effectively.
Infrastructure and Investment
Implementing machine learning systems may require substantial infrastructure and financial investment.
Organizations must weigh the potential benefits against the costs of adopting these technologies.
Conclusion
The integration of machine learning into power grid management represents a significant leap forward in ensuring a reliable and efficient energy supply.
By improving load prediction and enhancing energy management techniques, machine learning can address some of the key challenges faced by traditional methods.
While there are obstacles to overcome, the potential benefits of machine learning in this field are enormous, promising a future where power grids are more adaptive, efficient, and resilient.
資料ダウンロード
QCD調達購買管理クラウド「newji」は、調達購買部門で必要なQCD管理全てを備えた、現場特化型兼クラウド型の今世紀最高の購買管理システムとなります。
ユーザー登録
調達購買業務の効率化だけでなく、システムを導入することで、コスト削減や製品・資材のステータス可視化のほか、属人化していた購買情報の共有化による内部不正防止や統制にも役立ちます。
NEWJI DX
製造業に特化したデジタルトランスフォーメーション(DX)の実現を目指す請負開発型のコンサルティングサービスです。AI、iPaaS、および先端の技術を駆使して、製造プロセスの効率化、業務効率化、チームワーク強化、コスト削減、品質向上を実現します。このサービスは、製造業の課題を深く理解し、それに対する最適なデジタルソリューションを提供することで、企業が持続的な成長とイノベーションを達成できるようサポートします。
オンライン講座
製造業、主に購買・調達部門にお勤めの方々に向けた情報を配信しております。
新任の方やベテランの方、管理職を対象とした幅広いコンテンツをご用意しております。
お問い合わせ
コストダウンが利益に直結する術だと理解していても、なかなか前に進めることができない状況。そんな時は、newjiのコストダウン自動化機能で大きく利益貢献しよう!
(Β版非公開)