- お役立ち記事
- Fundamentals of fatigue damage in metal materials and application to fatigue life and strength prediction using AI (machine learning)
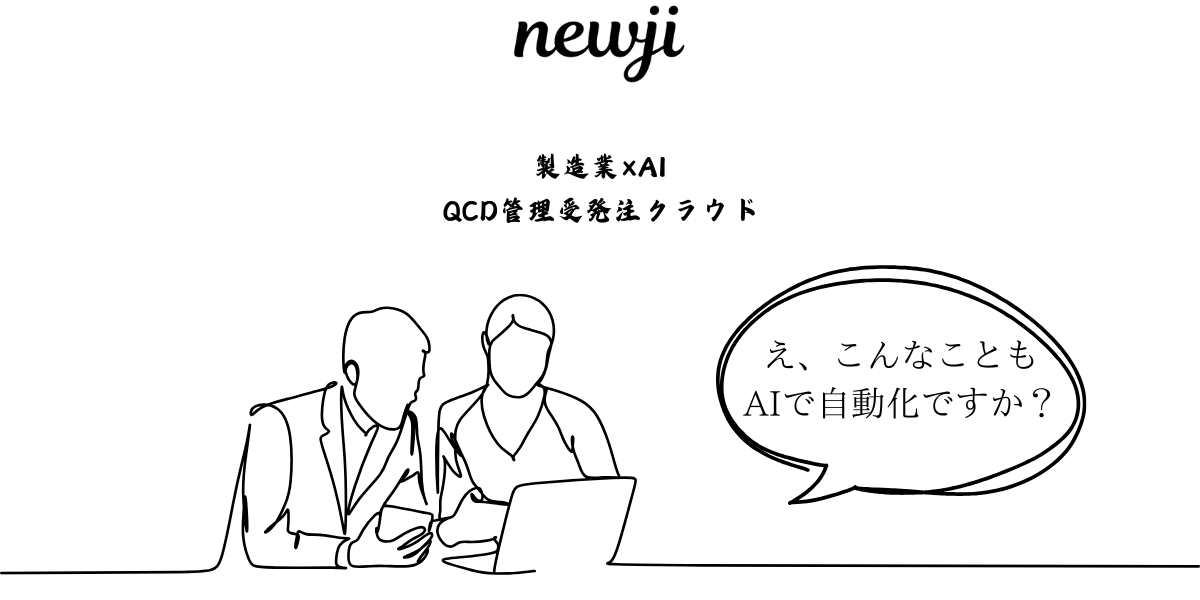
Fundamentals of fatigue damage in metal materials and application to fatigue life and strength prediction using AI (machine learning)
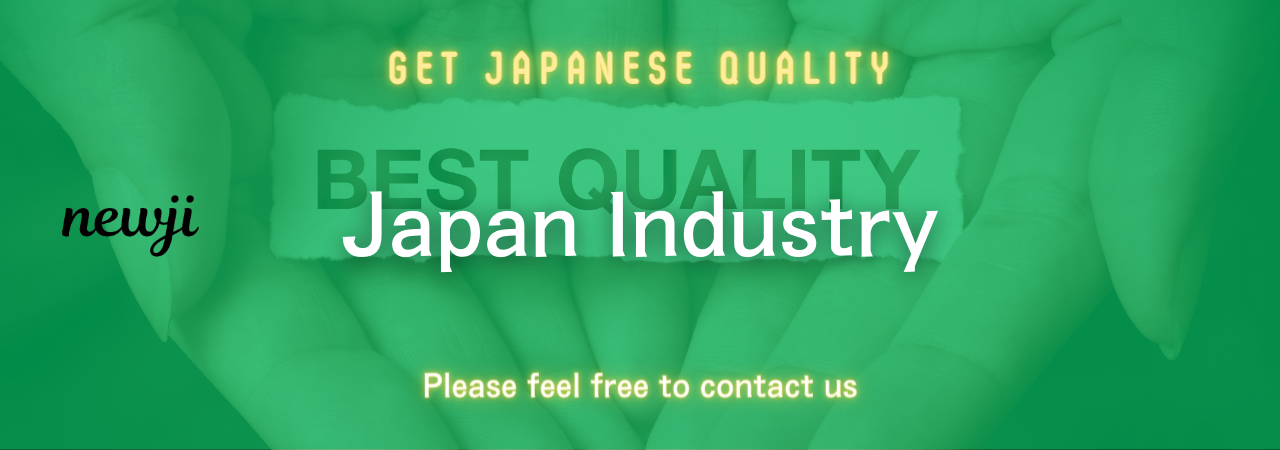
目次
Understanding Fatigue Damage in Metal Materials
Fatigue damage is a common issue faced by metal materials when subjected to repetitive stress or loading over time.
This phenomenon leads to the gradual progression of structural damage, ultimately resulting in failure of the material without significant plastic deformation.
Understanding the fundamentals of fatigue damage is crucial for designing durable components and structures, particularly in industries such as aerospace, automotive, and construction.
Metal fatigue occurs because of the propagation of microscopic cracks within the material.
These cracks start at stress concentration points such as surface defects, inclusions, or other forms of localized weakness.
As the material experiences cyclic loading, these cracks grow, eventually leading to a complete fracture.
It is essential to predict when and how this will occur to ensure safety and reliability in metal structures.
Factors Influencing Metal Fatigue
Several factors influence the fatigue life of metal materials.
One of the primary factors is the magnitude and frequency of the applied stress.
Higher stress levels can significantly reduce the fatigue life, while lower stress levels may allow the material to last longer.
Additionally, the environment plays a vital role.
For instance, corrosive environments can expedite the fatigue process by promoting crack initiation and propagation.
The surface condition of the metal is another crucial factor.
Rough surfaces with scratches or notches act as stress concentrators that accelerate fatigue damage.
On the other hand, polished or well-finished surfaces often exhibit better fatigue performance due to reduced stress concentration sites.
Furthermore, the material’s inherent properties, such as its microstructure, composition, and heat treatment, can also affect its fatigue behavior.
Materials with more homogeneous microstructures tend to have better resistance to fatigue damage compared to those with heterogeneous structures.
Predicting Fatigue Life and Strength Using Traditional Methods
Traditionally, predicting fatigue life and strength in metal materials has been achieved through empirical methods and mathematical models.
The most commonly used method is the S-N curve (also known as the Wöhler curve), which represents the relationship between the cyclic stress amplitude (S) and the number of cycles to failure (N).
This curve is derived from extensive experimental data and helps engineers predict the lifespan of a material under specific loading conditions.
Another traditional approach is the Paris Law, which models the rate of fatigue crack growth based on stress intensity factors.
By understanding how quickly cracks will propagate under varying loads, engineers can predict the remaining life of a material before failure occurs.
However, these traditional methods have limitations.
They often require extensive experimental data, which can be time-consuming and costly to obtain.
Moreover, they may not always accurately predict fatigue life under complex loading scenarios or for new material alloys.
Leveraging AI and Machine Learning for Accurate Fatigue Predictions
The advent of artificial intelligence (AI) and machine learning has opened new avenues for predicting fatigue life and strength in metal materials more accurately and efficiently.
These technologies allow for the analysis of large datasets, enabling the creation of advanced predictive models that can account for complex factors and interactions affecting fatigue behavior.
Machine learning algorithms, such as neural networks and support vector machines, can learn patterns from historical data and predict future occurrences of fatigue-related failures.
By training these models on existing datasets, they can make predictions with relatively high accuracy and reduce the reliance on extensive experimental testing.
AI can also be used to optimize material design and manufacturing processes by identifying key parameters that influence fatigue resistance.
This way, engineers can improve the performance of metal components by selecting appropriate materials and treatment methods.
Benefits of AI in Predicting Fatigue Life
One of the most significant benefits of using AI in fatigue prediction is its ability to handle vast amounts of data efficiently.
AI systems can analyze input variables, such as stress levels, environmental conditions, and material properties, simultaneously, providing enhanced insight into the factors contributing to fatigue damage.
Moreover, AI-based models are adaptive, meaning they can be continuously updated as new data becomes available.
This adaptability ensures that predictions remain accurate and relevant as material properties or operating conditions change over time.
AI also offers considerable time and cost savings.
With machine learning models, engineers can reduce the need for extensive empirical testing, thus accelerating the design and certification process for new components and structures.
Conclusion
Understanding and predicting fatigue damage in metal materials are crucial tasks for ensuring the safety, reliability, and durability of engineering structures.
Traditional methods, while effective, have their limitations, particularly when dealing with complex variables and scenarios.
AI and machine learning present a promising solution, offering enhanced predictive capabilities and greater efficiency.
These advanced technologies enable engineers to make more informed decisions, optimize material performance, and anticipate potential failures before they occur.
As industries continue to adopt AI for fatigue prediction, the future of metal materials looks increasingly secure and resilient.
資料ダウンロード
QCD調達購買管理クラウド「newji」は、調達購買部門で必要なQCD管理全てを備えた、現場特化型兼クラウド型の今世紀最高の購買管理システムとなります。
ユーザー登録
調達購買業務の効率化だけでなく、システムを導入することで、コスト削減や製品・資材のステータス可視化のほか、属人化していた購買情報の共有化による内部不正防止や統制にも役立ちます。
NEWJI DX
製造業に特化したデジタルトランスフォーメーション(DX)の実現を目指す請負開発型のコンサルティングサービスです。AI、iPaaS、および先端の技術を駆使して、製造プロセスの効率化、業務効率化、チームワーク強化、コスト削減、品質向上を実現します。このサービスは、製造業の課題を深く理解し、それに対する最適なデジタルソリューションを提供することで、企業が持続的な成長とイノベーションを達成できるようサポートします。
オンライン講座
製造業、主に購買・調達部門にお勤めの方々に向けた情報を配信しております。
新任の方やベテランの方、管理職を対象とした幅広いコンテンツをご用意しております。
お問い合わせ
コストダウンが利益に直結する術だと理解していても、なかなか前に進めることができない状況。そんな時は、newjiのコストダウン自動化機能で大きく利益貢献しよう!
(Β版非公開)