- お役立ち記事
- Fundamentals of Bayesian inference and applications to signal processing and machine learning
Fundamentals of Bayesian inference and applications to signal processing and machine learning
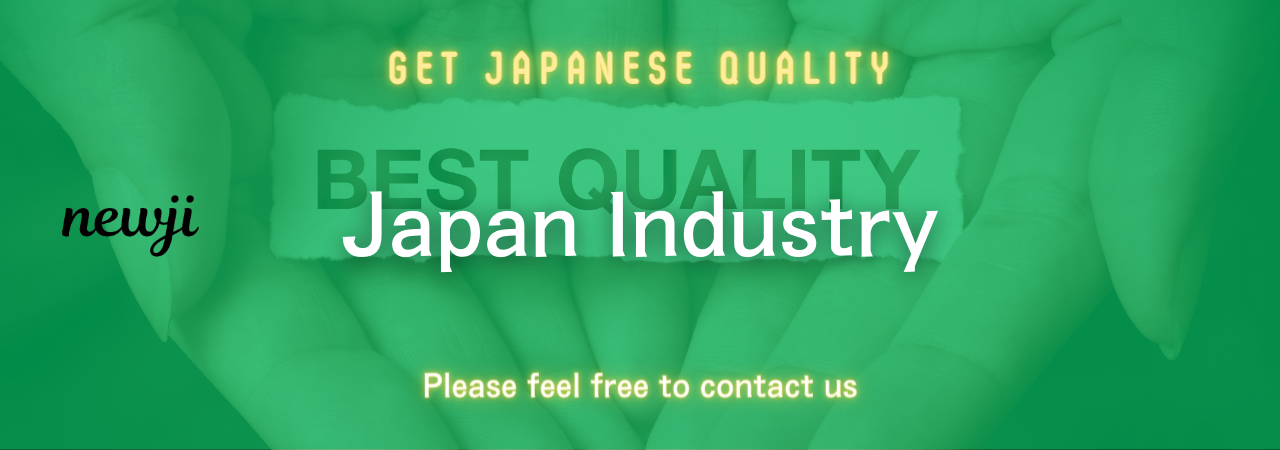
目次
Understanding Bayesian Inference
Bayesian inference is a method of statistical inference that uses probabilities to make predictions and draw conclusions from data.
It is based on Bayes’ Theorem, which provides a way to update the probability of a hypothesis being true, given new evidence or data.
This approach contrasts with traditional frequentist statistics, which often relies on point estimates and hypothesis testing.
At its core, Bayesian inference combines prior knowledge about a problem with new data to update our beliefs or predictions.
This makes it particularly powerful in situations where data is limited or uncertain.
By incorporating prior knowledge, Bayesian inference can improve the accuracy and robustness of predictions.
One crucial aspect of Bayesian inference is the concept of prior and posterior distributions.
The prior distribution represents our initial beliefs before observing any data.
Once we collect and analyze data, we update these beliefs, resulting in a posterior distribution.
The posterior distribution reflects our revised beliefs after taking into account the new evidence.
The Role of Bayes’ Theorem
Bayes’ Theorem is the mathematical foundation of Bayesian inference.
It provides a formal method for updating probabilities based on new information.
The theorem can be expressed as:
P(H|D) = [P(D|H) * P(H)] / P(D)
In this equation:
– P(H|D) is the posterior probability, the probability of the hypothesis H given the observed data D.
– P(D|H) is the likelihood, the probability of observing the data D given that the hypothesis H is true.
– P(H) is the prior probability, representing our initial beliefs about the hypothesis.
– P(D) is the marginal likelihood, a normalization constant that ensures the probabilities sum to one.
Bayesian inference uses Bayes’ Theorem to update the prior distribution into a posterior distribution.
By considering both the likelihood of the data and the prior beliefs, Bayesian inference provides a balanced and flexible framework for statistical analysis.
Applications in Signal Processing
Signal processing is an area where Bayesian inference plays a significant role.
In this context, signals refer to any time-varying or spatially varying data that represent information or physical phenomena.
Bayesian methods provide a systematic way to process and analyze these signals, especially when dealing with noise or incomplete data.
One common application of Bayesian inference in signal processing is noise reduction.
In many real-world scenarios, signals are contaminated with noise, making it challenging to extract meaningful information.
Bayesian methods can model the noise characteristics and use prior information to filter out the noise while preserving the underlying signal.
This leads to improved signal quality and accuracy.
Another application is in feature extraction and pattern recognition.
Bayesian inference can be used to identify and extract important features from signals, such as speech or image data.
By leveraging prior knowledge and probabilistic models, Bayesian methods can enhance the identification of relevant patterns and improve classification performance.
Bayesian Estimation Techniques
Several Bayesian estimation techniques are commonly used in signal processing, including:
– Maximum A Posteriori (MAP) Estimation: This technique estimates the most probable value of a parameter by maximizing the posterior distribution.
MAP estimation strikes a balance between fitting the data and adhering to prior beliefs.
– Bayesian Filtering: This approach is used for estimating the state of a dynamic system over time.
Kalman filters and particle filters are popular Bayesian filtering methods that account for uncertainties in the data and model parameters.
– Bayesian Wavelet Shrinkage: This technique is used for denoising signals using wavelet transforms.
Bayesian wavelet shrinkage combines wavelet coefficients with prior distributions to achieve optimal noise reduction while maintaining signal integrity.
Applications in Machine Learning
Machine learning is another domain where Bayesian inference has found extensive applications.
Bayesian methods provide a natural framework for modeling uncertainty and managing complex data structures.
They offer advantages in various machine learning tasks, such as classification, regression, clustering, and model selection.
In classification, Bayesian approaches can estimate the probability of each class given the observed data and provide a measure of uncertainty in the predictions.
This is particularly useful in applications where decision-making under uncertainty is critical.
In regression tasks, Bayesian methods can capture the uncertainty in parameter estimates and model predictions.
This helps in making more informed decisions and understanding the reliability of the results.
Hierarchical Models and Bayesian Networks
Hierarchical models and Bayesian networks are powerful tools within the Bayesian framework.
Hierarchical models, also known as multi-level models, allow for the modeling of complex data structures with multiple levels of variability.
They are useful in scenarios with nested data, such as modeling group effects or spatial-temporal data patterns.
Bayesian networks, on the other hand, represent probabilistic relationships between variables using directed acyclic graphs.
These networks model the dependencies between variables and provide a graphical representation of the joint probability distribution.
Bayesian networks are widely used in machine learning for tasks such as causal inference, decision making, and belief updating.
Conclusion
Bayesian inference is a fundamental concept in statistics with widespread applications in signal processing and machine learning.
Its ability to incorporate prior knowledge and update beliefs based on new data makes it a powerful tool for handling uncertainty and complexity.
In signal processing, Bayesian methods enable noise reduction, feature extraction, and pattern recognition.
In machine learning, they enhance classification, regression, and model selection by providing probabilistic frameworks for modeling uncertainty.
By understanding and applying Bayesian inference, practitioners can improve the accuracy and reliability of their models, making better-informed decisions in various domains.
As the availability of data continues to grow, the significance of Bayesian methods in addressing real-world challenges is set to increase, making them an indispensable part of modern analytics.
資料ダウンロード
QCD調達購買管理クラウド「newji」は、調達購買部門で必要なQCD管理全てを備えた、現場特化型兼クラウド型の今世紀最高の購買管理システムとなります。
ユーザー登録
調達購買業務の効率化だけでなく、システムを導入することで、コスト削減や製品・資材のステータス可視化のほか、属人化していた購買情報の共有化による内部不正防止や統制にも役立ちます。
NEWJI DX
製造業に特化したデジタルトランスフォーメーション(DX)の実現を目指す請負開発型のコンサルティングサービスです。AI、iPaaS、および先端の技術を駆使して、製造プロセスの効率化、業務効率化、チームワーク強化、コスト削減、品質向上を実現します。このサービスは、製造業の課題を深く理解し、それに対する最適なデジタルソリューションを提供することで、企業が持続的な成長とイノベーションを達成できるようサポートします。
オンライン講座
製造業、主に購買・調達部門にお勤めの方々に向けた情報を配信しております。
新任の方やベテランの方、管理職を対象とした幅広いコンテンツをご用意しております。
お問い合わせ
コストダウンが利益に直結する術だと理解していても、なかなか前に進めることができない状況。そんな時は、newjiのコストダウン自動化機能で大きく利益貢献しよう!
(Β版非公開)