- お役立ち記事
- Quality and safety assurance technology and verification methods for AI-equipped systems and their important points
Quality and safety assurance technology and verification methods for AI-equipped systems and their important points
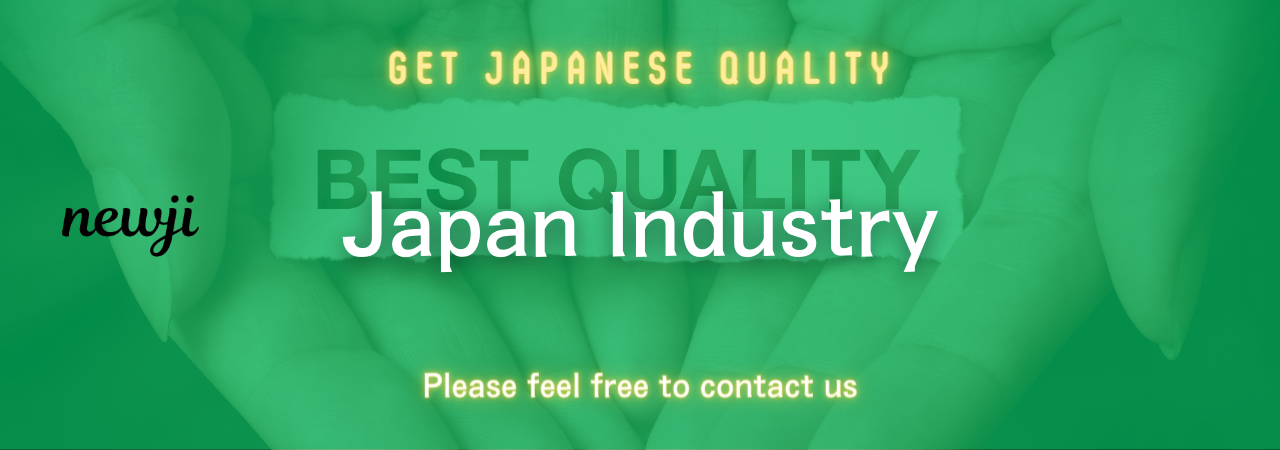
目次
Introduction to AI-Equipped Systems
AI-equipped systems are increasingly becoming a staple of modern technology, transforming industries like healthcare, automotive, finance, and more.
These systems are renowned for their ability to process vast amounts of data, adapt to new information, and perform tasks with a level of intelligence that mimics human reasoning.
However, with the advancement of these technologies comes the crucial need for quality and safety assurance.
Ensuring that these systems operate safely and effectively is paramount, which is why understanding the verification methods is crucial.
The Importance of Quality and Safety Assurance
The deployment of AI systems without proper oversight can lead to significant risks, ranging from malfunctioning algorithms to unintended behavior.
These issues can harm users, damage trust in AI technologies, and, in severe cases, lead to catastrophic outcomes.
Therefore, the importance of quality and safety assurance cannot be overstated.
It provides a safety net, ensuring that systems perform as intended, minimizing risks to humans and infrastructure.
Quality assurance (QA) in AI involves continuous monitoring, testing, and refinement of these systems throughout their lifecycle.
Safety assurance, meanwhile, applies rigorous standards and protocols to safeguard against potential hazards.
Verification Methods for AI-Equipped Systems
Verification is a critical aspect of ensuring that AI systems meet their design specifications and functional requirements.
Several methods have been developed to verify AI systems effectively.
Formal Verification
Formal verification involves mathematically proving the correctness of algorithms within AI systems.
By employing logic and mathematical models, engineers can ensure that the systems function according to their specifications.
This method is particularly useful in high-stakes environments like aerospace and finance, where errors can lead to serious consequences.
Simulation and Testing
Simulation and testing involve creating virtual environments where AI systems can be evaluated under controlled conditions.
Engineers can simulate different scenarios to see how the AI reacts, ensuring that it behaves safely and predictably.
Testing can be conducted for both pre-release and post-deployment systems, offering a comprehensive understanding of the system’s capabilities and limitations.
Machine Learning Model Audits
These audits involve a thorough examination of the machine learning models to ensure they operate accurately and without bias.
Audits help identify potential flaws or biases, thereby improving model accuracy and fairness.
This method also ensures that decisions made by AI are transparent and justifiable.
Continuous Monitoring and Feedback Loops
Establishing continuous monitoring and feedback loops allows for real-time quality and safety assessment of AI systems.
By constantly reviewing performance data and user feedback, developers can promptly address issues, make improvements, and ensure ongoing compliance with safety standards.
Important Points in Ensuring Quality and Safety
Ensuring the quality and safety of AI-equipped systems involves several critical considerations.
Robustness and Reliability
AI systems must be robust and reliable, with the ability to function under diverse conditions and withstand unexpected inputs.
To achieve robustness, systems need extensive testing and validation against various real-world scenarios.
Transparency and Accountability
Developers must build AI systems with transparency, making the operations and decision-making processes understandable to users.
Accountability involves holding developers responsible for the actions and outputs of AI systems.
Implementing robust documentation and version control systems can help maintain transparency and accountability.
Bias and Fairness
AI systems must be scrutinized for biases that can lead to unfair outcomes.
Developers need to ensure that data sets used for training AI are diverse and representative of different demographics.
Fairness can be ensured through regular audits and updates to the AI models.
Security Measures
Security is a significant aspect of AI safety assurance.
This involves implementing measures to protect AI systems from cyber threats and unauthorized access.
Encryption, regular security updates, and strict access controls are essential components of a robust security strategy.
Ethical Considerations
Ethical considerations play a substantial role in the development of AI systems.
Developers need to address ethical questions about how AI technologies are used, ensuring they align with societal values and do not cause harm.
Conclusion
AI-equipped systems promise tremendous potential across various sectors, but their successful integration hinges on robust quality and safety assurance measures.
By adopting reliable verification methods and focusing on critical considerations such as robustness, transparency, bias, security, and ethics, developers can build safe and effective AI systems.
As technology evolves, continuous improvement and adaptation of these practices are essential to maintaining trust and ensuring the safety of AI technologies in our daily lives.
資料ダウンロード
QCD調達購買管理クラウド「newji」は、調達購買部門で必要なQCD管理全てを備えた、現場特化型兼クラウド型の今世紀最高の購買管理システムとなります。
ユーザー登録
調達購買業務の効率化だけでなく、システムを導入することで、コスト削減や製品・資材のステータス可視化のほか、属人化していた購買情報の共有化による内部不正防止や統制にも役立ちます。
NEWJI DX
製造業に特化したデジタルトランスフォーメーション(DX)の実現を目指す請負開発型のコンサルティングサービスです。AI、iPaaS、および先端の技術を駆使して、製造プロセスの効率化、業務効率化、チームワーク強化、コスト削減、品質向上を実現します。このサービスは、製造業の課題を深く理解し、それに対する最適なデジタルソリューションを提供することで、企業が持続的な成長とイノベーションを達成できるようサポートします。
オンライン講座
製造業、主に購買・調達部門にお勤めの方々に向けた情報を配信しております。
新任の方やベテランの方、管理職を対象とした幅広いコンテンツをご用意しております。
お問い合わせ
コストダウンが利益に直結する術だと理解していても、なかなか前に進めることができない状況。そんな時は、newjiのコストダウン自動化機能で大きく利益貢献しよう!
(Β版非公開)