- お役立ち記事
- Fundamentals of generative AI and large-scale language models and points for system development and implementation using generative AI
Fundamentals of generative AI and large-scale language models and points for system development and implementation using generative AI
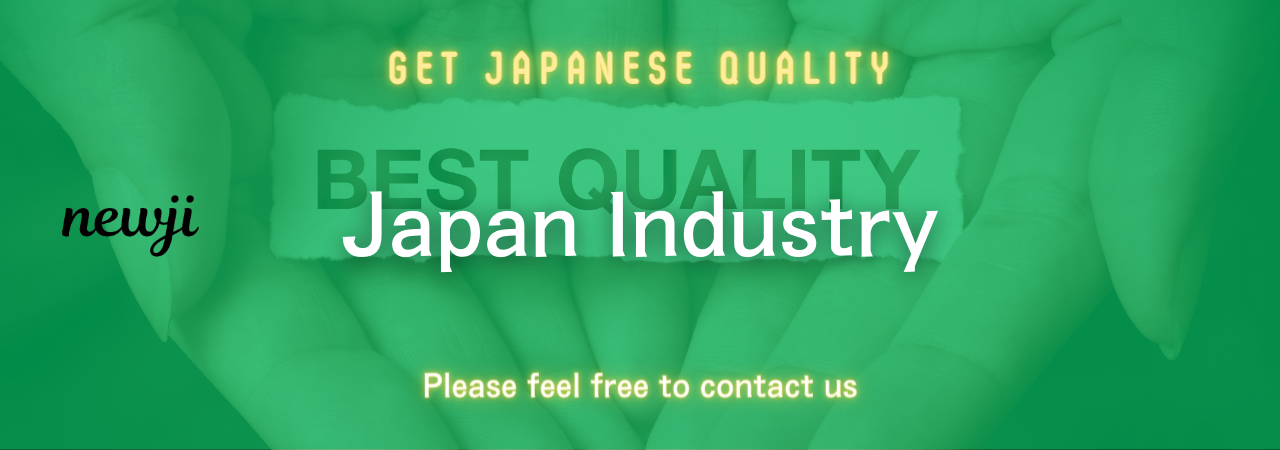
目次
Understanding Generative AI
Generative AI is a subfield of artificial intelligence focused on creating systems that can generate human-like text, images, music, and more.
Derived from vast datasets, these AI models learn patterns and structures within the data to create new content.
Unlike traditional AI, which follows predefined instructions, generative AI models have the ability to handle a much broader spectrum of tasks due to their open-ended nature.
At the core of generative AI is its ability to produce content that seems thoughtfully crafted by humans.
This capability comes from training models on large datasets, allowing them to learn intricate details of human language and visual aesthetics.
The most remarkable aspect of generative AI is its versatility, which can be applied to numerous industries, from creative fields like art and content generation to practical applications like coding and data analysis.
The Role of Large-Scale Language Models
Large-scale language models are a foundational component of generative AI.
These models, such as OpenAI’s GPT (Generative Pre-trained Transformer), are trained on extensive text data, enabling them to understand context, grammar, and even nuances of human language.
They improve upon their predecessors by incorporating transformer architectures, which handle dependencies between words more efficiently.
The remarkable prowess of large-scale language models lies in their ability to generate coherent and contextually relevant text.
They achieve this by predicting the next word in a sentence, considering all the preceding words.
Such capabilities allow them to engage in meaningful conversations, write essays, compose articles, and even assist in creative writing endeavors.
Moreover, their understanding of language goes beyond mere word prediction.
They can perform tasks like summarization, translation, and sentiment analysis, making them invaluable in fields that require language comprehension and generation.
Benefits of Implementing Generative AI
The implementation of generative AI offers multiple advantages, significantly transforming how businesses and industries operate.
One primary benefit is enhanced productivity.
Generative AI can automate repetitive tasks, streamline workflows, and generate content that would otherwise require considerable human input, thereby freeing up time for more strategic endeavors.
Additionally, generative AI can improve customer experiences by providing personalized content and services.
For instance, in the retail industry, generative models can create personalized marketing materials or even design custom products based on individual preferences.
In the creative realm, generative AI acts as a catalyst for innovation.
It assists artists and designers by offering new perspectives and ideas, thereby expanding the boundaries of human creativity.
This symbiotic relationship allows human creators to push the envelope while leveraging AI’s computational power and diversity in thinking.
Challenges in Developing Generative AI Systems
While the potential of generative AI is vast, there are several challenges in system development and implementation.
One prominent challenge is the requirement for immense computational resources for training these large-scale models.
The training process entails processing enormous datasets, a task that demands significant energy and hardware investments.
Another concern is the quality and bias in the training data.
Models trained on biased or low-quality data can produce undesirable or skewed outputs.
Ensuring data diversity and fairness is crucial for developing reliable generative AI systems that provide equitable outcomes.
Moreover, the interpretability of these models poses a concern.
Understanding why a model produced specific results can be challenging due to the complex nature of neural networks.
This lack of transparency can lead to mistrust and unpredicted behaviors in critical applications.
Strategies for Successful System Implementation
To successfully implement generative AI systems, a strategic approach is essential.
Firstly, defining clear goals is crucial.
Understanding what the generative AI system needs to achieve guides the development process and aligns it with organizational objectives.
This clarity ensures that the resources invested in generative AI contribute effectively to business goals.
Secondly, data quality must be prioritized.
Thoroughly vetting datasets for accuracy, representation, and unbiased content is vital to developing trustworthy models.
This aspect often involves implementing robust data governance frameworks and ongoing monitoring to ensure sustained data integrity.
Thirdly, the integration of ethical guidelines is necessary to navigate the ethical implications of generative AI.
Developers must establish and adhere to principles that promote fairness, transparency, and accountability.
Engaging with stakeholders, including users and ethicists, helps inform these guidelines, ensuring that the system aligns with societal and ethical standards.
Lastly, continuous evaluation and iteration are key tenets for system refinement.
Feedback loops and performance assessments allow developers to fine-tune the models, addressing potential pitfalls and enhancing their capabilities over time.
Regular updates ensure that the generative AI system remains relevant and effective in fluctuating environments.
Future Prospects of Generative AI
The future of generative AI is both exciting and challenging.
As technological advancements continue, we can anticipate even more sophisticated models with heightened capabilities.
These models will likely offer enhanced creativity, increased adaptability, and new applications we have yet to envision.
There is significant potential in fields such as healthcare, where generative AI can assist in drug discovery and personalized medicine.
In education, these models could personalize learning experiences, adapting pace and content to individual learning styles.
Nevertheless, with these advancements come increased responsibility regarding regulation, ethical use, and the societal impact of AI.
Ongoing dialogue between technologists, policymakers, and society at large will be crucial in shaping a future where generative AI is harnessed for the collective good.
The path forward lies in fostering a collaborative environment where diversity of thought and cross-disciplinary expertise drive innovation in a responsible and equitable manner.
By keeping an eye on developments and prioritizing ethical considerations, generative AI can unlock unprecedented possibilities, revolutionizing industries while upholding values that benefit society as a whole.
資料ダウンロード
QCD調達購買管理クラウド「newji」は、調達購買部門で必要なQCD管理全てを備えた、現場特化型兼クラウド型の今世紀最高の購買管理システムとなります。
ユーザー登録
調達購買業務の効率化だけでなく、システムを導入することで、コスト削減や製品・資材のステータス可視化のほか、属人化していた購買情報の共有化による内部不正防止や統制にも役立ちます。
NEWJI DX
製造業に特化したデジタルトランスフォーメーション(DX)の実現を目指す請負開発型のコンサルティングサービスです。AI、iPaaS、および先端の技術を駆使して、製造プロセスの効率化、業務効率化、チームワーク強化、コスト削減、品質向上を実現します。このサービスは、製造業の課題を深く理解し、それに対する最適なデジタルソリューションを提供することで、企業が持続的な成長とイノベーションを達成できるようサポートします。
オンライン講座
製造業、主に購買・調達部門にお勤めの方々に向けた情報を配信しております。
新任の方やベテランの方、管理職を対象とした幅広いコンテンツをご用意しております。
お問い合わせ
コストダウンが利益に直結する術だと理解していても、なかなか前に進めることができない状況。そんな時は、newjiのコストダウン自動化機能で大きく利益貢献しよう!
(Β版非公開)