- お役立ち記事
- Basics of Bayesian statistics and practice of Bayesian estimation using Python
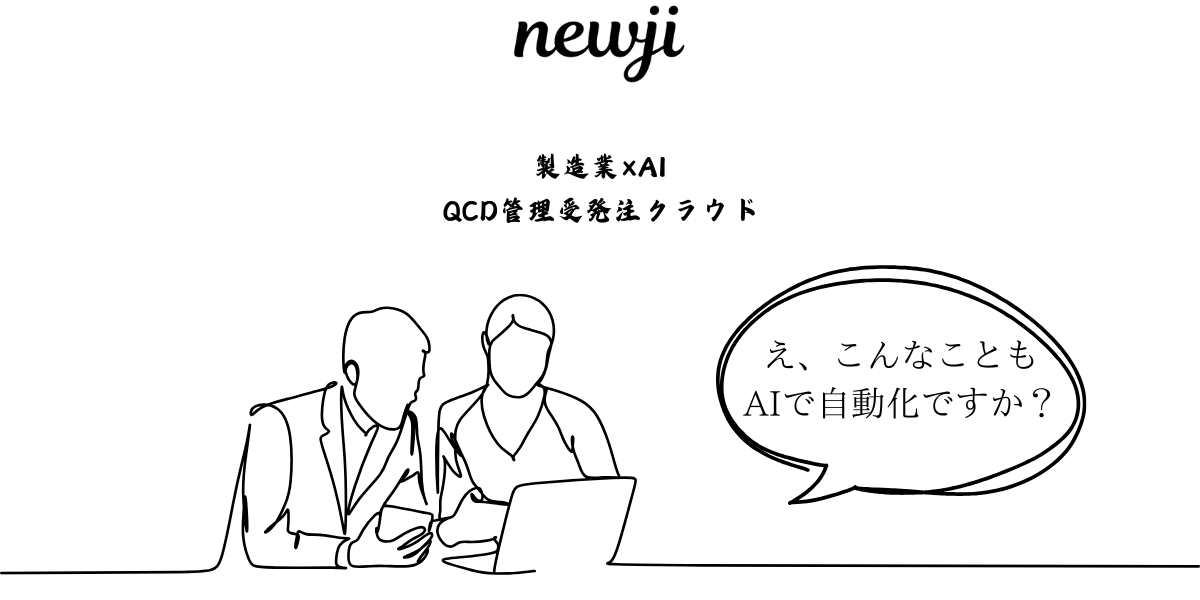
Basics of Bayesian statistics and practice of Bayesian estimation using Python
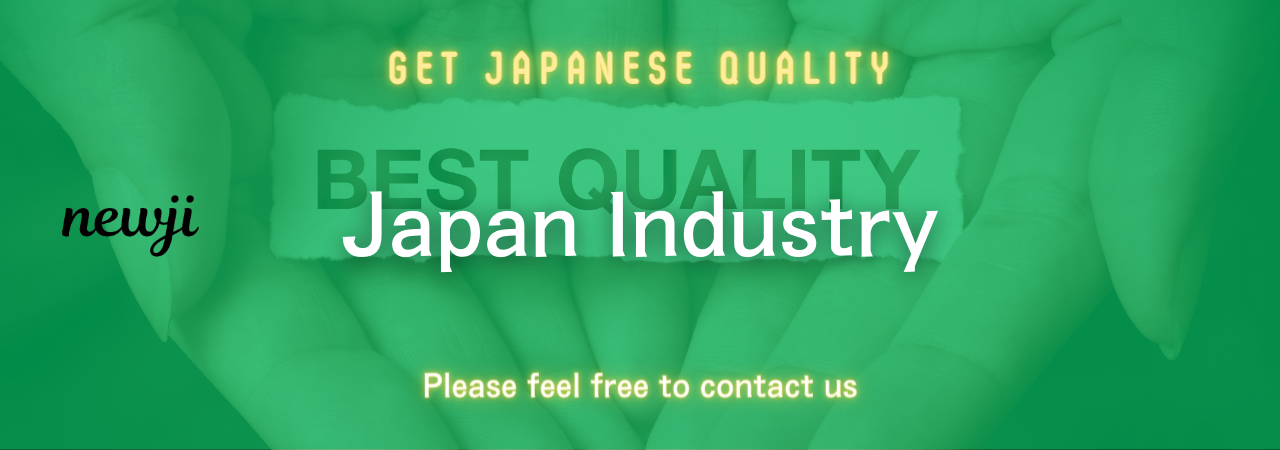
目次
What is Bayesian Statistics?
Bayesian statistics is a branch of statistics based on Bayes’ Theorem, which provides a probabilistic framework for updating beliefs based on new evidence or data.
It contrasts with classical or frequentist statistics by incorporating prior knowledge or beliefs into the statistical analysis.
In Bayesian analysis, probabilities are interpreted as degrees of belief about a hypothesis, rather than long-run frequencies.
Bayesian statistics revolves around the concept of updating our knowledge.
We start with an initial belief or prior distribution about a parameter or hypothesis.
As we gather data, we update this belief using Bayes’ Theorem to obtain a posterior distribution.
This posterior distribution then serves as the new foundation for making predictions or decisions.
Bayes’ Theorem Explained
Bayes’ Theorem is a mathematical formula that describes how to update the probabilities of hypotheses when given new evidence.
Mathematically, it can be expressed as:
P(H|E) = [P(E|H) * P(H)] / P(E)
Where:
– P(H|E) is the posterior probability, or the probability of the hypothesis H given the evidence E.
– P(E|H) is the likelihood, or the probability of observing the evidence E, given that hypothesis H is true.
– P(H) is the prior probability, or the initial probability of hypothesis H before observing the evidence.
– P(E) is the marginal probability, or the probability of observing the evidence under all possible hypotheses.
This theorem is fundamental in the process of Bayesian updating and is central to Bayesian analysis.
Applications of Bayesian Statistics
Bayesian statistics is widely used in various fields due to its flexibility and ability to incorporate prior information.
Some common applications include:
1. Medical Research
In medical research, Bayesian methods can incorporate prior clinical trials or expert opinion into the analysis of new data.
This is particularly useful in drug development, where historical data on drug effects can inform the design of new experiments.
2. Machine Learning
Bayesian methods underpin many machine learning algorithms, such as Bayesian networks and Gaussian processes.
These techniques are used for classification, regression, and clustering tasks, allowing for probabilistic predictions that include uncertainty estimates.
3. Finance
In finance, Bayesian statistics can improve models for stock returns, risk analysis, and portfolio optimization.
Prior beliefs about market conditions can be updated as new financial data becomes available, leading to more adaptive and responsive investment strategies.
4. Environmental Sciences
Researchers in environmental science use Bayesian statistics for modeling the impact of human activities on ecosystems.
By incorporating prior knowledge about environmental systems, Bayesian methods can offer more accurate predictions and assessments.
Bayesian Estimation Using Python
Python is a popular programming language that provides several libraries for Bayesian estimation and modeling.
Here, we’ll discuss a basic approach to implementing Bayesian statistics in Python.
1. Setting Up the Environment
First, you need to install the necessary Python libraries.
The most commonly used libraries for Bayesian statistics in Python are PyMC3, ArviZ, and NumPy.
You can install them using pip:
“`
pip install pymc3 arviz numpy
“`
2. Defining the Model
To illustrate Bayesian estimation, suppose we have a simple coin-flipping experiment.
Let’s say we want to estimate the probability of the coin landing heads-up (denoted as θ).
We start by defining a prior distribution for θ.
Assume a uniform prior, indicating no initial preference:
“`python
import pymc3 as pm
# Define the model
with pm.Model() as model:
theta = pm.Uniform(‘theta’, lower=0, upper=1)
“`
3. Specifying the Likelihood
Next, we incorporate new data, i.e., the result of several coin flips.
Assuming we flipped the coin 10 times and observed 7 heads, we use a binomial likelihood:
“`python
# Likelihood
observations = pm.Binomial(‘obs’, n=10, p=theta, observed=7)
“`
4. Performing Bayesian Inference
To update our prior belief, we perform Bayesian inference using the No-U-Turn Sampler (NUTS):
“`python
# Inference
trace = pm.sample(1000, return_inferencedata=False)
“`
5. Summarizing the Posterior
Finally, we analyze the results to obtain the posterior distribution of θ:
“`python
import arviz as az
# Summarize the trace
az.plot_trace(trace)
az.plot_posterior(trace)
“`
This approach provides us with a posterior distribution that represents updated beliefs about the coin’s probability of heads after observing the data.
Benefits of Bayesian Statistics
Bayesian statistics offers numerous benefits compared to classical statistical methods:
1. Incorporation of Prior Knowledge
Bayesian methods allow for the integration of prior knowledge or expert opinion into the analysis.
This flexibility is useful when data is sparse or previous research provides valuable insights.
2. Probabilistic Interpretation
Bayesian statistics provides probabilistic interpretations of parameters, allowing for meaningful uncertainty estimates.
This is particularly beneficial in fields where decision-making under uncertainty is crucial.
3. Model Comparison and Selection
Bayesian framework supports rigorous model comparison and selection, even with complex models.
This capability is important when dealing with multiple competing hypotheses or models.
Conclusion
Bayesian statistics is a powerful tool with a wide range of applications across various fields.
Its ability to update prior beliefs with new evidence makes it an invaluable approach for complex decision-making processes.
With tools like Python libraries PyMC3 and ArviZ, implementing Bayesian estimation has become more accessible to researchers and practitioners.
As data continues to grow in importance, understanding and applying Bayesian statistics will be a valuable skill for analyzing and interpreting data in a meaningful way.
Whether you’re in academia, industry, or a data-related profession, embracing Bayesian methods can enhance your work and lead to more informed conclusions.
資料ダウンロード
QCD調達購買管理クラウド「newji」は、調達購買部門で必要なQCD管理全てを備えた、現場特化型兼クラウド型の今世紀最高の購買管理システムとなります。
ユーザー登録
調達購買業務の効率化だけでなく、システムを導入することで、コスト削減や製品・資材のステータス可視化のほか、属人化していた購買情報の共有化による内部不正防止や統制にも役立ちます。
NEWJI DX
製造業に特化したデジタルトランスフォーメーション(DX)の実現を目指す請負開発型のコンサルティングサービスです。AI、iPaaS、および先端の技術を駆使して、製造プロセスの効率化、業務効率化、チームワーク強化、コスト削減、品質向上を実現します。このサービスは、製造業の課題を深く理解し、それに対する最適なデジタルソリューションを提供することで、企業が持続的な成長とイノベーションを達成できるようサポートします。
オンライン講座
製造業、主に購買・調達部門にお勤めの方々に向けた情報を配信しております。
新任の方やベテランの方、管理職を対象とした幅広いコンテンツをご用意しております。
お問い合わせ
コストダウンが利益に直結する術だと理解していても、なかなか前に進めることができない状況。そんな時は、newjiのコストダウン自動化機能で大きく利益貢献しよう!
(Β版非公開)