- お役立ち記事
- Time series data and pattern extraction
月間77,185名の
製造業ご担当者様が閲覧しています*
*2025年2月28日現在のGoogle Analyticsのデータより
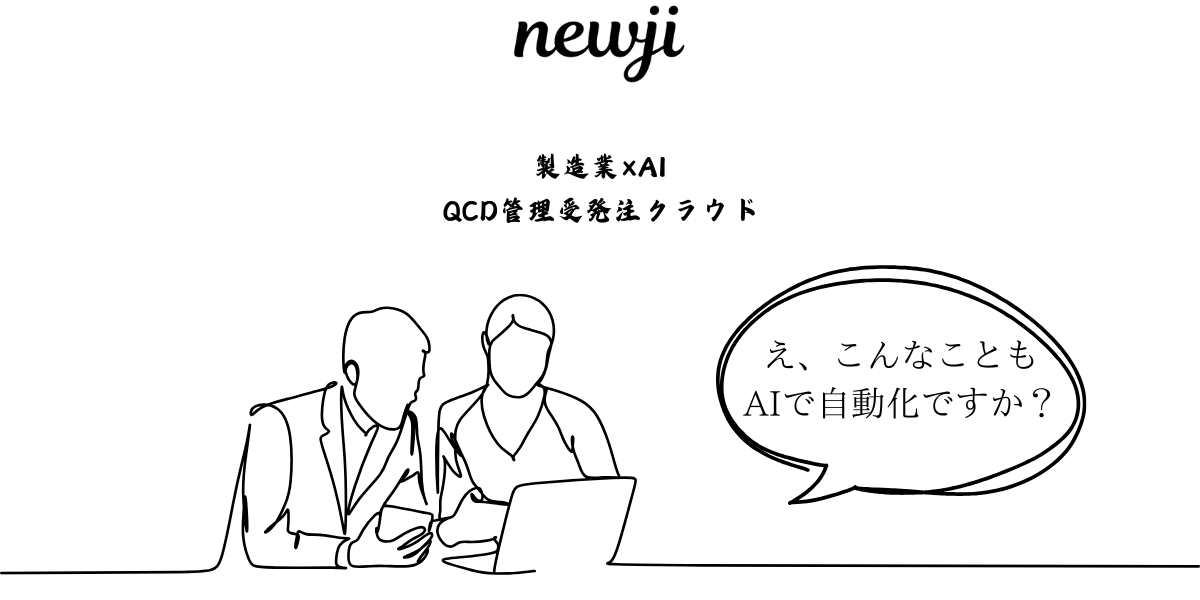
Time series data and pattern extraction
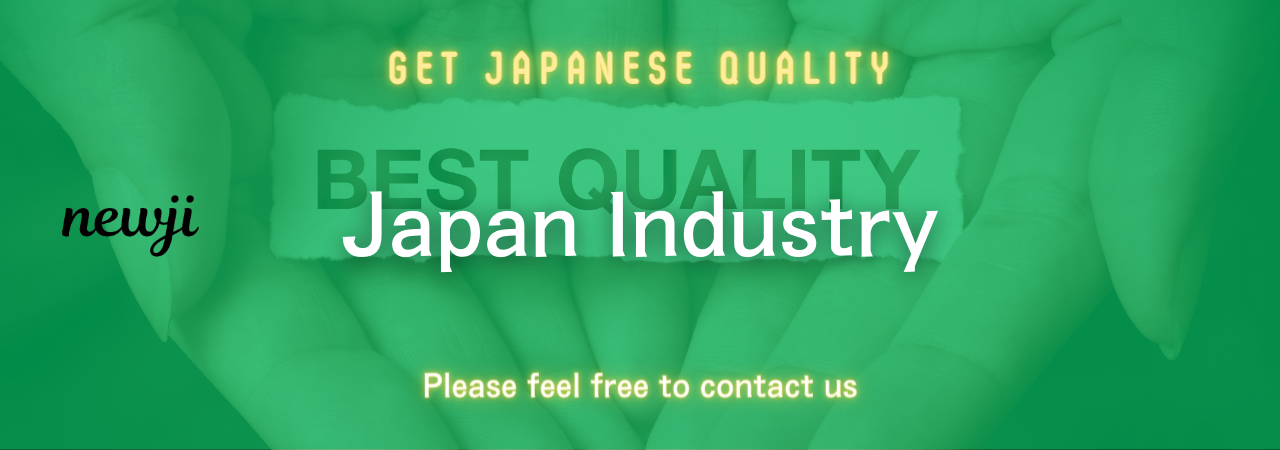
目次
Understanding Time Series Data
Time series data is a sequence of data points collected or recorded at specific time intervals.
This type of data is essential as it helps track changes over time, identify trends, and predict future outcomes.
The most common examples include stock prices, weather data, sales figures, and heart rate monitoring data.
The Significance of Time Series Analysis
Time series analysis involves examining data that have been collected over a period to understand the underlying structures and functions governing the observed phenomenon.
It helps in identifying patterns, trends, and forecasting future values.
For businesses, this can translate to better strategic planning as they understand market trends and customer behavior patterns.
Main Components of Time Series Data
There are generally four main components in time series data:
1. **Trend:** This refers to the long-term movement or direction in the data over an extended period.
A trend could be upward, downward, or stagnant.
2. **Seasonality:** These are periodic fluctuations that occur at regular intervals of time within a year.
For example, ice cream sales could increase during summer months and decrease during winter.
3. **Cyclic Patterns:** These occur when data exhibit fluctuations that are not of a fixed period but represent an occasional rise and fall.
Unlike seasonal patterns, cyclic patterns do not have a fixed frequency.
4. **Random Variations:** These are irregular fluctuations with no discernible pattern.
They can be caused by unexpected events or changes in conditions.
The Process of Pattern Extraction in Time Series Data
Data Collection and Preprocessing
The first step in pattern extraction is data collection.
The data must be accurate and relevant for meaningful analysis.
Once collected, the data might require preprocessing steps such as cleaning, normalization, and transformation to eliminate noise and prepare it for analysis.
Exploratory Data Analysis (EDA)
EDA is crucial in understanding the initial insights before proceeding with complex analyses.
It involves visualizing trends, checking for seasonality, identifying outliers, and understanding distributions using graphs and statistical methods.
Common tools for EDA include line plots, histograms, and scatter plots.
Pattern Recognition Techniques
Pattern recognition involves various techniques aimed at uncovering underlying patterns within the data.
– **Autocorrelation and Partial Autocorrelation:** Autocorrelation plots can help detect the presence of a pattern by comparing the time series with a lagged version of itself.
Partial autocorrelation is used to look at the correlation of the series with its lag without including the influence of intermediate lags.
– **Moving Averages:** This method involves averaging data points to smooth out short-term fluctuations and highlight longer-term trends or cycles.
– **Exponential Smoothing:** This technique captures trends, seasonality, and noise to help in forecasting.
It gives more weight to recent observations for more prompt response to changes.
– **Fourier Transform:** This is used to identify repeating patterns such as cycles by transforming the original time series data from time-domain to frequency-domain.
Ensuring Accuracy and Reliability
Checking the accuracy of the model is a key step in the analysis.
Cross-validation techniques such as rolling forecast origin or holdout validation can help ensure that the pattern extraction model is reliable.
Evaluating the performance using metrics like Mean Absolute Error (MAE), Root Mean Squared Error (RMSE), and Mean Absolute Percentage Error (MAPE) provides a quantitative measure of the model’s accuracy.
Applications of Time Series Pattern Extraction
Time series pattern recognition has several practical applications across industries.
Stock Market Analysis
In finance, time series analysis is crucial for predicting stock trends and dynamics.
Investors use the insights gained to make informed decisions on buying or selling stocks.
Weather Forecasting
Meteorologists use time series data to forecast weather conditions.
Analyzing patterns and cycles in historical weather data helps predict temperature changes, storms, and climate variations.
Healthcare Monitoring
Healthcare providers use time series data from vital signs monitoring equipment to detect abnormalities.
These patterns can indicate early signs of potential health issues, enabling timely medical interventions.
Sales and Revenue Forecasting
Businesses benefit from analyzing sales data to understand seasonal trends, future sales cycles, and inventory management.
These patterns help in crafting marketing strategies and improving business operations.
Conclusion
Understanding and extracting patterns from time series data is a powerful tool for making informed decisions.
Whether predicting financial trends, planning inventory, or monitoring patient health, time series analysis serves as a backbone in various fields by offering valuable insights into future events.
As technology advances, the methods for analyzing time series data continue to evolve, providing even more precise and actionable results.
資料ダウンロード
QCD管理受発注クラウド「newji」は、受発注部門で必要なQCD管理全てを備えた、現場特化型兼クラウド型の今世紀最高の受発注管理システムとなります。
ユーザー登録
受発注業務の効率化だけでなく、システムを導入することで、コスト削減や製品・資材のステータス可視化のほか、属人化していた受発注情報の共有化による内部不正防止や統制にも役立ちます。
NEWJI DX
製造業に特化したデジタルトランスフォーメーション(DX)の実現を目指す請負開発型のコンサルティングサービスです。AI、iPaaS、および先端の技術を駆使して、製造プロセスの効率化、業務効率化、チームワーク強化、コスト削減、品質向上を実現します。このサービスは、製造業の課題を深く理解し、それに対する最適なデジタルソリューションを提供することで、企業が持続的な成長とイノベーションを達成できるようサポートします。
製造業ニュース解説
製造業、主に購買・調達部門にお勤めの方々に向けた情報を配信しております。
新任の方やベテランの方、管理職を対象とした幅広いコンテンツをご用意しております。
お問い合わせ
コストダウンが利益に直結する術だと理解していても、なかなか前に進めることができない状況。そんな時は、newjiのコストダウン自動化機能で大きく利益貢献しよう!
(β版非公開)