- お役立ち記事
- Fundamentals of Bayesian signal processing and applications to noise removal
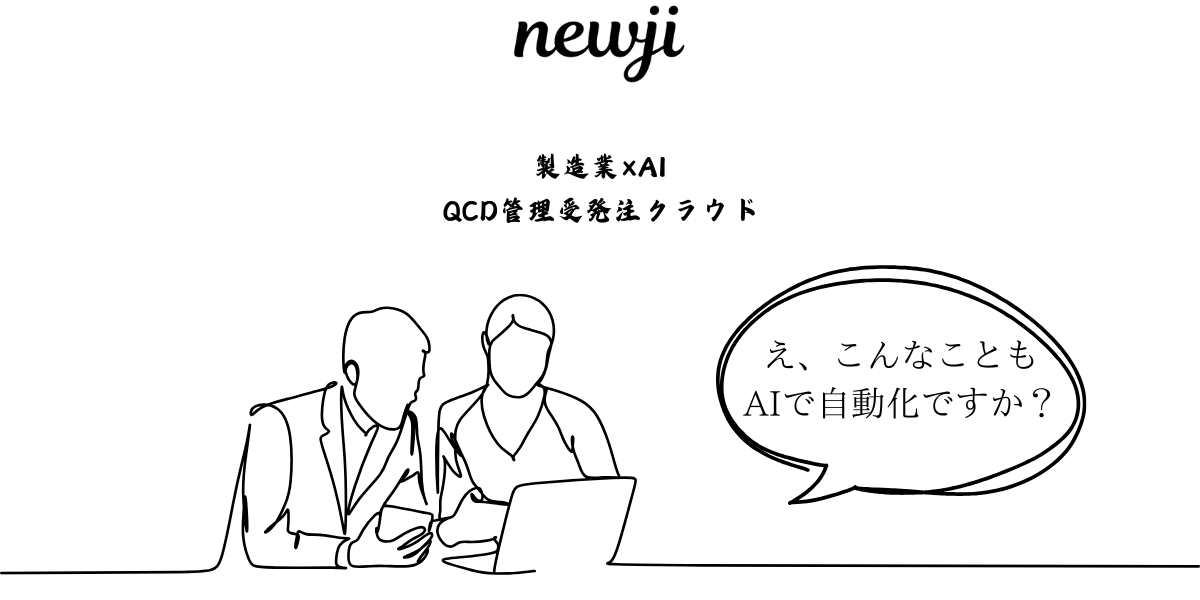
Fundamentals of Bayesian signal processing and applications to noise removal
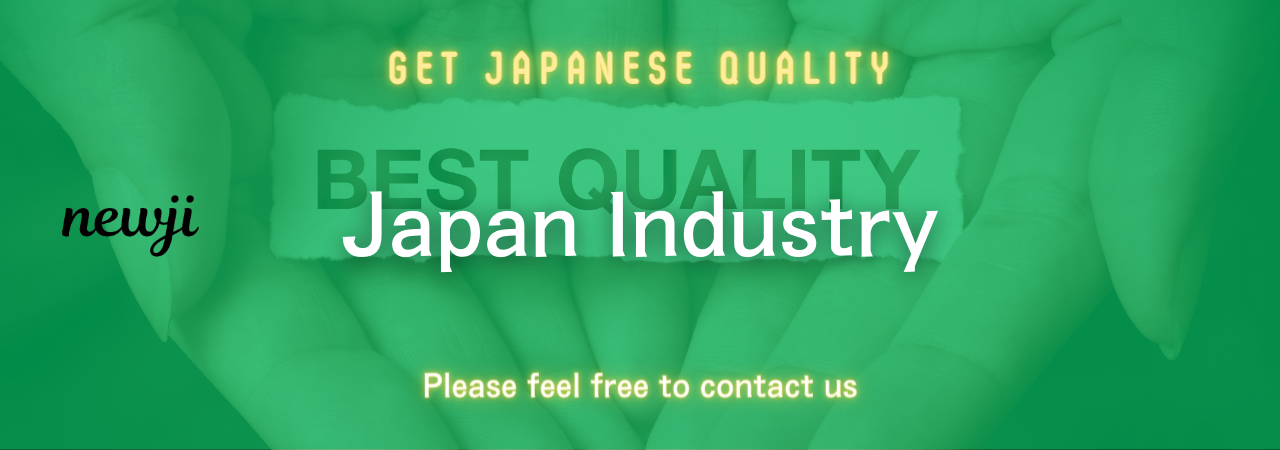
目次
Introduction to Bayesian Signal Processing
Bayesian signal processing is a powerful framework used to handle uncertainty in signals and data through the use of probability theory.
This approach is particularly useful in scenarios where noise can obscure the true information within a signal.
At its core, Bayesian signal processing combines prior knowledge with observed data to arrive at a better understanding or enhanced signal.
Whether you’re dealing with audio signals, image processing, or communication systems, Bayesian methods can significantly improve the quality of the processed outcome by effectively managing noise.
In this article, we will explore the basics of Bayesian signal processing and its applications in noise removal.
Why Use Bayesian Methods?
Bayesian methods offer several advantages:
1. **Incorporation of Prior Knowledge**: Unlike other approaches, Bayesian methods allow us to integrate prior knowledge about the signal or system.
This is crucial when dealing with uncertainties or incomplete data.
2. **Flexibility**: These methods can be adapted to a wide variety of problems, making them incredibly flexible and robust in applications such as noise reduction, estimation, and classification.
3. **Probabilistic Interpretation**: The Bayesian framework provides results in terms of probabilities, which can offer insights into the confidence of the estimates and decisions made.
4. **Iterative Updates**: Bayesian methods allow for iterative updates as new data becomes available, leading to progressively better solutions.
Understanding Bayesian Probability
At the heart of Bayesian signal processing is Bayes’ theorem, which relates conditional and marginal probabilities:
\[ P(A|B) = \frac{P(B|A) \cdot P(A)}{P(B)} \]
Where:
– \( P(A|B) \) is the posterior probability of A given B, representing our updated belief after observing B.
– \( P(B|A) \) is the likelihood, indicating how probable the observation B is when A is true.
– \( P(A) \) is the prior probability of A, representing our initial belief before observing B.
– \( P(B) \) is the evidence or total probability of observing B.
This theorem is the backbone of Bayesian analysis, informing us how to update our beliefs based on new evidence.
Bayesian Signal Processing Applications
Bayesian signal processing can be applied across different domains. Below are some key applications where it serves as a valuable tool:
1. Noise Removal
One of the most prevalent uses of Bayesian techniques is in noise removal.
In signal processing, noise can significantly degrade the quality of the signal.
Bayesian methods can filter out the noise by estimating the underlying true signal through posterior probabilities.
For instance, in audio processing, Bayesian filters can distinguish between speech and background noise, thus amplifying the desired sound while suppressing the noise.
2. Image Processing
In image processing, Bayesian approaches are utilized to enhance image quality by reducing the noise.
This is particularly important in medical imaging, where clarity is crucial for accurate diagnosis.
By employing prior knowledge about image properties, Bayesian models can effectively reconstruct images with minimal noise interference.
3. Communications
Bayesian signal processing plays a vital role in declining interference and improving data transmission in communication systems.
By employing Bayesian methods, systems can adaptively enhance signals, leading to better communication quality and reduced error rates.
4. Sensor Networks
In sensor networks, data from multiple sensors can be noisy or incomplete.
Bayesian methods help in fusing data from different sources to create a clearer picture, enhancing overall decision-making and estimation processes.
Steps in Bayesian Signal Processing
The process typically involves the following steps:
1. **Model Specification**: Define the probabilistic models for both the signal and noise.
This involves specifying the prior distribution for the parameters based on existing knowledge or assumptions.
2. **Inference**: Use observed data to update beliefs about the signal.
This often involves the computation of the posterior distribution using Bayes’ theorem.
3. **Estimation**: From the posterior distribution, estimate the desired signal or parameters.
This can be done using techniques such as Maximum A Posteriori (MAP) estimation or Bayesian estimation.
4. **Prediction and Decision Making**: Using the estimated parameters, make predictions or decisions that are probabilistically informed.
Challenges and Considerations
While Bayesian methods offer remarkable advantages, they present challenges, such as:
1. **Computational Complexity**: Bayesian computations, particularly in high-dimensional spaces, can be computationally intensive.
2. **Model Complexity**: Choosing and specifying the correct models and priors requires domain expertise and can significantly impact results.
3. **Convergence**: Iterative Bayesian methods, like those employing Markov Chain Monte Carlo (MCMC), require careful convergence checks to ensure accurate results.
Conclusion
Bayesian signal processing provides a robust framework for dealing with noisy data across a variety of applications.
Through the careful integration of prior knowledge and observed evidence, Bayesian methods enhance signal quality, leading to better decision-making and insights.
As technologies continue to advance, the role of Bayesian methods in signal processing will likely continue to grow, providing clearer and more accurate results in increasingly complex domains.
資料ダウンロード
QCD調達購買管理クラウド「newji」は、調達購買部門で必要なQCD管理全てを備えた、現場特化型兼クラウド型の今世紀最高の購買管理システムとなります。
ユーザー登録
調達購買業務の効率化だけでなく、システムを導入することで、コスト削減や製品・資材のステータス可視化のほか、属人化していた購買情報の共有化による内部不正防止や統制にも役立ちます。
NEWJI DX
製造業に特化したデジタルトランスフォーメーション(DX)の実現を目指す請負開発型のコンサルティングサービスです。AI、iPaaS、および先端の技術を駆使して、製造プロセスの効率化、業務効率化、チームワーク強化、コスト削減、品質向上を実現します。このサービスは、製造業の課題を深く理解し、それに対する最適なデジタルソリューションを提供することで、企業が持続的な成長とイノベーションを達成できるようサポートします。
オンライン講座
製造業、主に購買・調達部門にお勤めの方々に向けた情報を配信しております。
新任の方やベテランの方、管理職を対象とした幅広いコンテンツをご用意しております。
お問い合わせ
コストダウンが利益に直結する術だと理解していても、なかなか前に進めることができない状況。そんな時は、newjiのコストダウン自動化機能で大きく利益貢献しよう!
(Β版非公開)