- お役立ち記事
- Modeling of controlled objects and application to model predictive control
Modeling of controlled objects and application to model predictive control
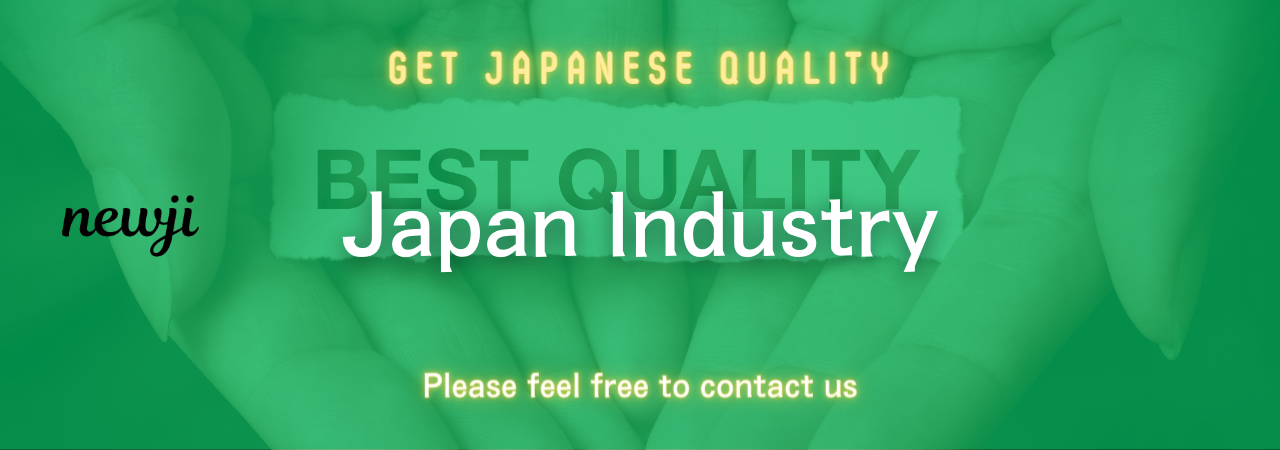
Understanding Model Predictive Control
Model Predictive Control (MPC) is a powerful and versatile method used extensively in industrial control systems to optimize the performance of dynamic processes.
The fundamental idea behind MPC is to predict the future behavior of a system using a mathematical model and then optimize the control inputs based on this prediction.
By continuously updating this model and adjusting the inputs, MPC ensures optimal performance even in the presence of disturbances or changing conditions.
At the heart of MPC is a process model that describes the behavior of the system you want to control.
This model can be linear or nonlinear, and its accuracy significantly impacts the effectiveness of the control strategy.
The model is used to predict how the system will respond over a specified future horizon, allowing the controller to plan the optimal set of control actions to achieve desired outcomes.
The Role of Controlled Object Modeling
The development of an accurate model of the controlled object, or process, is a crucial step in implementing model predictive control.
This is because the model serves as the foundation for predicting future system behavior.
The first step in modeling a controlled object is identifying the key variables that influence its behavior.
These variables could be inputs to the system, such as actuator positions or external disturbances, and outputs, such as temperature, pressure, or flow rate.
To create this model, engineers typically use mathematical equations to represent the relationships between these variables.
For linear systems, simple linear equations may suffice, but for more complex processes, nonlinear models might be necessary.
In some cases, data-driven techniques like machine learning can be employed to develop models based on historical data.
Once the model is established, it needs to be validated against the actual system to ensure it accurately represents its dynamics.
Model validation involves comparing the predicted outputs of the model with actual measured data from the system.
If discrepancies are found, the model may need further refinement to improve its accuracy.
Utilizing the Model in Model Predictive Control
In model predictive control, the validated model is used to predict future states of the system over a defined prediction horizon.
The control algorithm then calculates the optimal control inputs that will drive the system towards the desired setpoint or trajectory while respecting any constraints on inputs and outputs.
This optimization problem is typically solved using advanced mathematical techniques such as quadratic programming or linear programming.
The control horizon in MPC specifies the duration over which the controller must compute the optimal inputs.
Typically, the prediction horizon is longer than the control horizon, allowing for foresight into future system behavior.
By solving this optimization problem at each timestep, MPC offers robust and adaptive control, capable of handling multivariable systems and constraints seamlessly.
An exciting feature of MPC is its ability to incorporate constraints into the control design explicitly.
Real-world systems often have physical or operational constraints, such as upper or lower bounds for actuators or safety limits for process variables.
MPC readily handles these constraints by embedding them directly into the optimization problem, ensuring the optimal control action never violates them.
Applications of Model Predictive Control
Model Predictive Control is widely used across various industries due to its flexibility and adaptability.
In the chemical and petrochemical industries, MPC is implemented in process control systems to optimize parameters like temperature, pressure, and composition, enhancing safety and efficiency.
MPC has become indispensable in these industries due to its ability to manage complex, nonlinear, and multivariable processes.
In the automotive industry, MPC plays a significant role in advanced driver-assistance systems (ADAS) and autonomous driving.
It aids in vehicle stability control, adaptive cruise control, and trajectory planning, ensuring optimal vehicle performance and safety.
With the advent of electric and autonomous vehicles, the importance of MPC in this sector continues to grow.
The energy sector, including oil and gas, renewable energy, and power generation, also benefits from MPC.
It helps optimize the operation of power plants, wind turbines, and grids, improving efficiency and reducing emissions.
In the context of smart grids, MPC is vital for managing distributed energy resources and maintaining grid stability.
Furthermore, the use of MPC in aerospace applications allows aircraft systems to achieve precise trajectory control and manage fuel consumption efficiently.
It is used in spacecraft navigation for tasks such as attitude control and orbit maintenance, showcasing the versatility of MPC in demanding environments.
Future Prospects and Challenges
Despite its numerous advantages, implementing Model Predictive Control poses several challenges.
The development of accurate process models can be time-consuming and requires expertise, especially for complex nonlinear systems.
Additionally, real-time computation of the optimization problem demands significant computational resources.
However, advances in computational power and techniques like machine learning are addressing these challenges, making MPC more accessible and practical for a wider range of applications.
The integration of MPC with artificial intelligence and machine learning is a promising area, offering opportunities for improving model accuracy and computational efficiency.
As industries continue to automate and optimize their operations, the demand for adaptive and intelligent control systems like MPC will only increase.
The growth of Industry 4.0 and the Internet of Things (IoT) presents exciting possibilities for the integration of MPC in smart manufacturing and connected devices, paving the way for more efficient and responsive systems.
MPC’s potential to contribute to sustainability is another key area of interest.
By optimizing resource use and reducing waste, MPC can play a vital role in achieving energy efficiency and minimizing environmental impacts, aligning with global sustainability goals.
In summary, Model Predictive Control stands as a cornerstone in advanced control engineering, with widespread applications across industries.
The continued development of modeling techniques and computational tools promises to enhance its effectiveness, broadening its horizons in the future.
資料ダウンロード
QCD調達購買管理クラウド「newji」は、調達購買部門で必要なQCD管理全てを備えた、現場特化型兼クラウド型の今世紀最高の購買管理システムとなります。
ユーザー登録
調達購買業務の効率化だけでなく、システムを導入することで、コスト削減や製品・資材のステータス可視化のほか、属人化していた購買情報の共有化による内部不正防止や統制にも役立ちます。
NEWJI DX
製造業に特化したデジタルトランスフォーメーション(DX)の実現を目指す請負開発型のコンサルティングサービスです。AI、iPaaS、および先端の技術を駆使して、製造プロセスの効率化、業務効率化、チームワーク強化、コスト削減、品質向上を実現します。このサービスは、製造業の課題を深く理解し、それに対する最適なデジタルソリューションを提供することで、企業が持続的な成長とイノベーションを達成できるようサポートします。
オンライン講座
製造業、主に購買・調達部門にお勤めの方々に向けた情報を配信しております。
新任の方やベテランの方、管理職を対象とした幅広いコンテンツをご用意しております。
お問い合わせ
コストダウンが利益に直結する術だと理解していても、なかなか前に進めることができない状況。そんな時は、newjiのコストダウン自動化機能で大きく利益貢献しよう!
(Β版非公開)