- お役立ち記事
- Basics of reliability data analysis/Weibull analysis and practical points for failure rate/life prediction
Basics of reliability data analysis/Weibull analysis and practical points for failure rate/life prediction
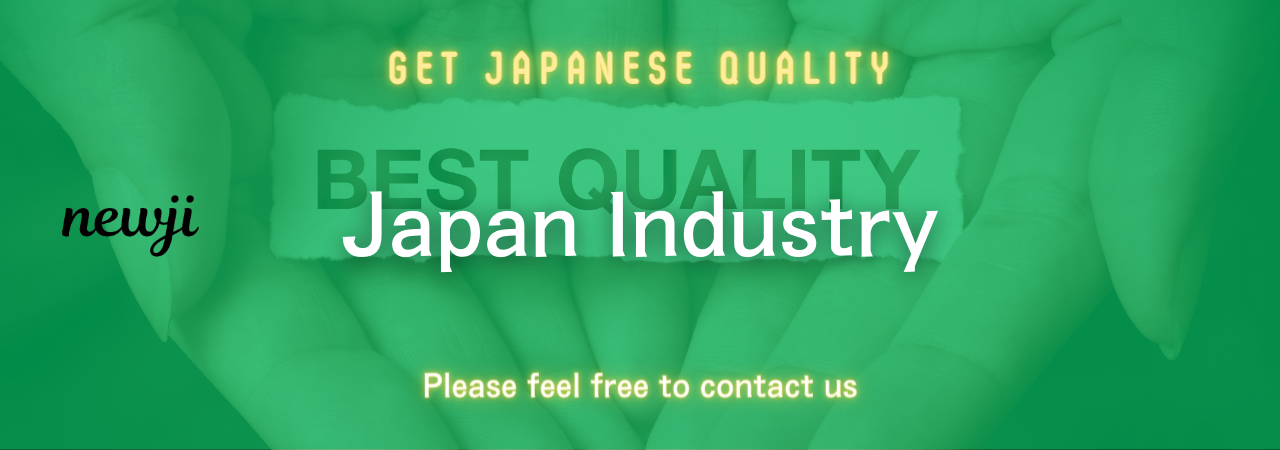
目次
Understanding Reliability Data Analysis
Reliability data analysis is a crucial aspect when it comes to predicting the lifespan and failure rates of products and systems.
This form of analysis helps businesses and engineers understand how products perform over time, ensuring they deliver optimum quality and dependability to consumers.
Reliability data analysis involves using past data to predict future performance, which allows for informed decision-making and strategic planning.
The Role of Weibull Analysis
Weibull analysis is a statistical technique often used in reliability engineering and failure analysis.
Named after Wallodi Weibull, this method is relatively simple and provides insights into various life data points.
Weibull analysis is used to model things like reliability, failure rates, and life data of products during different phases of their lifecycle.
The beauty of the Weibull method lies in its versatility.
It can effectively represent a wide array of data types, helping organizations evaluate the reliability of a product.
This technique can be pivotal during product design, development, and even during post-production support.
Understanding Weibull Distribution
At the core of Weibull analysis is the Weibull distribution.
The distribution helps in understanding the time until a particular failure will occur, which is instrumental in life prediction endeavors.
The Weibull distribution utilizes two primary parameters: shape parameter (often denoted as Beta, β) and scale parameter (denoted as Eta, η).
The shape parameter determines the type of distribution.
When β has a value less than 1, it suggests that the failure rate decreases over time, usually typical for products improving after debugging.
A β value of 1 indicates a constant failure rate, often seen in random failures.
A β greater than 1 suggests the failure rate increases over time, common in aging-related failures.
The scale parameter (η) provides a scale for the Weibull distribution, signifying the characteristic life where approximately 63.2% of the population will fail.
Why Weibull Analysis is Important
Weibull analysis aids in understanding failure behavior, which in turn has numerous practical implications.
Businesses can perform life testing for their products, leveraging the results to enhance product reliability.
Manufacturers can minimize warranty claims and operational costs while boosting customer satisfaction through reliable products.
Additionally, Weibull analysis helps in resource allocation by predicting when maintenance activities are necessary, preventing sudden failures.
Deciphering the failure patterns also allows businesses to innovate and improve their designs, fostering a culture of continuous improvement.
Practical Points for Failure Rate Prediction
Given the effectiveness of Weibull analysis, implementing it with precision is key to obtaining reliable predictions on failure rates and product life.
Data Collection and Preparation
The first step is gathering accurate failure data.
Ensuring data validity is crucial because inaccurate data can render your analysis meaningless, leading to incorrect predictions.
The data should encompass all potential failure modes of a product for comprehensive insights.
Ensure that the data is prepared correctly for analysis.
This involves filtering for relevant data, eliminating anomalies, and choosing the appropriate sampling mechanisms.
Parameter Estimation
Estimating Weibull parameters is an essential step in the process.
Several methods exist, like Maximum Likelihood Estimation (MLE) and Rank Regression (RR), each with its own use cases and advantages.
Choosing the correct method depends on the nature of your data and the insights you seek to obtain.
Model Validation
To assure the accuracy of the predictive model, it’s vital to validate it against historical data.
This step ensures that the conclusions drawn will be credible and will reflect in practical scenarios.
Use goodness-of-fit tests or plotting positions to validate the model.
Predicting Life Expectancy
With validated models, predicting the life expectancy of products becomes straightforward.
Decision-makers can use these predictions to schedule maintenance effectively, manage inventory better, and design with reliability in mind.
Life expectancy predictions also enable businesses to perform cost-benefit analyses for improvements or innovations in product lines, aligning their strategic planning with realistic projections.
Communicating Findings
After conducting a Weibull analysis, effectively communicating findings is essential.
Clear, concise reporting ensures that stakeholders can make informed choices based on the data.
Visual tools like Weibull plots or timeline graphs can aid in making the information digestible and actionable.
Conclusion
In today’s competitive landscape, understanding the nuances of reliability data analysis and Weibull methodologies can be a game-changer for businesses.
A well-executed Weibull analysis can provide vital insights, guiding organizations towards delivering robust, reliable products to market.
By embracing these techniques, companies can not only predict failures but also preempt them, ensuring customer satisfaction and brand loyalty.
資料ダウンロード
QCD調達購買管理クラウド「newji」は、調達購買部門で必要なQCD管理全てを備えた、現場特化型兼クラウド型の今世紀最高の購買管理システムとなります。
ユーザー登録
調達購買業務の効率化だけでなく、システムを導入することで、コスト削減や製品・資材のステータス可視化のほか、属人化していた購買情報の共有化による内部不正防止や統制にも役立ちます。
NEWJI DX
製造業に特化したデジタルトランスフォーメーション(DX)の実現を目指す請負開発型のコンサルティングサービスです。AI、iPaaS、および先端の技術を駆使して、製造プロセスの効率化、業務効率化、チームワーク強化、コスト削減、品質向上を実現します。このサービスは、製造業の課題を深く理解し、それに対する最適なデジタルソリューションを提供することで、企業が持続的な成長とイノベーションを達成できるようサポートします。
オンライン講座
製造業、主に購買・調達部門にお勤めの方々に向けた情報を配信しております。
新任の方やベテランの方、管理職を対象とした幅広いコンテンツをご用意しております。
お問い合わせ
コストダウンが利益に直結する術だと理解していても、なかなか前に進めることができない状況。そんな時は、newjiのコストダウン自動化機能で大きく利益貢献しよう!
(Β版非公開)