- お役立ち記事
- Fundamentals of precision control using data-driven learning and application to controller design
Fundamentals of precision control using data-driven learning and application to controller design
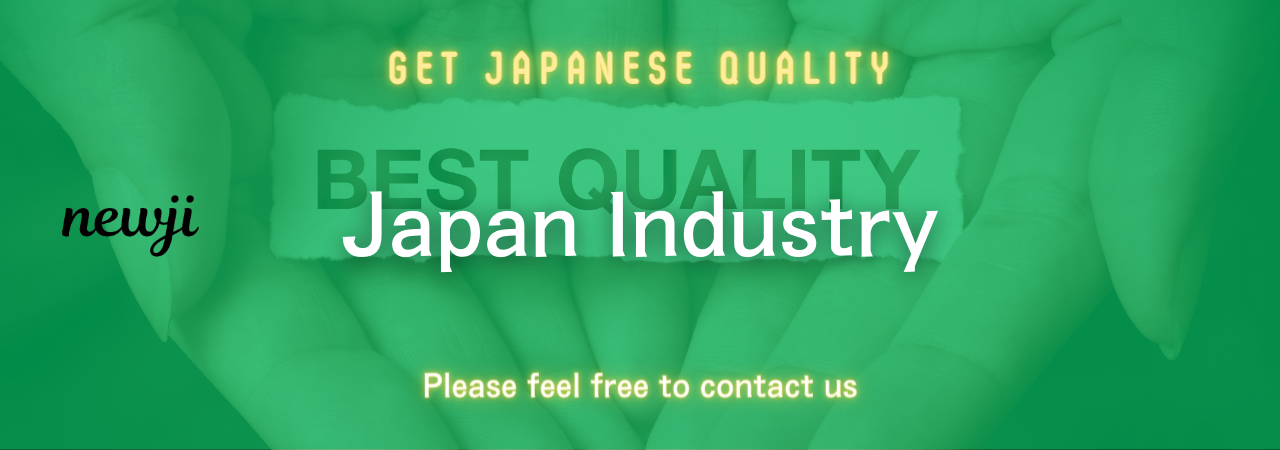
目次
Understanding Precision Control
Precision control is a vital component in many industrial and technological applications.
It ensures that machines and systems operate with high accuracy and efficiency.
At its core, precision control is about accurately managing and adjusting processes to meet desired outcomes.
Historically, it relied heavily on pre-determined models and parameters set by human experts.
But with the advent of data-driven learning, this domain is witnessing a significant transformation.
Data-Driven Learning in Precision Control
Data-driven learning marks a shift from traditional control methods.
Instead of relying solely on predefined models, systems now leverage vast amounts of data to make decisions.
This approach provides several advantages.
Firstly, it allows systems to adapt to changing conditions and environments without manual reprogramming.
Secondly, it harnesses real-time data to improve accuracy and performance over time.
Machine learning, a subset of artificial intelligence, plays a crucial role in this transformation.
Through machine learning algorithms, systems can analyze and interpret large datasets to uncover patterns and trends.
These insights enable machines to learn from their experiences and make informed decisions independently.
Benefits of Data-Driven Learning
1. **Increased Adaptability**: Systems are no longer static; they can adjust to new situations seamlessly.
2. **Enhanced Accuracy**: By continuously learning and refining based on real-time data, systems achieve higher precision.
3. **Predictive Capabilities**: Data-driven systems can anticipate potential issues or deviations and act proactively.
4. **Reduced Human Intervention**: Automation of analysis and decision-making reduces the need for constant human oversight.
Applications of Precision Control
Precision control is not limited to a single domain.
Its applications span across various industries, making it a versatile and indispensable tool.
Manufacturing
In manufacturing, precision control is crucial for maintaining product quality and efficiency.
Data-driven learning helps in optimizing production lines and minimizing waste.
For example, predictive maintenance uses real-time data to foresee equipment failures before they occur, reducing downtime.
Robotics
Robotics heavily relies on precision control for smooth and accurate movements.
With data-driven learning, robots can adapt their actions based on new data input, enhancing their functionality and safety.
This capability is particularly valuable in dynamic environments such as manufacturing floors or hazardous sites.
Aerospace
The aerospace industry demands exceptionally high levels of accuracy and reliability.
Precision control helps in navigation, flight control, and system monitoring.
Data-driven learning supports these efforts by providing systems with the ability to adjust to varying atmospheric conditions and operational demands, ensuring safe and efficient flights.
Healthcare
In the healthcare sector, precision control aids in the development of advanced medical devices and diagnostic tools.
These devices can offer real-time monitoring and adjust treatments as necessary.
For instance, data-driven algorithms can personalize drug dosages for patients, optimizing treatment outcomes.
Energy Sector
Energy production and distribution benefit significantly from precision control.
Data-driven strategies enhance grid management and optimize energy usage.
By analyzing consumption patterns and predicting demand, systems can effectively balance load distribution, reducing energy waste and improving supply continuity.
Designing Controllers with Data-Driven Learning
Controller design is a critical aspect where precision control principles are applied.
Designing a controller traditionally required extensive expertise and experience to set appropriate parameters.
With the integration of data-driven learning, this process is becoming more streamlined and effective.
Steps in Controller Design
1. **Data Collection**: Gathering comprehensive datasets from the system is the first step. This data will later inform the learning algorithms.
2. **Model Training**: Machine learning models are trained using the collected data. This step involves identifying relationships and patterns that will inform the controller’s decisions.
3. **Simulation and Testing**: Before full-scale implementation, simulation tools are used to test the controller in various scenarios, ensuring that it performs as expected.
4. **Deployment**: Once solutions are validated, the controller is deployed to the actual system, where it operates based on the learned parameters.
5. **Continuous Learning**: The control system continues to receive data post-deployment. This data is used to refine and enhance the controller’s performance continually.
Challenges and Future Directions
While the benefits of data-driven learning in precision control are clear, certain challenges remain.
Data Quality and Quantity
The accuracy of a data-driven system is heavily dependent on the quality and richness of the data it uses.
Incomplete or biased datasets can lead to inaccurate predictions and decisions.
Therefore, ensuring robust data collection and management practices is essential.
Computational Power
Processing vast amounts of data requires significant computational resources.
Organizations need to invest in advanced computing infrastructure to handle the demands of data-driven learning.
Security and Privacy
Handling sensitive and proprietary data poses security risks.
Implementing stringent data protection measures is vital to safeguard information and maintain user trust.
The future of precision control with data-driven learning looks promising.
Continued developments in machine learning algorithms, coupled with advancements in computing technology, will further enhance its capabilities.
As industries continue to embrace digital transformation, precision control will become even more central to operations, ensuring efficiency and innovation across the board.
資料ダウンロード
QCD調達購買管理クラウド「newji」は、調達購買部門で必要なQCD管理全てを備えた、現場特化型兼クラウド型の今世紀最高の購買管理システムとなります。
ユーザー登録
調達購買業務の効率化だけでなく、システムを導入することで、コスト削減や製品・資材のステータス可視化のほか、属人化していた購買情報の共有化による内部不正防止や統制にも役立ちます。
NEWJI DX
製造業に特化したデジタルトランスフォーメーション(DX)の実現を目指す請負開発型のコンサルティングサービスです。AI、iPaaS、および先端の技術を駆使して、製造プロセスの効率化、業務効率化、チームワーク強化、コスト削減、品質向上を実現します。このサービスは、製造業の課題を深く理解し、それに対する最適なデジタルソリューションを提供することで、企業が持続的な成長とイノベーションを達成できるようサポートします。
オンライン講座
製造業、主に購買・調達部門にお勤めの方々に向けた情報を配信しております。
新任の方やベテランの方、管理職を対象とした幅広いコンテンツをご用意しております。
お問い合わせ
コストダウンが利益に直結する術だと理解していても、なかなか前に進めることができない状況。そんな時は、newjiのコストダウン自動化機能で大きく利益貢献しよう!
(Β版非公開)