- お役立ち記事
- Fundamentals of speech recognition technology, improvement of recognition accuracy through deep learning, and application to speech recognition systems
Fundamentals of speech recognition technology, improvement of recognition accuracy through deep learning, and application to speech recognition systems
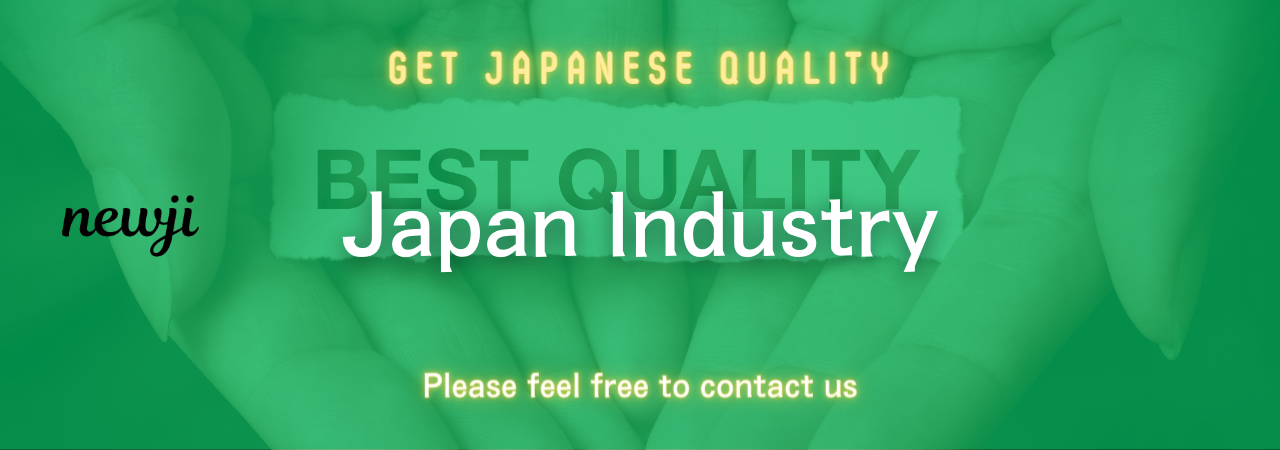
目次
Understanding Speech Recognition Technology
Speech recognition technology has come a long way since its inception, evolving from simple voice commands to complex systems capable of understanding and processing natural human language.
At its core, speech recognition involves converting spoken words into text.
This process is more intricate than it sounds, as it requires the system to accurately capture the nuances of human speech, including different accents, speech speeds, and intonations.
In the early days, speech recognition systems relied on rule-based models and simple algorithms.
These systems could only recognize limited vocabularies and often required users to speak in a monotonous tone or slower pace for accurate results.
Due to technological and computational constraints, they were mainly used in controlled environments, such as call centers and voice-operated controls in industrial settings.
The Role of Acoustic and Language Models
Modern speech recognition systems use two primary models: acoustic and language models.
Acoustic models analyze the sound waves of speech, breaking down the waves into phonemes, the smallest units of sound.
These phonemes are then compared against stored data to identify words.
The accuracy of this process is greatly influenced by the quality and quantity of the training data used in the models.
Language models, on the other hand, focus on context, predicting the probability of word sequences in a language.
They rely on large corpuses of text data to understand language patterns, grammar, and syntax.
By combining acoustic signals with language context, today’s speech recognition systems can interpret continuous speeches with higher accuracy.
The Impact of Deep Learning on Recognition Accuracy
One of the most transformative advancements in speech recognition has been the integration of deep learning techniques.
Deep learning involves neural networks with many layers, capable of learning complex patterns in large datasets.
This technology has significantly improved the capability of speech recognition systems, allowing them to process more natural and varied speech inputs.
With the introduction of deep learning, acoustic models are now built using deep neural networks (DNNs).
These models are exceptionally proficient at identifying sound patterns, even in noisy environments, which was a significant limitation of earlier systems.
DNNs can learn from vast amounts of voice data, improving their ability to generalize from past experiences to understand new speech inputs better.
Recurrent Neural Networks and Long Short-Term Memory
Recurrent neural networks (RNNs) and their variant, long short-term memory networks (LSTMs), have further advanced speech recognition.
These networks are designed to understand temporal sequences, which is crucial for processing natural speech that varies in speed and rhythm.
RNNs and LSTMs contribute to language models by remembering previous inputs and using them to predict future words in a sentence.
This feature allows them to understand context better and provide more accurate transcriptions.
For instance, they can discern the difference between homophones, like “there” and “their,” based on the surrounding text.
Practical Applications of Speech Recognition Systems
With advancements in deep learning, speech recognition systems are now more reliable and versatile, finding applications in numerous areas.
These systems have made their way into consumer electronics, business operations, healthcare, and even vehicles.
Virtual Assistants
Perhaps one of the most well-known applications is the integration of speech recognition in virtual assistants like Apple’s Siri, Amazon’s Alexa, and Google Assistant.
These assistants perform tasks based on voice commands, such as setting reminders, searching the web, or controlling smart home devices.
They are designed to understand natural language, enhancing user interaction and accessibility.
Automated Customer Support
Speech recognition technology is also widely used in automated customer support systems.
By understanding natural language, these systems can guide users through troubleshooting processes, handle inquiries, and even process reservations or purchases without needing human intervention.
This reduces wait times and operational costs for companies while providing customers with quicker service.
Transcription Services
In education and business, speech recognition systems are utilized for transcription services.
They can convert lectures, meetings, or interviews into text quickly, enhancing accessibility and allowing for better record-keeping.
These transcriptions can then be used for further analysis and documentation.
Accessibility and Assistive Technologies
For individuals with disabilities, speech recognition technology can serve as an invaluable tool.
It facilitates communication through voice-to-text capabilities and controls devices with voice commands, offering greater independence and accessibility.
Challenges and Future Directions
Despite significant advancements, speech recognition technology still faces challenges, particularly with understanding varied dialects, slang, and languages with smaller datasets.
Another challenge is improving the understanding of speech in noisy environments or with low-quality microphones.
While deep learning has improved noise filtering and environmental adaptation, there’s still room for refinement.
In the future, we can expect further improvements in personalization, where systems will better understand individual speech patterns over time.
Furthermore, ongoing advancements in machine learning algorithms and hardware will likely lead to more robust and efficient speech recognition capabilities.
As this technology continues to evolve, it will play an increasingly important role in how we interact with our devices and the world around us.
The integration of more sophisticated models and real-time processing capabilities will enhance the accuracy and usability of speech recognition, making it a cornerstone of future human-computer interaction.
資料ダウンロード
QCD調達購買管理クラウド「newji」は、調達購買部門で必要なQCD管理全てを備えた、現場特化型兼クラウド型の今世紀最高の購買管理システムとなります。
ユーザー登録
調達購買業務の効率化だけでなく、システムを導入することで、コスト削減や製品・資材のステータス可視化のほか、属人化していた購買情報の共有化による内部不正防止や統制にも役立ちます。
NEWJI DX
製造業に特化したデジタルトランスフォーメーション(DX)の実現を目指す請負開発型のコンサルティングサービスです。AI、iPaaS、および先端の技術を駆使して、製造プロセスの効率化、業務効率化、チームワーク強化、コスト削減、品質向上を実現します。このサービスは、製造業の課題を深く理解し、それに対する最適なデジタルソリューションを提供することで、企業が持続的な成長とイノベーションを達成できるようサポートします。
オンライン講座
製造業、主に購買・調達部門にお勤めの方々に向けた情報を配信しております。
新任の方やベテランの方、管理職を対象とした幅広いコンテンツをご用意しております。
お問い合わせ
コストダウンが利益に直結する術だと理解していても、なかなか前に進めることができない状況。そんな時は、newjiのコストダウン自動化機能で大きく利益貢献しよう!
(Β版非公開)