- お役立ち記事
- Unsupervised and supervised pattern recognition
Unsupervised and supervised pattern recognition
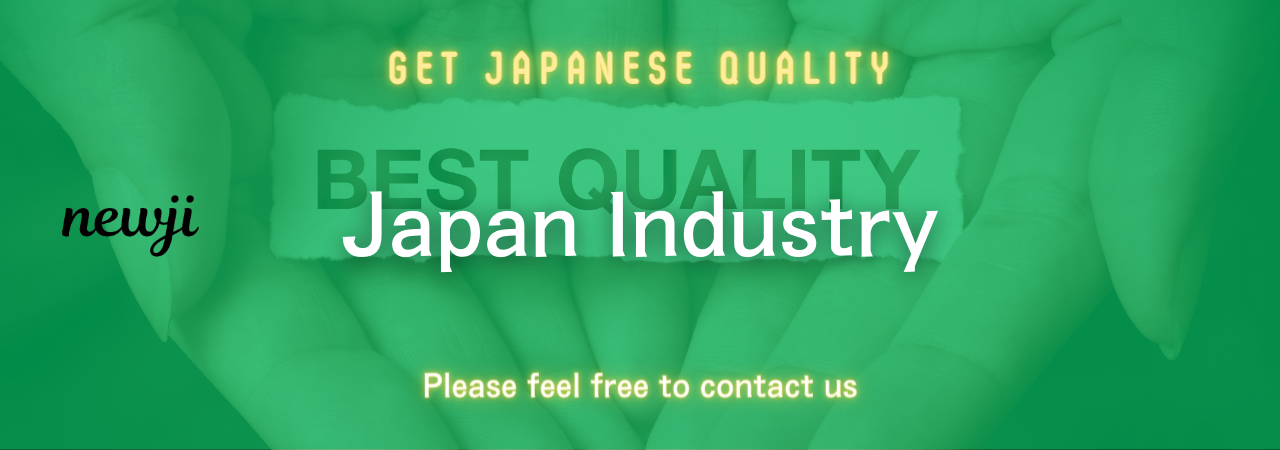
目次
Understanding Pattern Recognition
Pattern recognition is a crucial part of data analysis, a field that helps in identifying patterns in data and making sense of them.
It is an essential component of machine learning and artificial intelligence.
Within pattern recognition, there are two major types: unsupervised and supervised.
Understanding these types is crucial for anyone interested in harnessing the power of machine learning to interpret and utilize data effectively.
Supervised Pattern Recognition
What is Supervised Pattern Recognition?
Supervised pattern recognition involves training a system using a labeled dataset.
Each data point is tagged with the correct output, which helps the model to learn over time.
The primary goal here is to predict outputs based on input data after learning from the training phase.
How It Works
In supervised pattern recognition, data is split into two parts: the training set and the test set.
The model learns from the training set, adjusting its parameters to minimize error in predictions.
This involves iterating over the data, recognizing patterns, and making corrections based on the set labels.
Once trained, the model is tested on the test set, evaluating its ability to correctly predict outcomes for unseen data.
Performance is assessed through metrics such as accuracy, precision, recall, and F1 score, reflecting the model’s proficiency in recognizing patterns and making precise predictions.
Examples of Supervised Pattern Recognition
Supervised pattern recognition is widely used in various applications, such as:
– Speech recognition: Systems learn to differentiate between different sounds and words by training on labeled datasets of spoken language.
– Image classification: Models are trained to identify objects within an image or recognize people’s faces by learning from datasets with labeled examples.
– Sentiment analysis: Text data is labeled as positive, neutral, or negative to train systems that can analyze customers’ sentiments in reviews or social media posts.
– Fraud detection: With labeled samples of fraudulent and legitimate transaction data, models learn patterns indicating fraudulent activities to predict and prevent them.
Unsupervised Pattern Recognition
What is Unsupervised Pattern Recognition?
Unlike supervised pattern recognition, unsupervised pattern recognition involves working with data that has no predefined labels.
The goal here is to deduce patterns and structures from the data without any prior guidance.
This method is more exploratory and seeks to find hidden structures within the data.
How It Works
Unsupervised pattern recognition uses algorithms designed to identify patterns based on the inherent properties of the data.
These include clustering, dimensionality reduction, and association algorithms.
Clustering algorithms, for instance, group data points based on similarity, helping discover natural groupings or segments within the data.
Dimensionality reduction techniques, such as Principal Component Analysis (PCA), help simplify data by reducing its dimensions, making it easier to visualize and interpret.
Association algorithms are used to find rules that describe the relationships between data attributes.
The unsupervised approach provides insights into data structure, guiding further analysis and aiding decision-making, especially when dealing with vast sets of unlabelled data.
Examples of Unsupervised Pattern Recognition
Unsupervised pattern recognition finds application in various domains, including:
– Customer segmentation: Retailers use clustering techniques to segment customers based on purchasing behaviors and preferences, aiding in tailored marketing strategies.
– Anomaly detection: In network security, systems detect unusual patterns that could indicate a security breach or malfunction.
– Market basket analysis: Retailers analyze shopping patterns, identifying combinations of items frequently purchased together to optimize product placement and promotions.
– Document clustering: Large collections of text documents, such as articles and reports, can be grouped based on topics, enhancing information retrieval and organization.
The Differences and Overlaps Between Supervised and Unsupervised Pattern Recognition
Supervised pattern recognition requires labeled data and is focused on making predictions with high accuracy.
It is well-suited for scenarios where there is a clear output to predict based on input data.
On the other hand, unsupervised pattern recognition deals with unlabeled data, emphasizing pattern discovery and data structure understanding.
It is ideal for exploratory data analysis, where unexpected insights and patterns can emerge.
Despite their differences, these approaches can be complementary.
For example, unsupervised learning can help pre-process data by reducing noise and clustering data, providing a cleaner set of inputs for supervised learning models.
Hybrid approaches can also harness the strengths of both supervised and unsupervised learning.
Semi-supervised learning, for instance, involves a mix of labeled and unlabeled data, offering a balanced approach to utilising both paradigms.
Conclusion
Pattern recognition, both supervised and unsupervised, is a powerful tool in the realm of data analysis and machine learning.
Understanding these approaches and how they can be applied is vital for exploiting the full potential of data in various sectors.
As technology advances, the ability to discern patterns in data will continue to shape industries and innovations.
Whether predicting outcomes or uncovering hidden structures, mastering pattern recognition helps make informed decisions, opening up new possibilities in artificial intelligence and beyond.
資料ダウンロード
QCD調達購買管理クラウド「newji」は、調達購買部門で必要なQCD管理全てを備えた、現場特化型兼クラウド型の今世紀最高の購買管理システムとなります。
ユーザー登録
調達購買業務の効率化だけでなく、システムを導入することで、コスト削減や製品・資材のステータス可視化のほか、属人化していた購買情報の共有化による内部不正防止や統制にも役立ちます。
NEWJI DX
製造業に特化したデジタルトランスフォーメーション(DX)の実現を目指す請負開発型のコンサルティングサービスです。AI、iPaaS、および先端の技術を駆使して、製造プロセスの効率化、業務効率化、チームワーク強化、コスト削減、品質向上を実現します。このサービスは、製造業の課題を深く理解し、それに対する最適なデジタルソリューションを提供することで、企業が持続的な成長とイノベーションを達成できるようサポートします。
オンライン講座
製造業、主に購買・調達部門にお勤めの方々に向けた情報を配信しております。
新任の方やベテランの方、管理職を対象とした幅広いコンテンツをご用意しております。
お問い合わせ
コストダウンが利益に直結する術だと理解していても、なかなか前に進めることができない状況。そんな時は、newjiのコストダウン自動化機能で大きく利益貢献しよう!
(Β版非公開)