- お役立ち記事
- Concepts of Bayesian statistics and how to use data
Concepts of Bayesian statistics and how to use data
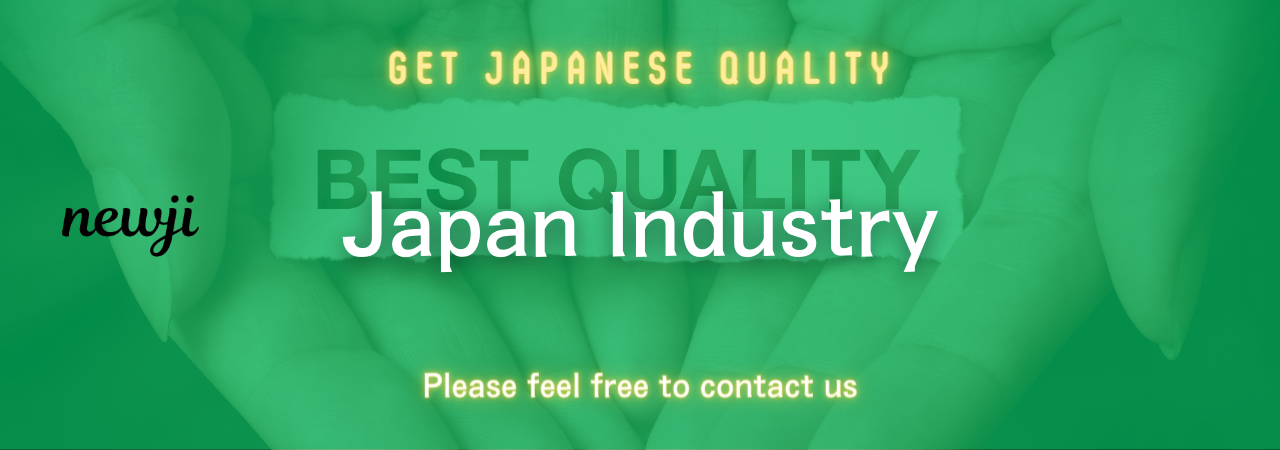
目次
Understanding Bayesian Statistics
Bayesian statistics is a fascinating approach to probability and data analysis that differs significantly from traditional frequentist statistics.
The central concept revolves around using probability to represent uncertainty in knowledge, which is updated as new evidence is introduced.
The focus isn’t on proving a hypothesis but on assessing the probability of various outcomes or hypotheses.
At its core, Bayesian statistics combines prior knowledge with current data to inform decision-making processes.
This is often represented through Bayes’ Theorem, a formula that describes how to update the probability estimate for a hypothesis as additional evidence is acquired.
Bayes’ Theorem: The Backbone
Bayes’ Theorem is the mathematical expression used to execute Bayesian inference.
It is articulated as:
P(H|E) = [P(E|H) * P(H)] / P(E)
Here, P(H|E) is the probability of the hypothesis H given the evidence E, known as the posterior probability.
P(E|H) is the likelihood of observing the evidence E given that hypothesis H is true.
P(H) is the prior probability, reflecting the initial belief about the hypothesis.
Finally, P(E) is the probability of observing the evidence under all possible hypotheses.
This theorem allows one to update their beliefs systematically by taking into account both the prior knowledge and the likelihood of new data.
The ability to continuously update probabilities with new data makes Bayesian statistics particularly powerful, especially in fields where evidence accumulates over time.
Priors and Posterior Distribution
Understanding priors is crucial in Bayesian statistics.
A prior represents your initial belief about a parameter before analyzing current data.
These beliefs could stem from past research, expert opinion, or even subjective judgment.
The posterior distribution is the updated belief after taking into account the new evidence.
It combines the prior and the likelihood to provide a more accurate and refined estimate of the probability of a hypothesis.
The choice of prior can significantly influence the results, especially when data is limited.
However, with ample data, the effect of the prior diminishes, allowing the data to speak more clearly.
Bayesian Inference in Action
The power of Bayesian inference lies in its flexibility and adaptability in diverse scenarios.
It is widely utilized in machine learning, medicine, finance, and more.
For instance, in medical diagnostics, Bayesian methods allow practitioners to update their confidence in a diagnosis as new test results become available.
If initial tests suggest a low probability of a disease but subsequent tests show positive results, the Bayesian approach adjusts the probability upwards based on this new evidence, leading to better-informed decisions.
In finance, Bayesian models help in asset pricing and risk assessment by updating predictions as economic indicators change.
Comparison with Frequentist Statistics
A fundamental difference between Bayesian and frequentist statistics is the interpretation of probability.
Frequentist statistics interpret probability as the long-run frequency of events, focusing on hypothesis testing and p-values.
In contrast, Bayesian statistics interpret probability as a degree of belief or certainty.
This leads to different approaches in solving statistical problems.
For instance, frequentist methods may reject a hypothesis based on a small p-value, while Bayesian methods estimate the probability of the hypothesis given the observed data.
Frequentist methods rely on fixed parameters, while Bayesian methods treat parameters as random variables, allowing more flexibility in modeling complex systems.
Using Bayesian Concepts with Data
Implementing Bayesian statistics with data involves a series of steps, typically including defining the model, selecting priors, computing the likelihood, and applying Bayes’ Theorem to obtain the posterior distribution.
Step 1: Model Specification
The first step is defining a statistical model that represents the problem.
This involves identifying the parameter of interest and how it relates to the observed data.
Step 2: Selecting Priors
Choosing priors is crucial, as they reflect the initial beliefs or knowledge about the parameter before analyzing the data.
Care must be taken to ensure that priors are not overly informative, especially in cases with limited data.
Step 3: Computing the Likelihood
The likelihood estimates the probability of the observed data under different parameter values.
It measures how well different parameter values explain the observed data.
Step 4: Applying Bayes’ Theorem
With the priors and likelihood computed, Bayes’ Theorem is used to calculate the posterior distribution.
This distribution reflects updated beliefs about the parameter after considering the data.
Step 5: Making Decisions
The posterior distribution provides critical insights into decision-making.
By considering potential outcomes and their probabilities, one can make informed decisions based on the level of uncertainty inherent in the data.
Challenges and Considerations
Despite its advantages, Bayesian statistics is not without challenges.
Computational complexity is a significant issue, especially with complex models and large datasets.
However, advances in computational techniques, such as Markov Chain Monte Carlo (MCMC) methods, have alleviated these concerns to some extent.
Another consideration is the subjective nature of priors, which can lead to different conclusions depending on initial beliefs.
This underscores the importance of transparent reasoning and careful justification of prior choices.
Conclusion
Bayesian statistics offers a robust framework for dealing with uncertainty and incorporating prior knowledge into data analysis.
Its ability to update beliefs with new evidence makes it an invaluable tool in various fields where decision-making is crucial.
Understanding its principles and how to implement them with data empowers analysts and researchers to make informed, evidence-based conclusions.
As computational methods continue to evolve, Bayesian statistics will likely play a more significant role in the future of data science, highlighting its relevance in an ever-changing world.
資料ダウンロード
QCD調達購買管理クラウド「newji」は、調達購買部門で必要なQCD管理全てを備えた、現場特化型兼クラウド型の今世紀最高の購買管理システムとなります。
ユーザー登録
調達購買業務の効率化だけでなく、システムを導入することで、コスト削減や製品・資材のステータス可視化のほか、属人化していた購買情報の共有化による内部不正防止や統制にも役立ちます。
NEWJI DX
製造業に特化したデジタルトランスフォーメーション(DX)の実現を目指す請負開発型のコンサルティングサービスです。AI、iPaaS、および先端の技術を駆使して、製造プロセスの効率化、業務効率化、チームワーク強化、コスト削減、品質向上を実現します。このサービスは、製造業の課題を深く理解し、それに対する最適なデジタルソリューションを提供することで、企業が持続的な成長とイノベーションを達成できるようサポートします。
オンライン講座
製造業、主に購買・調達部門にお勤めの方々に向けた情報を配信しております。
新任の方やベテランの方、管理職を対象とした幅広いコンテンツをご用意しております。
お問い合わせ
コストダウンが利益に直結する術だと理解していても、なかなか前に進めることができない状況。そんな時は、newjiのコストダウン自動化機能で大きく利益貢献しよう!
(Β版非公開)