- お役立ち記事
- Innovative experimental design with Multi-Sigma
Innovative experimental design with Multi-Sigma
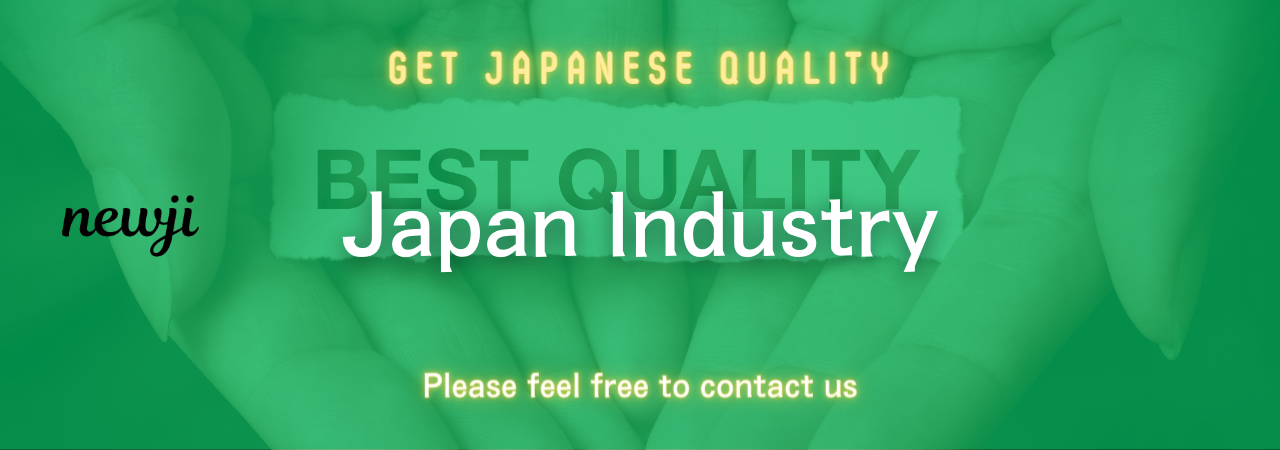
Understanding Multi-Sigma in Experimental Design
In the world of scientific research and development, designing experiments is a critical component that influences the overall success of any project.
A robust experimental design allows researchers to systematically investigate questions and derive meaningful insights.
One innovative methodology that is gaining attention in this field is the Multi-Sigma approach.
This approach enhances the precision and reliability of experimental results by incorporating multiple standard deviations, or “sigmas,” into the design process.
What is Multi-Sigma?
Multi-Sigma is a technique used in statistical analysis and experimental design to better understand variability and uncertainty in data.
In simple terms, “sigma” signifies a standard deviation, which measures the extent of variation in a dataset.
Applying multiple sigmas means considering several levels of variation while designing an experiment.
This method aims to minimize risks associated with random errors and biases, providing more precise conclusions.
The Importance of Experimental Design
Experimental design is a framework that outlines the procedures for conducting experiments.
It is essential for ensuring that an experiment is executed effectively and can be replicated with consistency.
A sound design reduces errors, improves data accuracy, and ensures the reliability of results.
Incorporating Multi-Sigma into this process further strengthens the design by addressing all potential sources of variability.
Advantages of Multi-Sigma Design
One primary advantage of the Multi-Sigma approach is its ability to improve the robustness of experimental results.
By accounting for different levels of variability, researchers can better understand the impact of various factors on their findings.
This leads to more reliable and generalizable results that are less susceptible to random noise and uncertain conditions.
Additionally, Multi-Sigma helps identify critical variables that significantly influence outcomes, allowing researchers to focus their efforts effectively.
Implementing Multi-Sigma in Your Experiments
To incorporate Multi-Sigma into your experimental design, begin by identifying key variables and parameters that might affect your results.
Understand the typical range of variability for each and determine the necessary number of sigmas needed to cover potential deviations.
Once defined, incorporate these sigmas into the design of your trials and ensure your data collection methods are capable of capturing this breadth of variation.
Visualization is also an important aspect of implementation.
Look for ways to graphically represent your data with the appropriate sigmas, such as standard deviation curves, to help visualize the extent of variability in your dataset.
This visual evidence can support findings and illustrate the level of uncertainty present in the results.
Potential Challenges and How to Overcome Them
While Multi-Sigma provides a strong foundation for experimental accuracy, it’s not without challenges.
Calculating and integrating multiple sigmas can become complex and require advanced statistical knowledge.
This complexity can be addressed by utilizing software tools designed to manage statistical analyses or by consulting with a statistician to ensure correct application.
Additionally, experiments involving numerous variables might require larger sample sizes to accurately capture the full scope of variation.
Planning for this in the initial stages of design, and allocating sufficient resources, is critical for successful implementation.
Case Studies and Real-World Applications
Several industries and fields have begun employing Multi-Sigma to enhance the quality of their experimental outcomes.
In pharmaceuticals, for instance, researchers use this technique to control for variability in drug response among different populations, thereby strengthening clinical trial conclusions.
The manufacturing sector applies Multi-Sigma to improve product testing reliability, ensuring quality control measures overcome production variances.
Through these diverse applications, it becomes evident that Multi-Sigma offers a flexible tool applicable to a variety of experimental scenarios.
Future of Experimental Designs with Multi-Sigma
As technology progresses, the potential for Multi-Sigma to shape experimental designs is significant.
As more researchers familiarize themselves with this approach, its integration into standard experimental protocols will likely increase.
The development of dedicated software tools that simplify complex statistical calculations will facilitate its wider adoption, broadening its accessibility.
Moreover, as industries continue to seek more reliable data to inform decisions, the demand for robust experimental design methods like Multi-Sigma will grow.
This trend predicts a promising future where research efficiency meets the accuracy in diverse fields of study.
Conclusion
Adopting an innovative approach like Multi-Sigma in experimental design can elevate the quality and reliability of research findings.
It enables researchers to navigate and mitigate variability in their experiments, ensuring robust and trustworthy results.
As this methodology gains traction, more industries will benefit from its application, ultimately advancing scientific knowledge and innovation across various disciplines.
資料ダウンロード
QCD調達購買管理クラウド「newji」は、調達購買部門で必要なQCD管理全てを備えた、現場特化型兼クラウド型の今世紀最高の購買管理システムとなります。
ユーザー登録
調達購買業務の効率化だけでなく、システムを導入することで、コスト削減や製品・資材のステータス可視化のほか、属人化していた購買情報の共有化による内部不正防止や統制にも役立ちます。
NEWJI DX
製造業に特化したデジタルトランスフォーメーション(DX)の実現を目指す請負開発型のコンサルティングサービスです。AI、iPaaS、および先端の技術を駆使して、製造プロセスの効率化、業務効率化、チームワーク強化、コスト削減、品質向上を実現します。このサービスは、製造業の課題を深く理解し、それに対する最適なデジタルソリューションを提供することで、企業が持続的な成長とイノベーションを達成できるようサポートします。
オンライン講座
製造業、主に購買・調達部門にお勤めの方々に向けた情報を配信しております。
新任の方やベテランの方、管理職を対象とした幅広いコンテンツをご用意しております。
お問い合わせ
コストダウンが利益に直結する術だと理解していても、なかなか前に進めることができない状況。そんな時は、newjiのコストダウン自動化機能で大きく利益貢献しよう!
(Β版非公開)