- お役立ち記事
- Fundamentals of MT systems and their use in discrimination/abnormality detection and their key points
Fundamentals of MT systems and their use in discrimination/abnormality detection and their key points
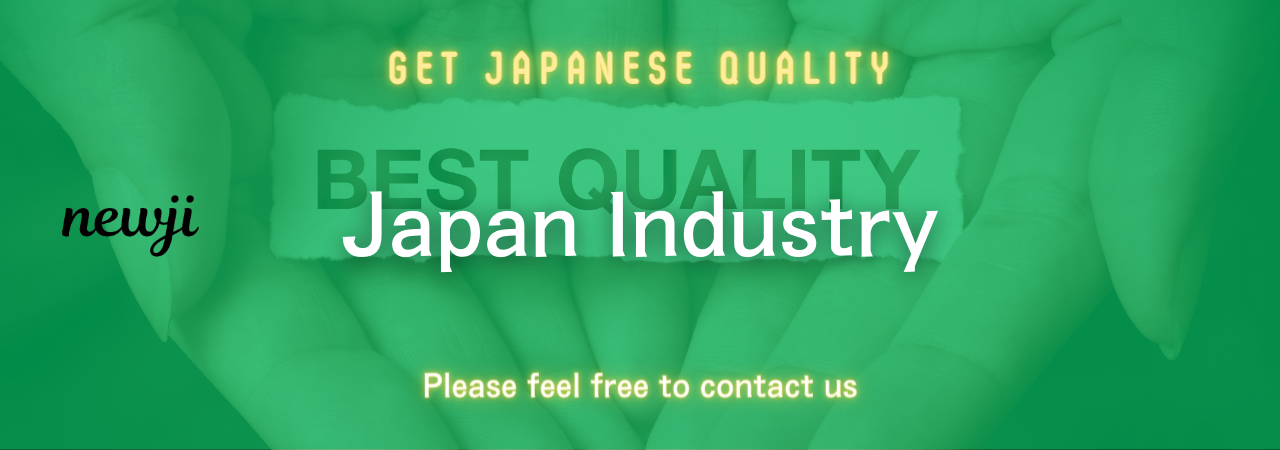
目次
Introduction to Machine Translation Systems
Machine Translation (MT) systems have revolutionized the way we communicate across different languages.
These systems are designed to automatically convert text from one language to another, leveraging advanced algorithms and models.
With globalization on the rise, the importance of efficient and accurate translation processes cannot be overstated.
Initially, MT systems were rule-based, relying on linguistic rules and dictionaries to render translations.
However, as technology progressed, statistical methods and, more recently, neural network models have taken center stage.
These advancements have improved the accuracy and fluency of translations, making MT systems more reliable and easier to use.
Types of Machine Translation Systems
MT systems can broadly be categorized into three main types:
1. Rule-Based Machine Translation (RBMT)
RBMT relies on linguistic rules and grammar expertise to translate text.
Developers create a comprehensive set of grammar rules and a bilingual dictionary that the system uses to process translations.
While this approach ensures grammatical accuracy, it often struggles with idiomatic expressions and contextual nuances.
2. Statistical Machine Translation (SMT)
SMT systems utilize statistical methods to determine the most likely translation of a given text.
By analyzing large corpora of bilingual text, these systems calculate probabilities and use them to make translation decisions.
Although more flexible than RBMT, SMT may still struggle with context and idiomatic language.
3. Neural Machine Translation (NMT)
NMT is the latest innovation in MT systems, employing deep learning techniques to translate text.
By using neural networks, these systems can better understand context, nuance, and idiomatic expressions, resulting in more natural translations.
NMT systems learn from vast amounts of data and continuously improve as they process more information.
Applications in Discrimination and Abnormality Detection
Beyond conventional translation tasks, MT systems are increasingly used in discrimination and abnormality detection.
In these contexts, MT systems help identify biased language, inappropriate content, or unusual patterns in text data.
Discrimination Detection
One powerful application is the detection of discriminatory language or bias in written content.
Organizations can deploy MT systems to monitor communication channels, ensuring that language used is inclusive and non-discriminatory.
By analyzing sentiment and context, MT systems flag potentially biased expressions for further review.
For instance, companies can use MT systems to evaluate customer service interactions or internal communications to ensure compliance with diversity and inclusion standards.
Such use enhances organizational culture and protects against reputational damage.
Abnormality Detection
MT systems are also valuable in detecting abnormalities in text data, which is crucial in fields like cybersecurity and finance.
By processing vast amounts of text data, MT systems can identify patterns and flag anomalies that may suggest security breaches or fraudulent activity.
For example, in financial sectors, MT systems can analyze transaction logs written in various languages to detect suspect behavior, such as money laundering activities.
Similarly, in cybersecurity, MT systems can scrutinize network communications to pinpoint phishing attempts and unauthorized data exfiltration.
Key Points for Effective MT System Use
While MT systems offer numerous advantages, it is vital to employ them effectively to harness their full potential.
Here are some key points to consider:
1. Choosing the Right Type
The selection of a specific MT system type should align with organizational needs.
For simple translation tasks, RBMT may suffice.
However, for nuanced and context-heavy translations, NMT is preferable due to its ability to learn and adapt.
2. Training and Data Quality
The performance of MT systems largely depends on the quality and quantity of data they are trained on.
Providing comprehensive and high-quality datasets is crucial for accurate translations and effective bias detection.
3. Continuous Learning and Updates
As language evolves, MT systems must be updated to incorporate new linguistic patterns and expressions.
Continuous learning from recent data allows the MT system to improve its accuracy and relevance over time.
4. Integration and Feedback
Integrating MT systems with existing workflows and tools enables seamless operation and maximizes their utility.
Additionally, feedback mechanisms should be implemented so that users can report translation errors or biases, contributing to system refinement.
Conclusion
MT systems have transformed from simplistic language converters into sophisticated tools capable of contextually accurate translations and valuable insights in discrimination and abnormality detection.
By understanding their types, applications, and key considerations for effective use, organizations can harness their power not only for global communication but also for enhancing operational security and maintaining ethical standards.
As technology continues to advance, the scope and efficacy of MT systems will undoubtedly expand, opening new avenues for use across various sectors.
資料ダウンロード
QCD調達購買管理クラウド「newji」は、調達購買部門で必要なQCD管理全てを備えた、現場特化型兼クラウド型の今世紀最高の購買管理システムとなります。
ユーザー登録
調達購買業務の効率化だけでなく、システムを導入することで、コスト削減や製品・資材のステータス可視化のほか、属人化していた購買情報の共有化による内部不正防止や統制にも役立ちます。
NEWJI DX
製造業に特化したデジタルトランスフォーメーション(DX)の実現を目指す請負開発型のコンサルティングサービスです。AI、iPaaS、および先端の技術を駆使して、製造プロセスの効率化、業務効率化、チームワーク強化、コスト削減、品質向上を実現します。このサービスは、製造業の課題を深く理解し、それに対する最適なデジタルソリューションを提供することで、企業が持続的な成長とイノベーションを達成できるようサポートします。
オンライン講座
製造業、主に購買・調達部門にお勤めの方々に向けた情報を配信しております。
新任の方やベテランの方、管理職を対象とした幅広いコンテンツをご用意しております。
お問い合わせ
コストダウンが利益に直結する術だと理解していても、なかなか前に進めることができない状況。そんな時は、newjiのコストダウン自動化機能で大きく利益貢献しよう!
(Β版非公開)