- お役立ち記事
- Fundamentals of AI control modeling and practical application to building control models
Fundamentals of AI control modeling and practical application to building control models
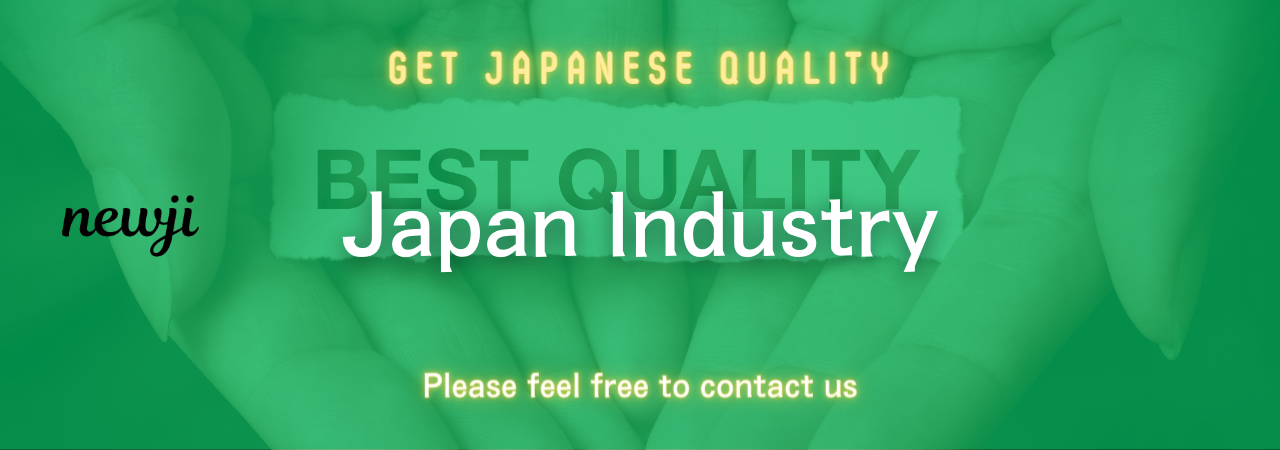
目次
Understanding AI Control Models
Artificial Intelligence (AI) is significantly transforming various sectors, with control systems being one of the prominent areas witnessing this change.
AI control models are at the forefront of this transformation, providing innovative solutions that enhance the efficiency and performance of control systems.
In simple terms, AI control models are algorithms or systems designed to manage and control processes, machines, or operations autonomously, using intelligent behavior similar to human capabilities.
Importance of AI in Control Systems
The integration of AI into control systems brings about numerous benefits.
It allows for the automation of complex tasks, reducing human intervention and error.
AI control models can process vast amounts of data in real-time, making quick decisions based on the insights derived from the data.
This ability significantly increases the precision and reliability of control systems, making them more efficient and effective.
Moreover, AI control models can learn and adapt over time.
This means they can improve their performance by learning from past experiences, which is a significant departure from traditional control systems that rely on pre-set rules and cannot adapt to changing conditions.
Building AI Control Models
Data Collection and Preprocessing
The first step in building an AI control model is data collection.
This is a critical step as the model’s performance heavily relies on the quality and quantity of data it is trained on.
Data can be collected from sensors, databases, or real-time data streams, depending on the specific application.
Once the data is collected, it needs to be preprocessed to ensure it is clean and free from noise.
Data preprocessing involves tasks such as data cleaning, normalization, transformation, and reduction.
Model Selection and Training
After data preprocessing, the next step is model selection.
There are various types of AI models available, such as neural networks, decision trees, and reinforcement learning models.
The choice of model depends on the nature of the control task, the complexity of the system, and the availability of data.
Once a model is selected, it is trained using the preprocessed data.
The training process involves feeding the data into the model and adjusting its parameters to minimize the error in its predictions.
Model Evaluation and Implementation
After training, the model needs to be evaluated to ensure it performs well on unseen data.
This is done by testing the model on a separate dataset, known as the validation set.
Various metrics, such as accuracy, precision, and recall, are used to evaluate the model’s performance.
If the model meets the desired performance criteria, it can be implemented in the real-world system.
Implementation involves integrating the model with the existing control system and ensuring it functions correctly in the live environment.
Practical Applications of AI Control Models
AI control models have a wide range of applications across different industries.
Here are some practical applications:
Smart Buildings
In the context of building control, AI is being used to create smart buildings.
AI control models can manage heating, ventilation, and air conditioning (HVAC) systems to maintain optimal indoor climates while minimizing energy consumption.
They can also control lighting and security systems, enhancing both comfort and safety in buildings.
Manufacturing
In manufacturing, AI control models are used to control robotic arms, optimize production lines, and manage supply chains.
They can predict equipment failures through predictive maintenance, reducing downtime and saving costs.
AI can also make real-time adjustments to improve product quality and manufacturing efficiency.
Transportation
AI control models are pivotal in the development of autonomous vehicles and smart traffic management systems.
They enable vehicles to navigate safely by processing real-time data from sensors and making split-second decisions.
In traffic management, AI helps optimize traffic flow, reduce congestion, and improve safety on the roads.
Challenges and Future Prospects
Despite the numerous benefits, there are challenges associated with AI control models.
One major challenge is ensuring the security and reliability of AI systems, as they are susceptible to cyberattacks.
Another challenge is the ethical considerations of AI decisions, especially in safety-critical applications.
Moreover, the high cost and complexity of implementing AI systems can be prohibitive for some organizations.
Looking to the future, AI control models are expected to become more sophisticated and widespread.
Advancements in AI technology, coupled with increasing data availability, will drive innovation in control systems.
We can anticipate more intelligent, adaptable, and autonomous control solutions that will continue to transform various industries.
In conclusion, AI control models are revolutionizing the way control systems are designed and operated.
Their ability to learn, adapt, and make decisions autonomously holds the potential to significantly enhance efficiency, performance, and safety across different applications.
As technology evolves, so too will the capabilities and applications of AI control models, paving the way for more advanced and intelligent systems.
資料ダウンロード
QCD調達購買管理クラウド「newji」は、調達購買部門で必要なQCD管理全てを備えた、現場特化型兼クラウド型の今世紀最高の購買管理システムとなります。
ユーザー登録
調達購買業務の効率化だけでなく、システムを導入することで、コスト削減や製品・資材のステータス可視化のほか、属人化していた購買情報の共有化による内部不正防止や統制にも役立ちます。
NEWJI DX
製造業に特化したデジタルトランスフォーメーション(DX)の実現を目指す請負開発型のコンサルティングサービスです。AI、iPaaS、および先端の技術を駆使して、製造プロセスの効率化、業務効率化、チームワーク強化、コスト削減、品質向上を実現します。このサービスは、製造業の課題を深く理解し、それに対する最適なデジタルソリューションを提供することで、企業が持続的な成長とイノベーションを達成できるようサポートします。
オンライン講座
製造業、主に購買・調達部門にお勤めの方々に向けた情報を配信しております。
新任の方やベテランの方、管理職を対象とした幅広いコンテンツをご用意しております。
お問い合わせ
コストダウンが利益に直結する術だと理解していても、なかなか前に進めることができない状況。そんな時は、newjiのコストダウン自動化機能で大きく利益貢献しよう!
(Β版非公開)