- お役立ち記事
- Self-localization in dynamic environments
Self-localization in dynamic environments
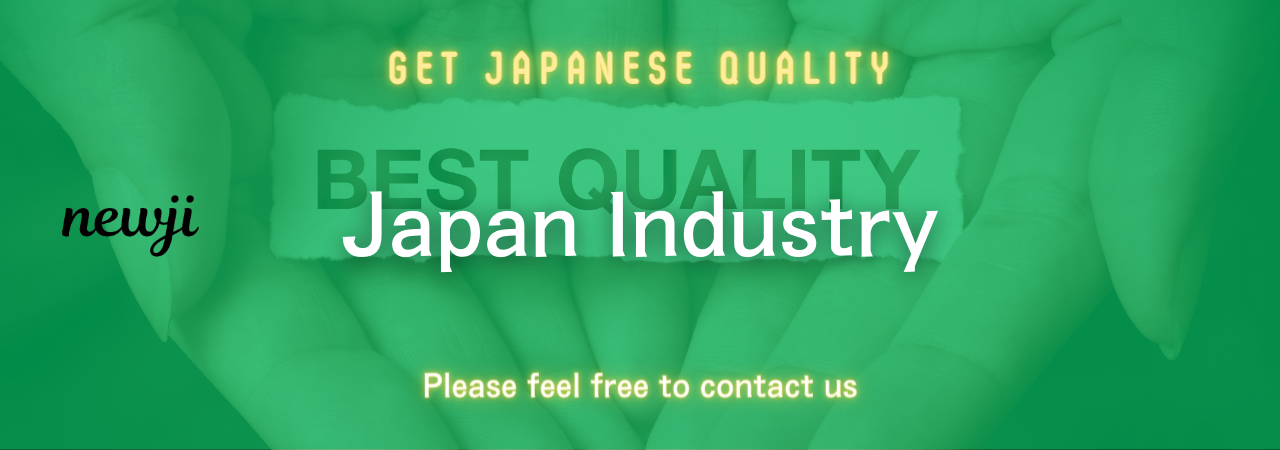
目次
Understanding Self-Localization
Self-localization refers to the ability of a system, typically a robot, to determine its own position within a given environment.
This capability is crucial for a wide range of applications, such as autonomous vehicles, robotic vacuum cleaners, and drones.
In simple terms, self-localization is comparable to how humans use landmarks to recognize their current location and navigate through space.
An effective self-localization system enhances autonomy by enabling a robot to make informed decisions and navigate efficiently.
Importance of Dynamic Environments
Dynamic environments are spaces where changes occur frequently and unpredictably, such as crowded streets or cluttered rooms.
In these scenarios, elements of the environment, like people and other objects, move around constantly.
For robots to function effectively in such environments, they must be able to adjust quickly and accurately to ongoing changes.
A robust self-localization system in a dynamic setting ensures that a robot can continually update its understanding of its surroundings, fostering seamless navigation and task execution.
Challenges in Self-Localization
Self-localization in dynamic environments presents unique challenges.
One of the main issues is accounting for the unpredictability of the surroundings.
Objects or individuals might unexpectedly obscure landmarks or alter paths.
Furthermore, sensory data collected might be noisy or incomplete, leading to potential inaccuracies in position estimation.
Another challenge is the sheer complexity and computation requirements needed to process real-time data.
Robots must be equipped with algorithms that can rapidly process large volumes of data to ensure they are reacting appropriately to changes.
Building robust models that can adaptively learn and improve over time is also a significant challenge.
Sensors and Technologies
Several sensors and technologies are integral to effective self-localization.
Lidar sensors, known for their precision, use laser light to project beams and measure distances, creating detailed maps of the environment.
Cameras serve as another essential tool, helping robots detect and recognize landmarks and objects, much like humans use their eyes.
Inertial measurement units (IMUs) provide data on acceleration and orientation, essential for understanding movement and position changes.
Combining these sensors can improve the accuracy and reliability of self-localization systems.
Algorithms That Make It Possible
To navigate dynamic environments effectively, robots rely on a variety of algorithms for self-localization.
Simultaneous Localization and Mapping (SLAM) stands as one of the most important techniques.
SLAM enables robots to build a map of unknown environments while keeping track of their location within that map.
This dual functionality is crucial for dynamic settings, where constant changes must be integrated into the map.
The Kalman filter is another common algorithm used in self-localization.
It helps refine position estimates by predicting future states and correcting errors based on current measurements.
Particle filters, on the other hand, use a probabilistic approach, maintaining several hypotheses about a robot’s position and refining them over time.
Applications in Everyday Life
Self-localization isn’t just a technical concept – it’s being effectively implemented in many aspects of daily life.
For instance, self-driving cars rely heavily on accurate self-localization to ensure safe navigation on roads filled with moving vehicles and pedestrians.
Robotic vacuum cleaners in homes use self-localization to efficiently navigate around furniture and ensure thorough cleaning.
Drones used for tasks like delivery or aerial photography also depend on self-localization to maintain stable flight paths and reach specific targets.
This technology opens the door to safer, more efficient operations in various fields.
Future Prospects and Developments
As technology advances, the capabilities of self-localization systems in dynamic environments continue to expand.
The integration of artificial intelligence and machine learning holds promise for even more adaptive and precise localization systems.
These technologies allow robots to learn from experience, improving their accuracy and effectiveness over time.
Additionally, advancements in sensor technology are anticipated to provide even more detailed and reliable environmental data.
Developing models that can process and make sense of this data efficiently will be a key focus area.
Conclusion
Self-localization in dynamic environments is a critical component in the development of autonomous systems.
By allowing robots to accurately determine and maintain their positions, they can effectively navigate complex and changing environments.
Through the use of advanced sensors, algorithms like SLAM, and the application of AI technologies, self-localization continues to improve, opening up new possibilities for innovation and practicality in everyday life.
As research and development in this field progress, the reliability and efficiency of self-localization systems will likely enhance, driving the future of robotics and autonomous technology forward.
資料ダウンロード
QCD調達購買管理クラウド「newji」は、調達購買部門で必要なQCD管理全てを備えた、現場特化型兼クラウド型の今世紀最高の購買管理システムとなります。
ユーザー登録
調達購買業務の効率化だけでなく、システムを導入することで、コスト削減や製品・資材のステータス可視化のほか、属人化していた購買情報の共有化による内部不正防止や統制にも役立ちます。
NEWJI DX
製造業に特化したデジタルトランスフォーメーション(DX)の実現を目指す請負開発型のコンサルティングサービスです。AI、iPaaS、および先端の技術を駆使して、製造プロセスの効率化、業務効率化、チームワーク強化、コスト削減、品質向上を実現します。このサービスは、製造業の課題を深く理解し、それに対する最適なデジタルソリューションを提供することで、企業が持続的な成長とイノベーションを達成できるようサポートします。
オンライン講座
製造業、主に購買・調達部門にお勤めの方々に向けた情報を配信しております。
新任の方やベテランの方、管理職を対象とした幅広いコンテンツをご用意しております。
お問い合わせ
コストダウンが利益に直結する術だと理解していても、なかなか前に進めることができない状況。そんな時は、newjiのコストダウン自動化機能で大きく利益貢献しよう!
(Β版非公開)