- お役立ち記事
- Fundamentals of emotional evaluation data analysis, key points of quantification and digitization, and its practice ~Demo included~
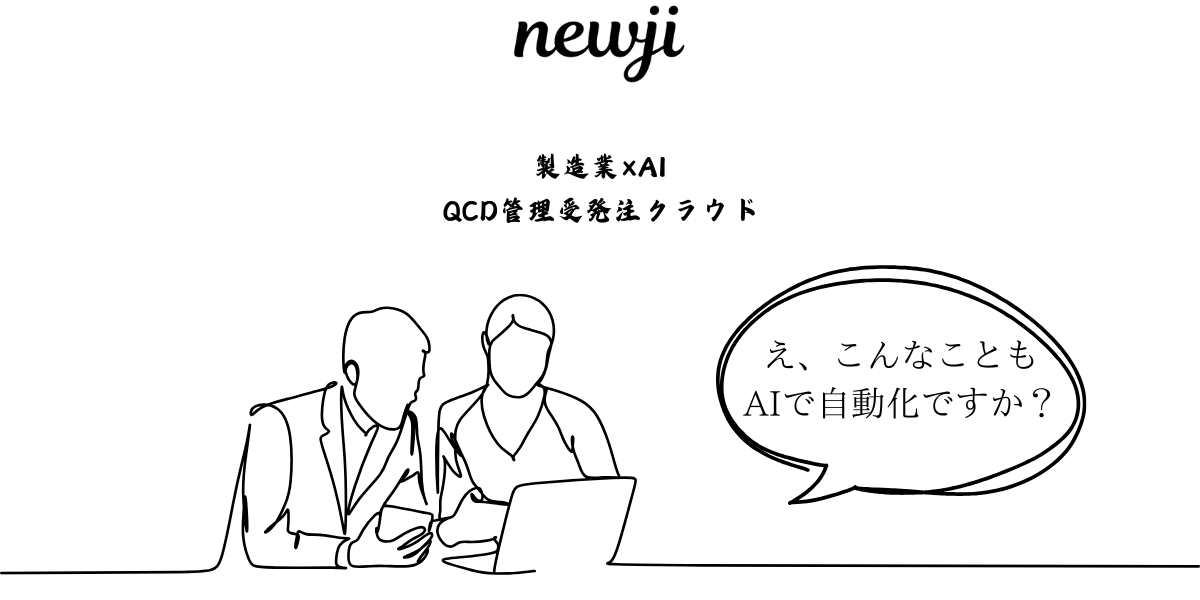
Fundamentals of emotional evaluation data analysis, key points of quantification and digitization, and its practice ~Demo included~
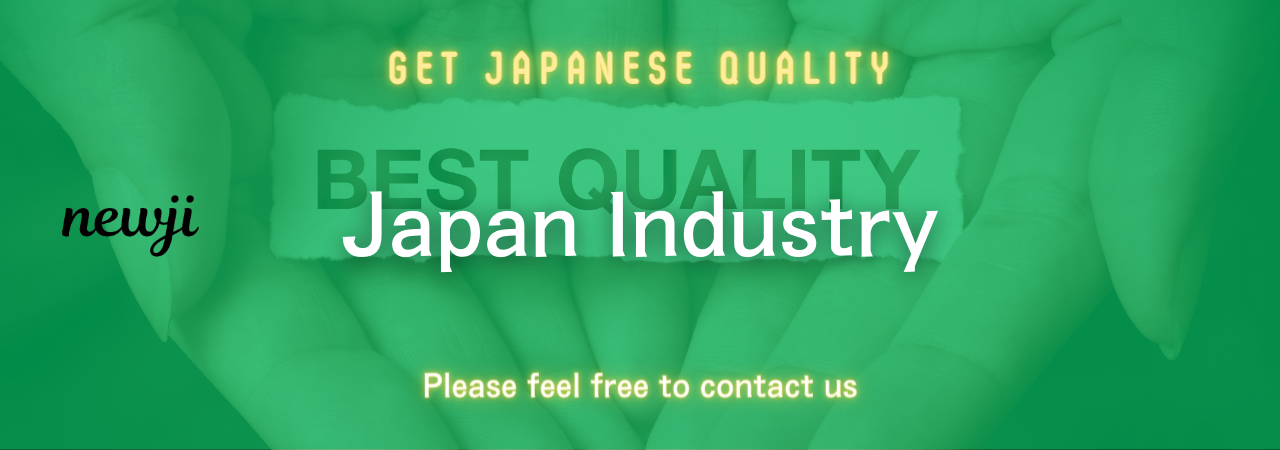
目次
Introduction to Emotional Evaluation Data Analysis
Emotional evaluation data analysis is an emerging field that merges the realms of psychology and data science to understand and predict human emotions.
With advancements in technology, it’s now possible to quantify and digitize emotional responses.
This technique can be crucial for various industries such as marketing, product development, and mental health.
Why Emotional Evaluation Matters
Understanding emotions goes beyond addressing human needs— it also plays a pivotal role in enhancing customer satisfaction, product engagement, and user experience.
Companies leverage emotional evaluation to create products and services that resonate deeply with their target audience.
For instance, emotive data can be used to tailor marketing strategies and improve storytelling elements in digital content.
By integrating emotional data, decision-makers can foster stronger connections and insights into consumer behavior.
Key Points of Quantification and Digitization
Identifying Emotional Data Sources
To effectively quantify emotional responses, one must first identify reliable data sources.
Traditional methods include interviews, surveys, and focus group discussions.
However, the digital age allows access to more dynamic sources such as social media analytics, wearable devices, and biometric feedback sensors.
Biometric sensors can measure physiological reactions like heart rate, pupil dilation, or skin conductivity to infer emotional states.
Data Collection and Pre-processing
Once the sources are identified, the next step is collecting and pre-processing data.
It’s essential to ensure data quality and relevance through processes like cleaning and sorting.
Removing irrelevant data, correcting errors, and standardizing information form a solid foundation for analysis.
Also, understanding the context of data is crucial since emotions can fluctuate widely based on variables like time, environment, and social interactions.
Quantification Methods
Two popular approaches to quantifying emotions are self-report scales and computational models.
Self-report scales, such as the Likert scale, involve individuals assessing their emotions directly.
Conversely, computational models employ algorithms to evaluate emotional cues from datasets.
Machine learning techniques, such as sentiment analysis and natural language processing (NLP), can categorize emotions by processing text, voice, or facial expressions.
Digitization Techniques
Digitization converts analog emotion data into a digital format that can be processed by computers.
For example, voice sentiment analysis uses audio data to extract emotional cues like tone and pitch.
In facial recognition, advanced software reads micro-expressions to identify emotions.
Once digitized, emotional data is ready for deeper analysis, allowing researchers to uncover patterns and trends.
Practical Applications of Emotional Data Analysis
Marketing and Product Development
In marketing, understanding consumer emotions can significantly enhance engagement strategies.
By analyzing customers’ emotional responses to advertisements, marketers can tailor content to evoke desired feelings.
Additionally, product developers use emotive feedback during prototyping phases to improve user interface and functionality.
Customer-centric models based on emotional data are more likely to result in innovative and successful products.
Mental Health and Well-being
Emotional analysis can also play a transformative role in mental health care.
By monitoring changes in emotional patterns, therapists can better understand and address psychological conditions.
Digital tools like mood-tracking apps provide users with insights into their emotional well-being over time.
This empowerment encourages proactive emotional management and supports mental health professionals in delivering personalized care.
Human-Computer Interaction (HCI)
Enhancing HCI through emotional evaluation creates more responsive and adaptive systems.
Smart assistants and interactive devices use emotive data to refine user interactions.
For example, an emotionally aware virtual assistant can adjust its responses to match the user’s mood, improving customer satisfaction.
In gaming, analyzing emotional responses can tailor experiences to extend engagement and enjoyment.
Practice: Conducting a Demo Analysis
Let’s conduct a quick demo analysis to illustrate emotional evaluation in practice.
Selecting a Dataset
Choose a dataset that provides a rich source of emotional data.
For instance, opt for tweets, product reviews, or digital focus group transcripts.
Social media platforms are particularly valuable due to their emotional expressions using text, emojis, and images.
Pre-processing the Data
Clean the dataset by removing noise and irrelevant elements.
Standardize text by addressing spelling errors, transforming slang, and eliminating special characters.
This preparation helps refine the accuracy of subsequent analysis phases.
Applying Analytic Tools
Utilize sentiment analysis tools and NLP libraries to classify and quantify emotions within the dataset.
For a more sophisticated approach, consider machine learning techniques that adaptively improve through iterative learning.
Key metrics to consider include sentiment polarity (positive, negative, neutral) and intensity levels (mild, moderate, strong).
Interpreting the Results
Finally, interpret the findings to identify trends or insights.
Look for patterns that reveal how emotions correlate with specific topics or events.
This analysis provides actionable insights, guiding decision-making and strategy formulation.
In conclusion, emotional evaluation data analysis holds the potential to revolutionize our understanding of human experiences.
By quantifying and digitizing emotions, industries can harness this knowledge to drive innovation, improve services, and enhance overall well-being.
From marketing to mental health, the application of emotional data offers profound opportunities across diverse fields.
資料ダウンロード
QCD調達購買管理クラウド「newji」は、調達購買部門で必要なQCD管理全てを備えた、現場特化型兼クラウド型の今世紀最高の購買管理システムとなります。
ユーザー登録
調達購買業務の効率化だけでなく、システムを導入することで、コスト削減や製品・資材のステータス可視化のほか、属人化していた購買情報の共有化による内部不正防止や統制にも役立ちます。
NEWJI DX
製造業に特化したデジタルトランスフォーメーション(DX)の実現を目指す請負開発型のコンサルティングサービスです。AI、iPaaS、および先端の技術を駆使して、製造プロセスの効率化、業務効率化、チームワーク強化、コスト削減、品質向上を実現します。このサービスは、製造業の課題を深く理解し、それに対する最適なデジタルソリューションを提供することで、企業が持続的な成長とイノベーションを達成できるようサポートします。
オンライン講座
製造業、主に購買・調達部門にお勤めの方々に向けた情報を配信しております。
新任の方やベテランの方、管理職を対象とした幅広いコンテンツをご用意しております。
お問い合わせ
コストダウンが利益に直結する術だと理解していても、なかなか前に進めることができない状況。そんな時は、newjiのコストダウン自動化機能で大きく利益貢献しよう!
(Β版非公開)