- お役立ち記事
- Fundamentals of multimodal information processing
Fundamentals of multimodal information processing
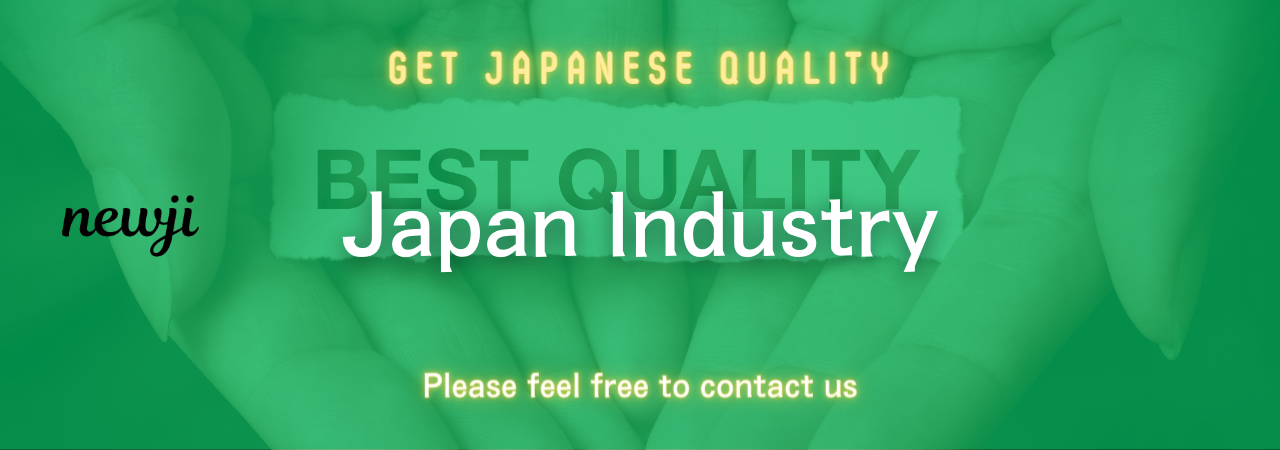
Understanding Multimodal Information Processing
Multimodal information processing is an exciting field that combines multiple types of data to enhance understanding and decision-making.
This approach utilizes diverse sources of information, such as text, images, audio, and video, to produce richer and more precise insights compared to using a single modality.
The integration of these data types mimics human sensory processing, which naturally combines inputs from our various senses to form a comprehensive understanding of our surroundings.
The Importance of Multimodal Information Processing
One crucial aspect of multimodal information processing is its ability to provide a more holistic view.
By leveraging different types of data, systems can understand context more deeply and accurately.
For instance, a video clip contains both visual and auditory information.
Analyzing these elements together rather than separately enhances the ability to interpret the scene more accurately, such as identifying emotions or actions.
Multimodal processing is integral in various technologies, including virtual assistants, autonomous vehicles, and medical imaging.
In each of these applications, different types of inputs need to be processed simultaneously to ensure the output is accurate and reliable.
Applications in Everyday Technology
Multimodal information processing is already a critical component of many technologies we use daily.
Virtual assistants like Siri and Alexa use voice recognition (audio) and context from previous queries (text) to provide users with relevant responses.
These systems learn continually to improve how effectively they understand and cater to individual user needs by integrating multiple data sources.
In the field of autonomous vehicles, multimodal processing is essential for safety and functionality.
These vehicles rely heavily on various sensors, such as cameras, radar, and LiDAR (Light Detection and Ranging) systems, to interpret the environment.
Combining these inputs ensures that vehicles can detect obstacles, interpret traffic signs, and navigate safely, even in complex or unpredictable traffic conditions.
In medical settings, the integration of different types of data can significantly enhance diagnostic accuracy.
For example, radiologists use a combination of different imaging modalities, such as X-rays, MRIs, and CT scans, to obtain a comprehensive view of a patient’s health.
Processing these images together provides a more accurate diagnosis than considering each one separately.
Challenges in Multimodal Information Processing
Despite its numerous advantages, multimodal information processing presents certain challenges.
One of the primary difficulties is data integration from multiple sources, which can vary in nature and format.
Ensuring compatibility and cohesiveness often requires sophisticated algorithms to synchronize and interpret data accurately.
Another challenge is the computational demand of processing multiple data types simultaneously.
Multimodal systems must handle a significant amount of data efficiently, which requires robust computational power and optimized algorithms.
Balancing accuracy and efficiency is crucial for the practical application of these systems.
Additionally, noise and ambiguities in data from different modalities can lead to incorrect interpretations.
Ensuring that the system can filter out irrelevant noise and comprehend nuanced information is vital for reliable performance.
Key Techniques in Multimodal Processing
To overcome these challenges, several techniques are utilized in multimodal information processing.
Feature extraction is a fundamental step where relevant data attributes are identified across all modalities.
This process aids in reducing the complexity and volume of data to be analyzed while highlighting critical aspects for decision-making.
Fusion strategies are also crucial, bringing together different data forms effectively.
Early fusion combines data from multiple modalities at the feature level, allowing integrated analysis from the start.
Late fusion, on the other hand, processes each modality separately and then combines the results, ensuring that each data type is evaluated independently before integration.
Deep learning has revolutionized multimodal processing by providing powerful tools for data integration and interpretation.
Neural networks, especially convolutional neural networks (CNNs) and recurrent neural networks (RNNs), are instrumental in recognizing patterns and correlations across different data types.
These models are trained to understand complex relationships within multimodal datasets, becoming more adept over time.
The Future of Multimodal Information Processing
The future of multimodal information processing is vibrant and full of possibilities.
As technology advances, we can anticipate even more sophisticated systems capable of understanding complex scenarios with improved accuracy.
Emerging technologies, such as augmented reality (AR) and virtual reality (VR), stand to benefit significantly from enhancements in multimodal information processing, offering more immersive and interactive experiences.
Furthermore, advancements in edge computing and artificial intelligence will contribute to more seamless integration of multimodal systems into everyday applications.
These improvements will allow for faster processing times and reduce the dependency on large computing infrastructures, facilitating the development of more accessible and user-friendly solutions.
Finally, as multimodal processing becomes more prevalent, ethical considerations surrounding data privacy, security, and bias will continue to be of paramount importance.
Addressing these concerns will be crucial to ensuring that these powerful technologies benefit society responsibly and equitably.
In conclusion, multimodal information processing is a revolutionary approach that enables complex data analysis through the integration of diverse modalities.
Its application spans countless fields and promises to drive innovation across industries, enhancing how we interpret and interact with the world around us.
資料ダウンロード
QCD調達購買管理クラウド「newji」は、調達購買部門で必要なQCD管理全てを備えた、現場特化型兼クラウド型の今世紀最高の購買管理システムとなります。
ユーザー登録
調達購買業務の効率化だけでなく、システムを導入することで、コスト削減や製品・資材のステータス可視化のほか、属人化していた購買情報の共有化による内部不正防止や統制にも役立ちます。
NEWJI DX
製造業に特化したデジタルトランスフォーメーション(DX)の実現を目指す請負開発型のコンサルティングサービスです。AI、iPaaS、および先端の技術を駆使して、製造プロセスの効率化、業務効率化、チームワーク強化、コスト削減、品質向上を実現します。このサービスは、製造業の課題を深く理解し、それに対する最適なデジタルソリューションを提供することで、企業が持続的な成長とイノベーションを達成できるようサポートします。
オンライン講座
製造業、主に購買・調達部門にお勤めの方々に向けた情報を配信しております。
新任の方やベテランの方、管理職を対象とした幅広いコンテンツをご用意しております。
お問い合わせ
コストダウンが利益に直結する術だと理解していても、なかなか前に進めることができない状況。そんな時は、newjiのコストダウン自動化機能で大きく利益貢献しよう!
(Β版非公開)