- お役立ち記事
- Data analysis method from a small amount of data using machine learning and experimental design and its application to material development and process design
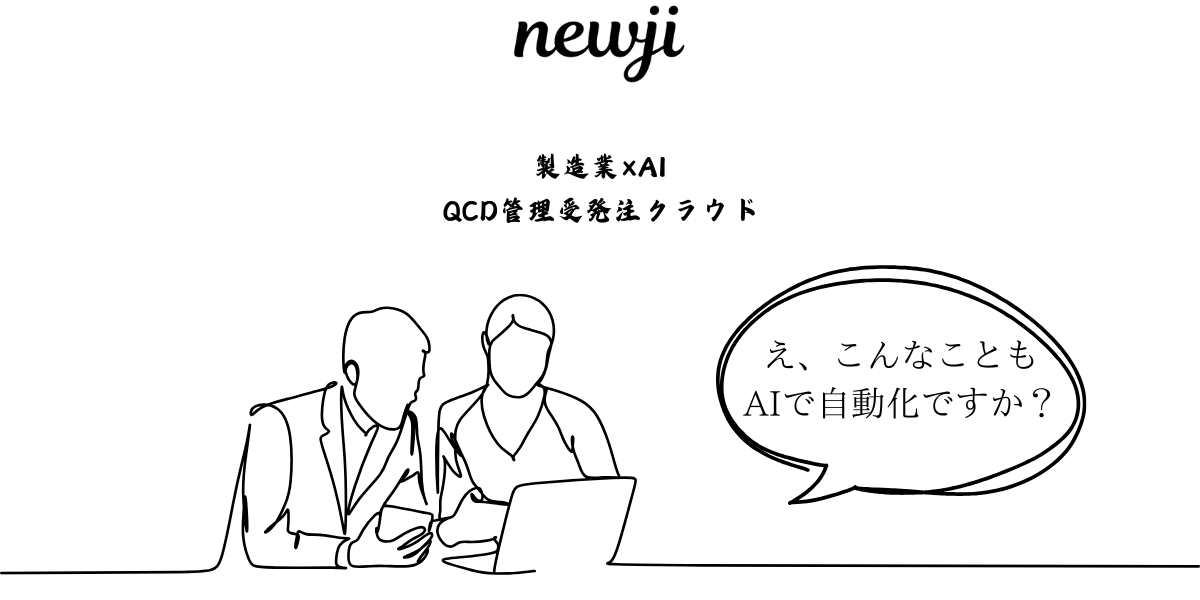
Data analysis method from a small amount of data using machine learning and experimental design and its application to material development and process design
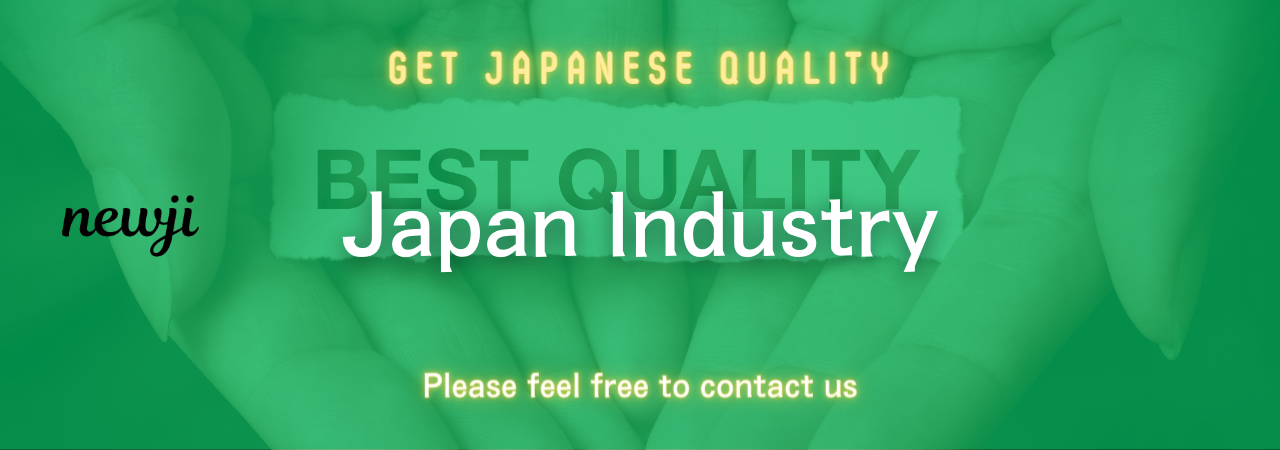
目次
Understanding Data Analysis with Machine Learning
Data analysis plays a significant role in today’s world.
It helps us make sense of the vast amounts of information that we encounter daily.
But what if you only have a small amount of data?
This is where the power of machine learning and experimental design comes into play.
These methods allow us to extract valuable insights even from limited data samples.
Machine learning algorithms have gained popularity because of their ability to identify patterns and make predictions.
They learn from data, much like humans learn from experience.
By applying these algorithms to small datasets, you can uncover hidden trends and make informed decisions.
Importance of Experimental Design
Experimental design is a critical component of data analysis.
It involves creating structured experiments to test hypotheses and gather data.
The objective is to control variables, allowing for precise measurement of the factors of interest.
When combined with machine learning, experimental design provides a powerful tool for analyzing small datasets.
By planning an experiment properly, you can minimize errors and maximize the quality of the data obtained.
This is especially important when dealing with limited data.
A well-designed experiment ensures that the results are reliable and meaningful.
Applications in Material Development
Material development is one area where data analysis, machine learning, and experimental design have a significant impact.
Materials science seeks to develop new materials with improved properties, such as strength, durability, or conductivity.
Researchers often face the challenge of working with limited datasets due to the high cost and time-intensive nature of experiments.
Using machine learning, researchers can predict the properties of new materials based on existing data.
Algorithms can identify relationships between the composition of materials and their properties.
This approach accelerates the discovery process by reducing the need for extensive trial-and-error experiments.
Experimental design helps by enabling more efficient testing of different material configurations.
By systematically varying composition and other factors, researchers can hone in on optimal solutions more quickly.
This methodology significantly speeds up the material development process.
Transforming Process Design with Data Analysis
Process design is another field benefitting from data analysis and machine learning.
In industries such as manufacturing, the goal is to optimize processes for efficiency, cost-effectiveness, and quality control.
Machine learning applications in process design include predictive maintenance, quality prediction, and process optimization.
By analyzing historical data, machine learning models can predict equipment failure before it happens.
This minimizes downtime, saving both time and money.
Experimental design ensures that the essential variables within a process are appropriately controlled and tested.
When combined with machine learning, it allows for detailed examination and simulation of complex processes.
This leads to better-informed decision-making and process adjustments.
Machine Learning Techniques for Small Data
While machine learning is typically associated with large datasets, certain techniques can be particularly effective for small data.
An important method is transfer learning, which involves using a model trained on a large dataset as a starting point for a smaller dataset.
This approach can significantly boost the performance of a machine learning model.
Bayesian methods are another powerful tool, allowing for robust predictions even when data is scarce.
These methods incorporate prior knowledge or beliefs into the analysis, leading to more accurate estimates.
Dimensionality reduction techniques, like Principal Component Analysis (PCA), help isolate the most informative features of a dataset.
This can be particularly beneficial when dealing with small data, as it reduces noise and potential overfitting.
Challenges and Considerations
Despite the potential, several challenges arise when applying machine learning and experimental design to small datasets.
These include the risk of overfitting, where a model learns the noise of the data rather than the signal.
Careful validation is key to avoiding overfitting.
Techniques such as cross-validation can help assess the model’s performance on different subsets of the data, ensuring its robustness.
Additionally, the quality of the data is crucial.
Poor-quality data can lead to inaccurate models, regardless of the sophistication of the analysis methods.
Data cleaning and preprocessing are vital steps before applying machine learning algorithms.
Finally, it’s essential to maintain a clear understanding of the domain.
Expert knowledge can inform the selection of appropriate models and interpretation of results.
The Future of Data-Driven Innovation
As data analysis, machine learning, and experimental design continue to advance, their applications in material development and process design will expand.
These methods offer the ability to extract meaningful insights from limited data, transforming the way industries approach innovation.
The integration of these techniques holds the promise of faster development cycles, reduced resource consumption, and enhanced product and process performance.
Ultimately, leveraging these data-driven methods can lead to significant breakthroughs and improvements across various fields.
By harnessing the power of machine learning and experimental design, professionals can unlock the full potential of small datasets, driving innovation and progress forward efficiently.
資料ダウンロード
QCD調達購買管理クラウド「newji」は、調達購買部門で必要なQCD管理全てを備えた、現場特化型兼クラウド型の今世紀最高の購買管理システムとなります。
ユーザー登録
調達購買業務の効率化だけでなく、システムを導入することで、コスト削減や製品・資材のステータス可視化のほか、属人化していた購買情報の共有化による内部不正防止や統制にも役立ちます。
NEWJI DX
製造業に特化したデジタルトランスフォーメーション(DX)の実現を目指す請負開発型のコンサルティングサービスです。AI、iPaaS、および先端の技術を駆使して、製造プロセスの効率化、業務効率化、チームワーク強化、コスト削減、品質向上を実現します。このサービスは、製造業の課題を深く理解し、それに対する最適なデジタルソリューションを提供することで、企業が持続的な成長とイノベーションを達成できるようサポートします。
オンライン講座
製造業、主に購買・調達部門にお勤めの方々に向けた情報を配信しております。
新任の方やベテランの方、管理職を対象とした幅広いコンテンツをご用意しております。
お問い合わせ
コストダウンが利益に直結する術だと理解していても、なかなか前に進めることができない状況。そんな時は、newjiのコストダウン自動化機能で大きく利益貢献しよう!
(Β版非公開)