- お役立ち記事
- Fundamentals of deep learning, image recognition/image generation technology, and its applications
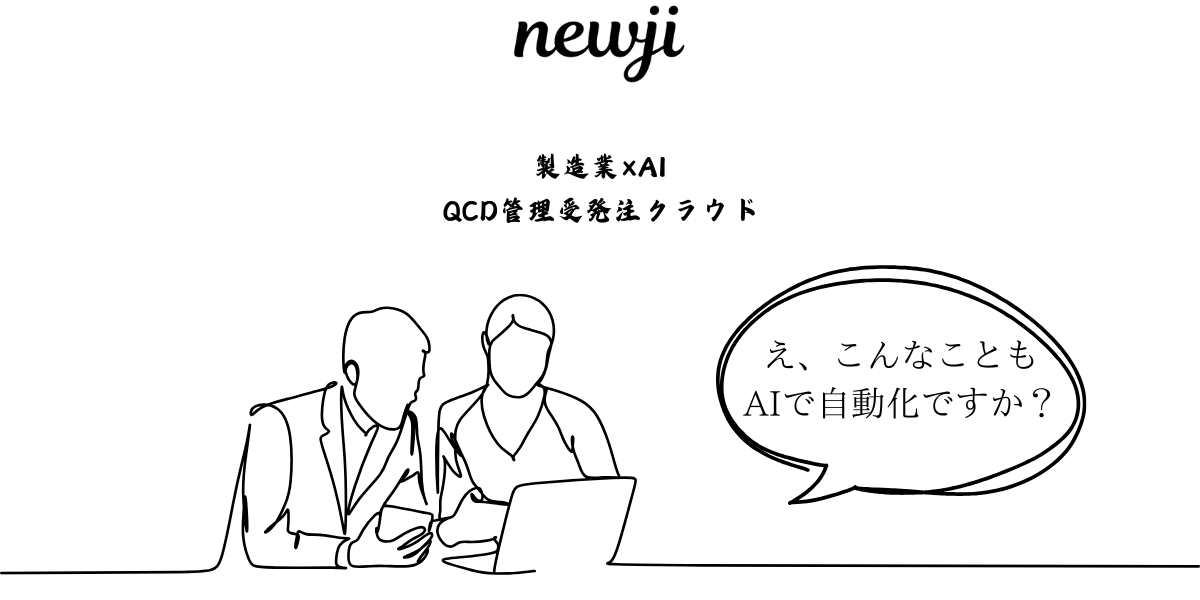
Fundamentals of deep learning, image recognition/image generation technology, and its applications
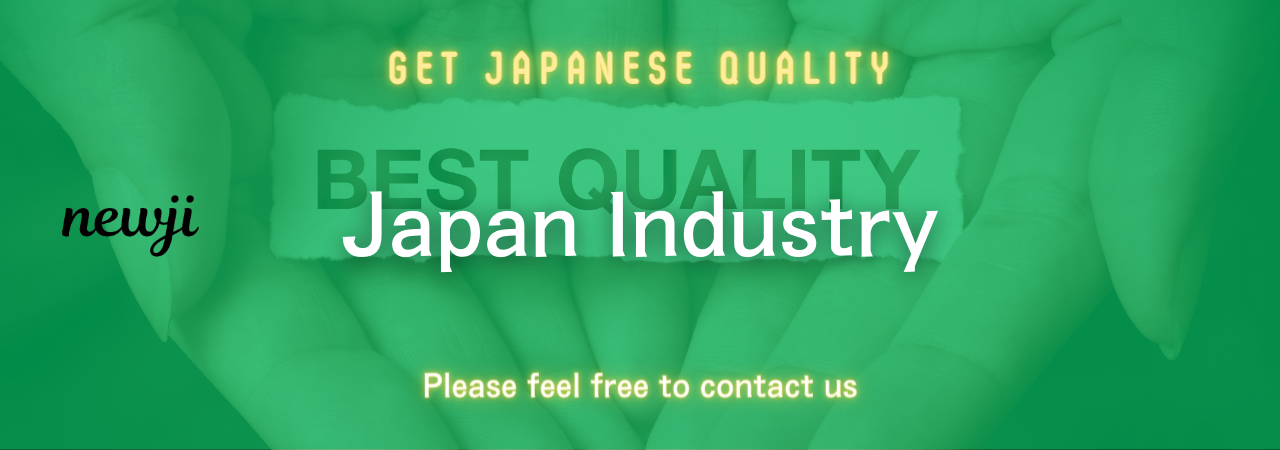
目次
Understanding Deep Learning
Deep learning is a subset of machine learning that falls under the broader spectrum of artificial intelligence.
It’s called “deep” learning because it involves neural networks with many layers – sometimes even hundreds.
These networks attempt to simulate the behavior of the human brain to learn from large amounts of data.
Deep learning is all about using algorithms that enable computers to learn patterns and apply this understanding to make decisions or predictions.
Some of the common types of neural networks used in deep learning include Convolutional Neural Networks (CNNs), Recurrent Neural Networks (RNNs), and Generative Adversarial Networks (GANs).
Through deep learning, computers have achieved remarkable capabilities like understanding speech, identifying objects in images, translating languages, and even creating impressive art.
Image Recognition Technology
One of the most celebrated applications of deep learning is image recognition.
This technology enables computers to interpret and categorize whatever they see.
Image recognition is commonly used in facial recognition technology, where a device recognizes and verifies a human face.
It’s also central in identification systems for objects, handwriting, and more.
This technology relies heavily on CNNs, which are particularly effective at processing structured grid data, like images.
By analyzing pixels in an image layer by layer, CNNs can identify complex patterns.
In practical terms, image recognition is utilized in various contexts: from social media platforms like Facebook tagging friends in photos to security systems in airports scanning for dangerous items.
How Image Recognition Works
To understand how image recognition works, it’s helpful to know about some fundamental processes involved.
The process involves detecting, classifying, and then interpreting images.
First, computers must preprocess images, converting them into grayscale or other forms that make them easier to handle.
Next, the neural network identifies features within the image—like edges, curves, and textures—building layers of understanding until the image can be classified.
Once these features are recognized, the model compares them to a training set—previously labeled images—to generate accurate predictions or recognition of the object.
Applications of Image Recognition
Image recognition is thriving beyond typical tech sectors as it branches into industries like healthcare, retail, and automotive.
In healthcare, image recognition is used in radiology to differentiate between malignant and benign tumors, aiding doctors in diagnostic tasks.
Self-driving cars utilize this technology to recognize traffic signs, pedestrians, and barriers.
Retailers use it to understand shopping behaviors and tailor the shopping experience uniquely through image-based product recommendations and inventory management.
Image Generation Technology
While image recognition involves identifying existing images, image generation technology does the opposite—it creates new images based on learned data.
This is often carried out using Generative Adversarial Networks (GANs).
GANs consist of two neural networks, a generator and a discriminator, that work against each other.
The generator aims to create new data resembling the training set, while the discriminator evaluates the authenticity of the generated data.
This technology is the driving force behind the creation of everything from realistic human faces not based on real people to deepfake videos.
Applications of Image Generation
Image generation is seeing substantial growth due to its broad applicability in creative industries and beyond.
It allows for the creation of virtual environments in video games, influences modern art, and develops digital marketing content personalized to target audiences.
In entertainment, GANs have been used to age or de-age actors in movies, bringing historical figures back to life virtually.
Marketing and advertising agencies leverage this technology to generate compelling visuals for campaigns, enhancing their creative methods.
Additionally, image generation supports research in areas such as climate change, automating complex visualizations for better understanding and communication of data-driven insights.
Deep Learning in the Real World
The practical applications of deep learning, including image recognition and generation, are vast and continuously expanding.
Businesses utilize it to streamline operations, educators employ it for personalized learning environments, and scientists harness it for research and development.
For consumers, it integrates seamlessly into daily life through voice assistants, personalized content, and smart home devices.
Challenges and Future Prospects
However, there are challenges to consider alongside deep learning’s promising applications.
These include concerns over privacy, especially with facial recognition, and ethical issues concerning bias in AI algorithms.
There remains a need for comprehensive regulatory frameworks to guide the ethical deployment of deep learning technologies.
Additionally, ensuring transparency in how AI systems arrive at their decisions is crucial.
Looking forward, ongoing research aims to solve current limitations, such as model interpretability and data efficiency, while pioneering new applications.
The potential for deep learning to revolutionize industries is enormous, promising advancements in automation, personalization, and efficiency.
Deep learning, with its image recognition and generation capabilities, continues to shape our modern world—transforming complex processes into tangible solutions that improve our daily experiences.
資料ダウンロード
QCD調達購買管理クラウド「newji」は、調達購買部門で必要なQCD管理全てを備えた、現場特化型兼クラウド型の今世紀最高の購買管理システムとなります。
ユーザー登録
調達購買業務の効率化だけでなく、システムを導入することで、コスト削減や製品・資材のステータス可視化のほか、属人化していた購買情報の共有化による内部不正防止や統制にも役立ちます。
NEWJI DX
製造業に特化したデジタルトランスフォーメーション(DX)の実現を目指す請負開発型のコンサルティングサービスです。AI、iPaaS、および先端の技術を駆使して、製造プロセスの効率化、業務効率化、チームワーク強化、コスト削減、品質向上を実現します。このサービスは、製造業の課題を深く理解し、それに対する最適なデジタルソリューションを提供することで、企業が持続的な成長とイノベーションを達成できるようサポートします。
オンライン講座
製造業、主に購買・調達部門にお勤めの方々に向けた情報を配信しております。
新任の方やベテランの方、管理職を対象とした幅広いコンテンツをご用意しております。
お問い合わせ
コストダウンが利益に直結する術だと理解していても、なかなか前に進めることができない状況。そんな時は、newjiのコストダウン自動化機能で大きく利益貢献しよう!
(Β版非公開)