- お役立ち記事
- Fundamentals of Bayesian statistics and subjective probability
Fundamentals of Bayesian statistics and subjective probability
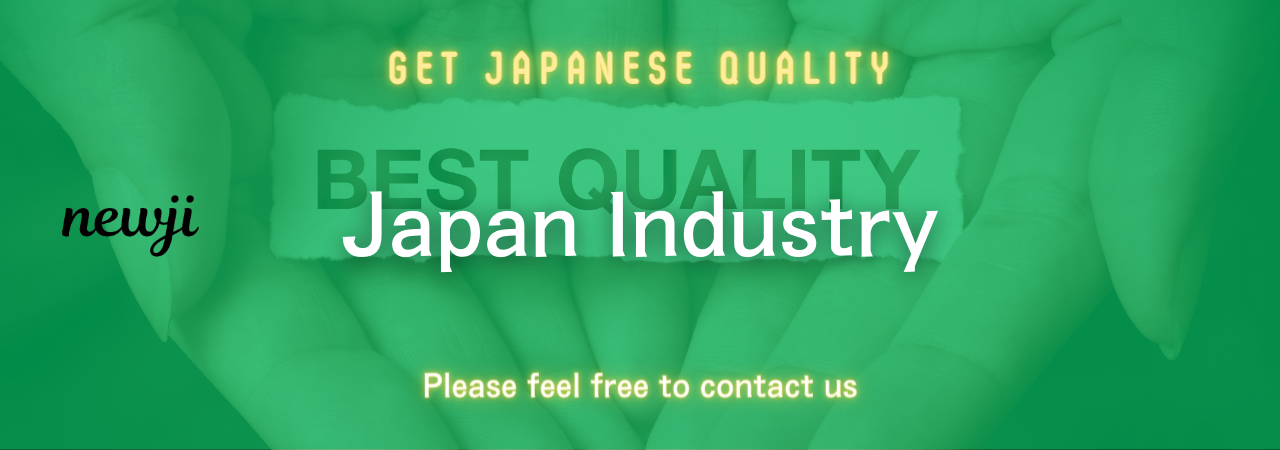
目次
Understanding Bayesian Statistics
Bayesian statistics is a branch of statistics that uses probability to represent uncertainty about the world.
Unlike classical statistics, which focuses more on frequency and likelihood, Bayesian statistics incorporates prior knowledge or beliefs when making inferences.
This approach allows statisticians to update probabilities as more evidence or information becomes available.
The foundation of Bayesian statistics is Bayes’ Theorem, a simple yet powerful mathematical formula that connects conditional and marginal probabilities.
Bayes’ Theorem helps us calculate the probability of a hypothesis given new data, integrating both prior beliefs and evidence to produce a more informed result.
To better grasp Bayesian statistics, imagine you’re a doctor determining the likelihood of a disease based on a test result.
You already know the estimated prevalence of the disease in the general population, and you also understand the accuracy of the test based on past cases.
Bayesian statistics allows you to combine these pieces of information to make a more reliable diagnosis.
Bayes’ Theorem: The Core Concept
Bayes’ Theorem is at the heart of Bayesian statistics.
It provides a mathematical framework to update the probability of a hypothesis as new evidence is introduced.
The theorem can be expressed as follows:
\[ P(H|E) = \frac{P(E|H) \times P(H)}{P(E)} \]
Where:
– \( P(H|E) \) is the probability of the hypothesis \( H \) given the evidence \( E \) (called the posterior probability).
– \( P(E|H) \) is the probability of the evidence \( E \) given that the hypothesis \( H \) is true (the likelihood).
– \( P(H) \) is the prior probability of the hypothesis before considering the evidence.
– \( P(E) \) is the probability of the evidence, which ensures the probabilities sum up correctly.
In simple terms, Bayes’ Theorem shows how to update our belief about a hypothesis by taking into account new evidence.
It emphasizes the role of prior knowledge in shaping our conclusions and enhances our ability to make predictions under uncertainty.
Priors: Introducing Subjective Probability
A distinctive feature of Bayesian statistics is its use of prior probabilities, which can be subjective.
Priors reflect our beliefs about a parameter or hypothesis before observing new data.
These beliefs might stem from historical data, expert opinions, or logical reasoning.
There are different types of priors used in Bayesian analysis:
1. **Informative Priors**: These incorporate specific, existing knowledge about a parameter.
For instance, if you’ve conducted similar experiments in the past, an informative prior would utilize this history to guide your analysis.
2. **Non-informative Priors**: Also known as vague or flat priors, these carry very little assumption about the parameter values.
They are often used when there’s a lack of previous data or when you wish to minimize the influence of prior beliefs on the analysis.
3. **Conjugate Priors**: Chosen for mathematical convenience, conjugate priors enable the resulting posterior distribution to be in the same family as the prior.
This can simplify calculations and make the analysis more straightforward.
Selecting the right prior is a critical decision in Bayesian analysis since it can influence the resulting posterior probabilities.
However, with adequate data, the influence of the prior tends to diminish as the evidence accumulates.
Posterior Probability and Decision Making
The beauty of Bayesian statistics lies in its ability to refine our inferences and decisions.
Once the prior and the likelihood are combined through Bayes’ Theorem, we get the posterior probability.
This updated probability distribution represents our new belief about the hypothesis after considering the evidence.
It combines both prior information and new data, offering a more comprehensive view of what’s likely true.
Posterior probabilities are key tools for decision-making in various fields, from medicine to machine learning and beyond.
For example, consider a machine learning model that predicts customer preferences based on historical purchase behavior.
Bayesian statistics allows the model to update its predictions when customer behavior changes, maintaining accuracy over time.
Applications of Bayesian Statistics
Bayesian statistics is widely used across different domains and industries due to its flexibility and robustness.
1. **Medical Research**: In clinical trials, Bayesian methods can help account for diverse sources of uncertainty, such as variable patient responses and prior scientific knowledge.
This approach provides more adaptable ways to assess the effectiveness of new treatments.
2. **Economics and Finance**: Bayesian analysis assists in modeling economic phenomena and financial markets, where uncertainty and prior beliefs play pivotal roles.
Analysts use Bayesian methods to predict market trends and improve investment strategies.
3. **Machine Learning**: Many advanced algorithms in machine learning, such as Bayesian networks and Gaussian processes, rely on Bayesian principles to handle uncertainty and improve predictive accuracy.
4. **Psychology and Social Sciences**: Bayesian methods allow researchers to combine evidence from various studies while considering subjective interpretations, crucial for understanding complex human behaviors.
Conclusion
Understanding Bayesian statistics and subjective probability opens new pathways for more informed decision-making and data analysis.
By blending prior knowledge with new data, Bayesian methods provide versatile and effective tools for dealing with uncertainty across diverse fields.
Whether you’re diagnosing medical conditions, predicting economic trends, or building machine-learning models, integrating Bayesian approaches can lead to smarter insights and better results.
As you continue to explore the world of statistics, keep in mind the power of Bayesian thinking to transform data into actionable knowledge.
資料ダウンロード
QCD調達購買管理クラウド「newji」は、調達購買部門で必要なQCD管理全てを備えた、現場特化型兼クラウド型の今世紀最高の購買管理システムとなります。
ユーザー登録
調達購買業務の効率化だけでなく、システムを導入することで、コスト削減や製品・資材のステータス可視化のほか、属人化していた購買情報の共有化による内部不正防止や統制にも役立ちます。
NEWJI DX
製造業に特化したデジタルトランスフォーメーション(DX)の実現を目指す請負開発型のコンサルティングサービスです。AI、iPaaS、および先端の技術を駆使して、製造プロセスの効率化、業務効率化、チームワーク強化、コスト削減、品質向上を実現します。このサービスは、製造業の課題を深く理解し、それに対する最適なデジタルソリューションを提供することで、企業が持続的な成長とイノベーションを達成できるようサポートします。
オンライン講座
製造業、主に購買・調達部門にお勤めの方々に向けた情報を配信しております。
新任の方やベテランの方、管理職を対象とした幅広いコンテンツをご用意しております。
お問い合わせ
コストダウンが利益に直結する術だと理解していても、なかなか前に進めることができない状況。そんな時は、newjiのコストダウン自動化機能で大きく利益貢献しよう!
(Β版非公開)