- お役立ち記事
- Fundamentals of sensor signal processing technology, noise removal/feature extraction methods, and implementation programming
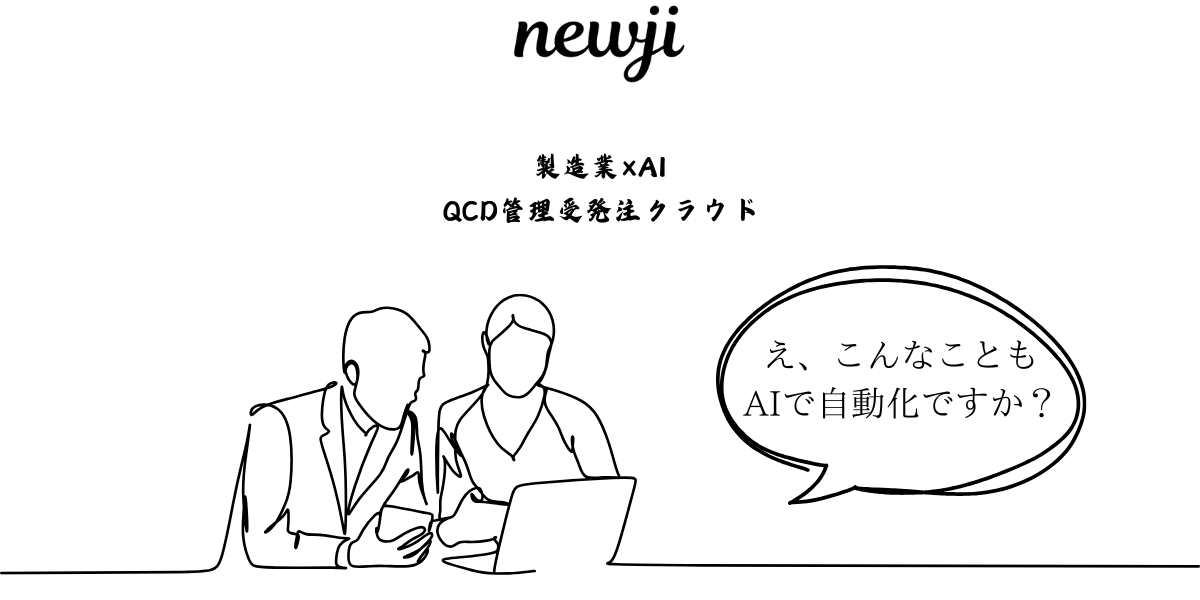
Fundamentals of sensor signal processing technology, noise removal/feature extraction methods, and implementation programming
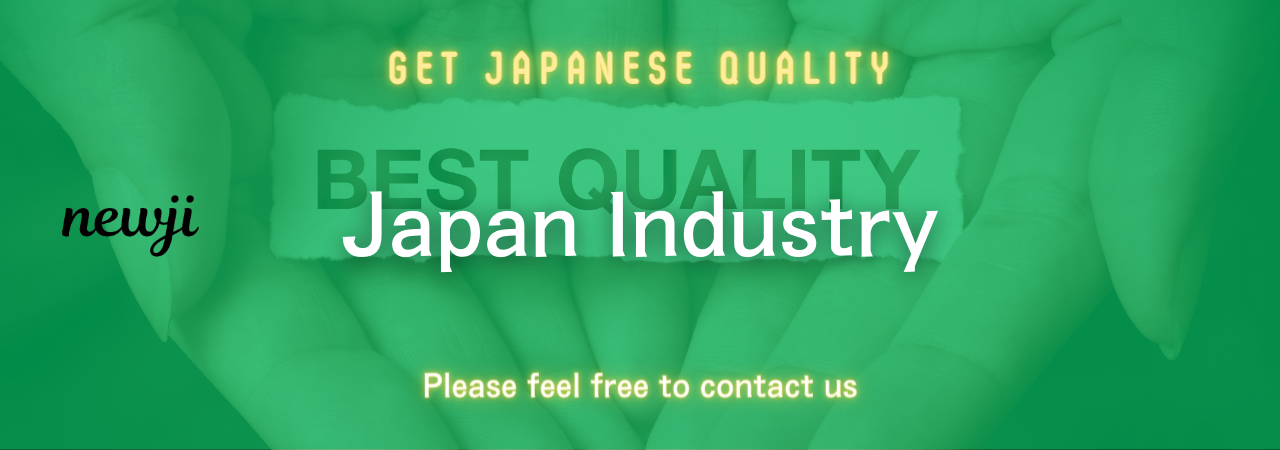
Understanding Sensor Signal Processing
Sensor signal processing is a crucial aspect of many modern technologies, from smartphones to industrial machinery, enabling devices to interpret real-world inputs accurately.
At its core, this process involves converting raw data collected by sensors into actionable information.
To fully grasp the concept, let’s delve into the fundamentals of this technology, including noise removal, feature extraction methods, and implementation in programming.
What is Sensor Signal Processing?
Sensor signal processing refers to the various techniques and methods used to convert raw data from sensors into useful information.
Sensors, such as accelerometers, temperature sensors, and cameras, produce signals that typically contain noise and other distortions.
The goal of signal processing is to clean these signals and extract meaningful features that can be utilized in various applications.
The Importance of Noise Removal
Noise is any unwanted or irrelevant data that can distort the true signal.
In sensor data, noise can originate from environmental factors, electronic interference, or the sensors themselves.
Removing noise is essential for ensuring that the data analyzed truly represents the phenomena being measured.
There are several techniques for noise removal, including:
– **Filtering:** One of the most common methods, filtering involves using algorithms to separate noise from the actual signal.
Filters such as low-pass, high-pass, and band-pass are frequently used to allow or block specific frequencies.
– **Smoothing:** Smoothing techniques, like the moving average method, are used to even out short-term fluctuations, thus revealing important trends and cycles.
– **Statistical Methods:** Techniques like principal component analysis (PCA) can reduce noise by transforming data into a space where noise is minimized.
Feature Extraction Techniques
Once noise is minimized, the next step is feature extraction, which involves identifying relevant pieces of information that can be used for further analysis.
Feature extraction is crucial for applications such as pattern recognition, data compression, and machine learning.
Some common feature extraction techniques include:
– **Fourier Transform:** This technique transforms signal data into their frequency components, making it easier to analyze complex signals.
– **Wavelet Transform:** Unlike the Fourier Transform, wavelet transform provides both time and frequency information, which is useful for analyzing non-stationary signals.
– **Principal Component Analysis (PCA):** PCA reduces the dimensionality of data, helping highlight significant patterns and correlations.
– **Time-Domain Features:** Parameters such as mean, median, variance, and entropy can quickly describe signal characteristics relevant to the application.
Programming Implementation
Turning theory into practice requires implementing these techniques in software.
Here are some key points to consider when programming sensor signal processing.
– **Choosing the Right Tools:** Popular programming languages for signal processing include Python, MATLAB, and C++.
Libraries such as NumPy and SciPy in Python or Signal Processing Toolbox in MATLAB offer ready-made functions for noise reduction and feature extraction.
– **Real-time Processing:** For many applications, such as wearable devices or autonomous vehicles, real-time processing is critical.
Programming for real-time requires efficient algorithm implementations and often the use of specialized hardware accelerators.
– **Machine Learning Integration:** With the rise of machine learning, integrating feature extraction with machine learning models can enhance pattern recognition and prediction capabilities.
Frameworks like TensorFlow or PyTorch can be used in conjunction with traditional signal processing techniques.
Applications of Sensor Signal Processing
Sensor signal processing is used in many fields, each requiring tailored techniques to suit specific needs.
– **Healthcare:** In medical devices, accurate signal processing is vital for monitoring vital signs, diagnostics, and imaging.
– **Automotive:** Sensors are integral in autonomous vehicles for navigation and safety systems.
Signal processing aids in interpreting vast amounts of data from cameras, lidars, and radars.
– **Consumer Electronics:** Smartphones and wearables rely heavily on processed sensor data for features like activity tracking, image stabilization, and augmented reality.
Conclusion
Understanding the fundamentals of sensor signal processing, including noise removal and feature extraction, is vital for leveraging sensor data.
By employing the right techniques and programming strategies, industries can achieve greater accuracy and efficiency in their operations.
With the ever-growing scope of sensor applications, proficiency in signal processing will continue to be a valuable skill in technology development.
資料ダウンロード
QCD調達購買管理クラウド「newji」は、調達購買部門で必要なQCD管理全てを備えた、現場特化型兼クラウド型の今世紀最高の購買管理システムとなります。
ユーザー登録
調達購買業務の効率化だけでなく、システムを導入することで、コスト削減や製品・資材のステータス可視化のほか、属人化していた購買情報の共有化による内部不正防止や統制にも役立ちます。
NEWJI DX
製造業に特化したデジタルトランスフォーメーション(DX)の実現を目指す請負開発型のコンサルティングサービスです。AI、iPaaS、および先端の技術を駆使して、製造プロセスの効率化、業務効率化、チームワーク強化、コスト削減、品質向上を実現します。このサービスは、製造業の課題を深く理解し、それに対する最適なデジタルソリューションを提供することで、企業が持続的な成長とイノベーションを達成できるようサポートします。
オンライン講座
製造業、主に購買・調達部門にお勤めの方々に向けた情報を配信しております。
新任の方やベテランの方、管理職を対象とした幅広いコンテンツをご用意しております。
お問い合わせ
コストダウンが利益に直結する術だと理解していても、なかなか前に進めることができない状況。そんな時は、newjiのコストダウン自動化機能で大きく利益貢献しよう!
(Β版非公開)