- お役立ち記事
- Basics of edge computing, key points for practical use, and application to lightweight AI implementation technology
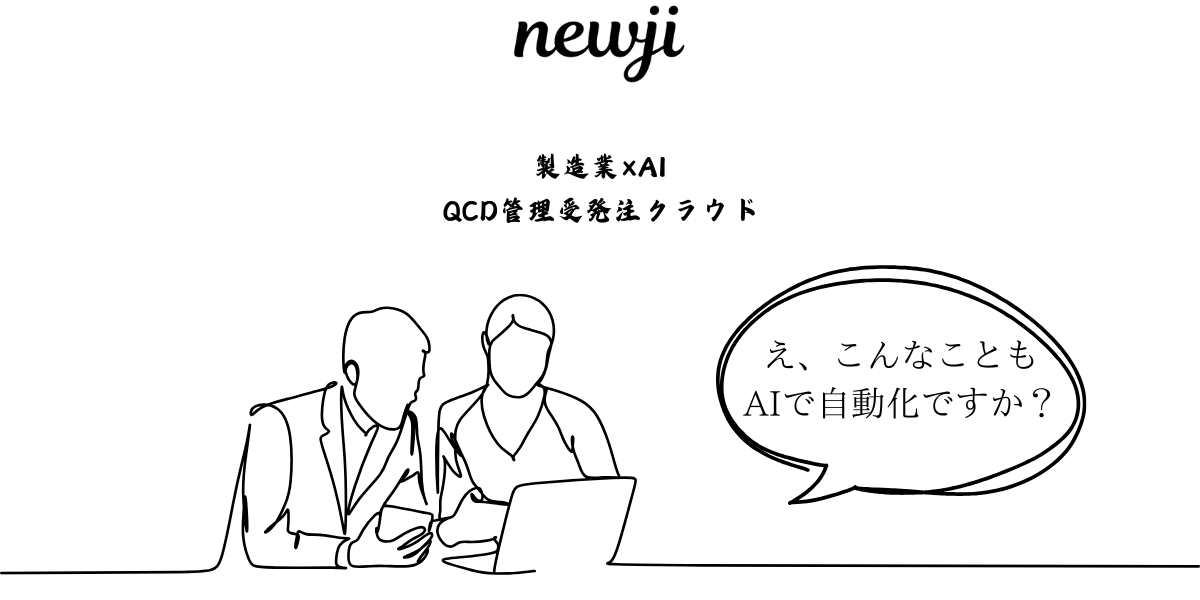
Basics of edge computing, key points for practical use, and application to lightweight AI implementation technology
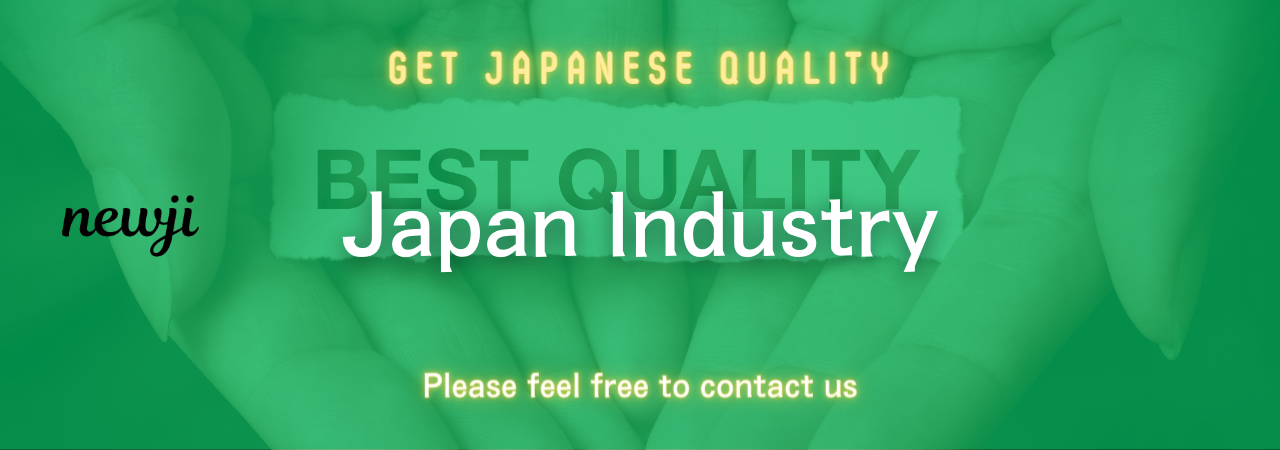
目次
Understanding Edge Computing
Edge computing is a transformative approach in computing technology that shifts data processing closer to the source of data generation, rather than relying heavily on centralized data centers.
This method is gaining traction due to its ability to enhance speed, reliability, and reduce latency in data processing.
In traditional cloud computing, data is sent to a centralized server for processing, which can result in delays and bandwidth constraints, especially for time-sensitive applications.
Edge computing alleviates these issues by processing data locally, at or near the source, which greatly improves efficiency and performance.
A prime example of edge computing in everyday use is in smart devices or wearables, such as fitness trackers.
These devices collect data continuously and perform real-time analysis to provide instant feedback to the user.
By processing data on the device itself or nearby, edge computing enables quick responses and reduces the need for constant communication with remote servers.
Thus, it makes technologies more responsive and interactive, providing users with faster and more reliable services.
Key Points for Practical Use
When implementing edge computing, there are several key points to consider to harness its full potential effectively.
1. Data Security and Privacy
With data being processed closer to its source, edge computing can potentially improve data security and privacy.
Fewer data are transmitted over large distances, reducing the risk of interception during transit.
Additionally, sensitive information can be kept local, rather than in the cloud, safeguarding it from potential vulnerabilities inherent in cloud storage.
However, it is still crucial to implement robust security measures on the edge devices themselves to protect from unauthorized access or attacks.
2. Scalability
Edge computing must be carefully planned to support scalability.
As the number of connected devices increases, the network should be able to handle additional demand without degrading performance.
This involves ensuring enough processing power and bandwidth at the edge to accommodate growth and the potential spike in data traffic.
A scalable edge computing infrastructure can efficiently adapt to varying loads and continue delivering high performance.
3. Power and Resource Management
Managing power consumption effectively is essential in edge computing.
Edge devices, often being IoT devices, may operate in environments where power supply is limited or battery life is a concern.
Resource management strategies, such as optimized computational tasks and efficient data transmission protocols, can help prolong device life and ensure sustained operation.
Adopting energy-efficient hardware components can also significantly contribute to reducing power usage.
4. Integration and Interoperability
To fully realize the advantages of edge computing, systems need to integrate smoothly with existing IT infrastructure.
Interoperability across various devices and platforms is crucial for seamless data exchange and processing.
Utilizing standardized protocols and interfaces can ensure compatibility and facilitate communication between different components within the system.
5. Deployment and Management
Deploying edge computing systems requires careful planning and management.
Strategically placing edge devices and nodes in optimal locations can improve system performance by reducing latency and improving data processing speeds.
Moreover, ongoing maintenance and management are essential to address issues promptly and keep the system running efficiently.
Applying Edge Computing to Lightweight AI Implementation
One of the exciting applications of edge computing is in the domain of artificial intelligence (AI), particularly with lightweight AI models.
These AI models are designed to run on edge devices, enabling real-time data processing and decision-making without the need for cloud-based AI computations.
This has significant implications in various sectors such as healthcare, automotive, and consumer electronics.
Real-Time Processing and Analysis
Edge computing empowers AI applications by enabling real-time data processing and analysis.
For example, in autonomous vehicles, rapid decision-making is crucial for safety.
Edge AI can process data from sensors and cameras instantly to make vehicle control decisions without relying on cloud connectivity.
This not only reduces latency but also improves the reliability of the system by ensuring it remains operational even in areas with poor network coverage.
Enhanced Privacy
In AI applications, particularly those involving personal data, privacy is a significant concern.
By processing data on the edge, sensitive information can be kept within the device, offering enhanced privacy protections.
This is particularly relevant in healthcare applications, where edge AI can analyze health data locally on a patient’s device, minimizing the risk of data breaches.
Energy Efficiency
Edge AI models are designed to be lightweight, meaning they require less computational power and energy compared to larger models running in the cloud.
This energy efficiency is crucial for battery-operated IoT devices, allowing them to perform intelligent tasks without exhausting their power resources quickly.
Thus, edge AI supports sustainable and prolonged operation in low-power environments.
Improved Network Bandwidth
With edge computing, only critical information needs to be sent to the cloud, if at all.
This reduces the amount of data transmitted over the network, freeing up bandwidth and reducing costs.
For example, in smart surveillance systems, edge AI can analyze video footage locally and only send relevant events or anomalies to central servers, drastically cutting down network usage.
Conclusion
Edge computing represents a significant leap in how data is processed and managed, offering a myriad of benefits including faster processing speeds, improved privacy, and efficient resource use.
Its synergy with lightweight AI implementation further underscores its potential to revolutionize industries by enabling smarter, more responsive technologies.
As the number of connected devices continues to expand, edge computing will play a critical role in supporting a seamless and efficient digital experience.
By understanding its foundational concepts and addressing key practical considerations, businesses and developers can effectively leverage edge computing to innovate and optimize their operations.
資料ダウンロード
QCD調達購買管理クラウド「newji」は、調達購買部門で必要なQCD管理全てを備えた、現場特化型兼クラウド型の今世紀最高の購買管理システムとなります。
ユーザー登録
調達購買業務の効率化だけでなく、システムを導入することで、コスト削減や製品・資材のステータス可視化のほか、属人化していた購買情報の共有化による内部不正防止や統制にも役立ちます。
NEWJI DX
製造業に特化したデジタルトランスフォーメーション(DX)の実現を目指す請負開発型のコンサルティングサービスです。AI、iPaaS、および先端の技術を駆使して、製造プロセスの効率化、業務効率化、チームワーク強化、コスト削減、品質向上を実現します。このサービスは、製造業の課題を深く理解し、それに対する最適なデジタルソリューションを提供することで、企業が持続的な成長とイノベーションを達成できるようサポートします。
オンライン講座
製造業、主に購買・調達部門にお勤めの方々に向けた情報を配信しております。
新任の方やベテランの方、管理職を対象とした幅広いコンテンツをご用意しております。
お問い合わせ
コストダウンが利益に直結する術だと理解していても、なかなか前に進めることができない状況。そんな時は、newjiのコストダウン自動化機能で大きく利益貢献しよう!
(Β版非公開)