- お役立ち記事
- Key points for appropriate parameter settings in system identification using Bayesian estimation method
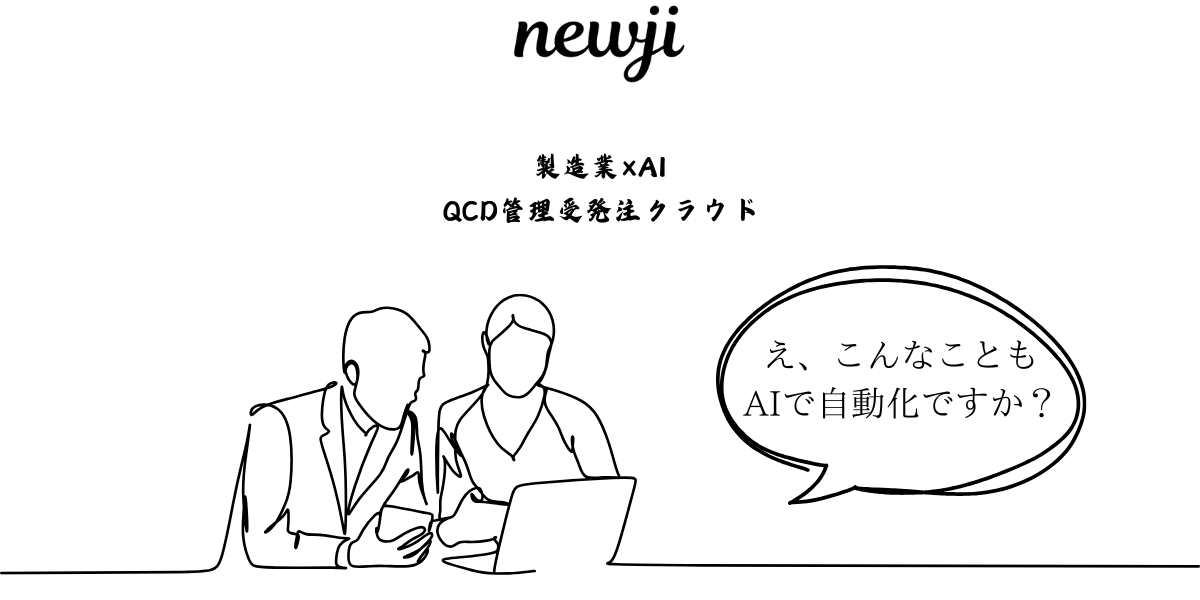
Key points for appropriate parameter settings in system identification using Bayesian estimation method
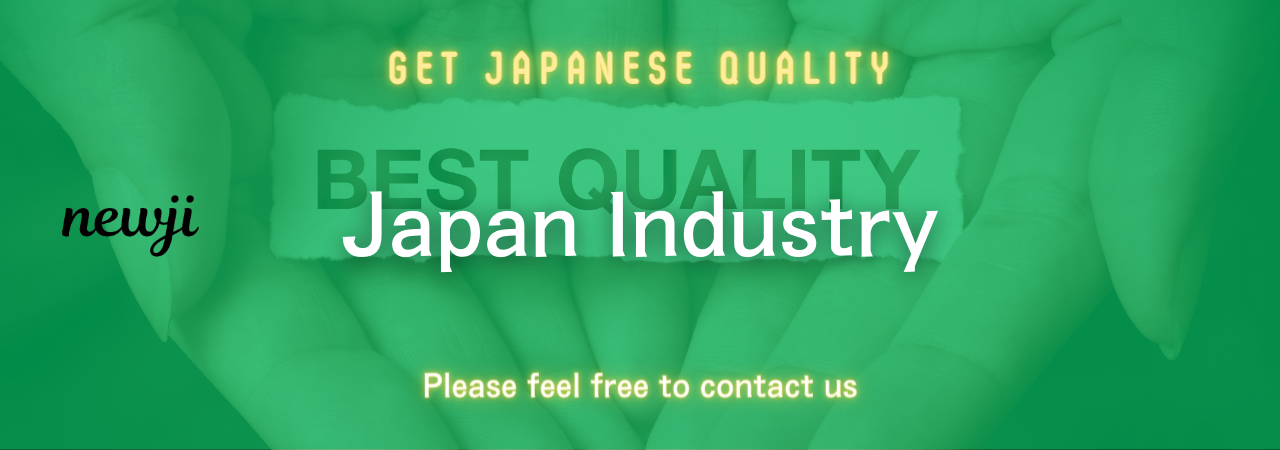
目次
Understanding System Identification
System identification is a process that involves developing mathematical models of dynamic systems based on measured data.
It’s primarily used in engineering for the purpose of analyzing and designing electrical systems, mechanical structures, and other technical systems.
This involves adjusting various parameters in the system’s mathematical model to ensure it mirrors the real-world behavior of the system being studied.
What is Bayesian Estimation?
Bayesian estimation is a statistical technique that updates the probabilities for a hypothesis as more evidence or information becomes available.
It’s particularly useful in system identification as it provides a robust method for parameter estimation.
In simple terms, Bayesian estimation combines prior knowledge about a system with new data to improve the accuracy of model parameters.
Importance of Parameter Settings
Setting appropriate parameters is crucial for the accuracy and reliability of system identification using Bayesian estimation.
Parameters are essentially the variables that define the behavior and characteristics of the system.
Incorrect settings can lead to poor model performance or even the failure to identify the system correctly.
Key Points for Setting Parameters
Here we will explore some key points to consider when setting parameters in system identification using the Bayesian estimation method.
1. Understanding the System
Before setting any parameters, it’s important to have a comprehensive understanding of the system you’re dealing with.
This includes knowing the physical and mathematical features of the system, the constraints, and any external factors that can affect its behavior.
2. Defining Prior Information
Bayesian estimation relies heavily on prior information.
This includes any existing knowledge or assumptions you have about the system’s parameters.
Defining this prior information accurately is key to obtaining reliable estimations.
3. Choosing the Right Model
Selecting the right model is crucial in the parameter-setting process.
The chosen model should be capable of capturing the dynamics of the system accurately.
This involves testing different models and selecting the one that provides the best fit for your data.
4. Using Good Quality Data
The reliability of your system identification is highly dependent on the quality of the data used.
Ensure that the data is accurate and relevant to the system’s behavior.
Errors in data can significantly affect parameter estimation and lead to inaccurate results.
5. Determining the Parameter Range
When setting parameters, it’s essential to determine reasonable ranges for them.
This includes setting lower and upper limits based on system constraints and prior information.
Ensuring parameters fall within these ranges prevents unrealistic estimations and reflects a more accurate system model.
6. Iterative Refinement
Parameter estimation is rarely perfect on the first try.
It’s often an iterative process that involves refining and adjusting parameters until the model aligns closely with real-world observations.
This may require multiple rounds of estimation based on new data as it becomes available.
Challenges and Considerations
Despite following these key points, there are still challenges in parameter setting for Bayesian estimation.
Handling Uncertainty
Uncertainty is inherent in any system, and managing it is essential in parameter estimation.
Bayesian estimation helps manage uncertainty, but it’s crucial to remain mindful and adjust as new information emerges.
Balancing Complexity and Simplicity
There’s often a trade-off between having a complex system with many parameters and a simpler model that is easier to work with but might not capture all dynamics.
The key is to strike a balance that provides a realistic and practical system model.
Computational Resources
Bayesian estimation can be computationally intensive, especially for complex systems with numerous parameters.
Adequate computational resources are necessary to handle the required calculations efficiently.
Optimizing this aspect is crucial for timely and effective system identification.
Conclusion
Appropriate parameter settings in system identification using the Bayesian estimation method are essential for achieving accurate and reliable models.
By understanding the system, leveraging prior information, and iteratively refining parameters, you can effectively use Bayesian estimation to model dynamic systems.
Keeping in mind the challenges and factors involved in data quality, model selection, and computational resource management will further enhance the accuracy of your system identification efforts.
With these key points and considerations, you’re better equipped to master the art of parameter setting in system identification.
資料ダウンロード
QCD調達購買管理クラウド「newji」は、調達購買部門で必要なQCD管理全てを備えた、現場特化型兼クラウド型の今世紀最高の購買管理システムとなります。
ユーザー登録
調達購買業務の効率化だけでなく、システムを導入することで、コスト削減や製品・資材のステータス可視化のほか、属人化していた購買情報の共有化による内部不正防止や統制にも役立ちます。
NEWJI DX
製造業に特化したデジタルトランスフォーメーション(DX)の実現を目指す請負開発型のコンサルティングサービスです。AI、iPaaS、および先端の技術を駆使して、製造プロセスの効率化、業務効率化、チームワーク強化、コスト削減、品質向上を実現します。このサービスは、製造業の課題を深く理解し、それに対する最適なデジタルソリューションを提供することで、企業が持続的な成長とイノベーションを達成できるようサポートします。
オンライン講座
製造業、主に購買・調達部門にお勤めの方々に向けた情報を配信しております。
新任の方やベテランの方、管理職を対象とした幅広いコンテンツをご用意しております。
お問い合わせ
コストダウンが利益に直結する術だと理解していても、なかなか前に進めることができない状況。そんな時は、newjiのコストダウン自動化機能で大きく利益貢献しよう!
(Β版非公開)