- お役立ち記事
- Model selection from measurement data using Bayesian inference
月間76,176名の
製造業ご担当者様が閲覧しています*
*2025年3月31日現在のGoogle Analyticsのデータより
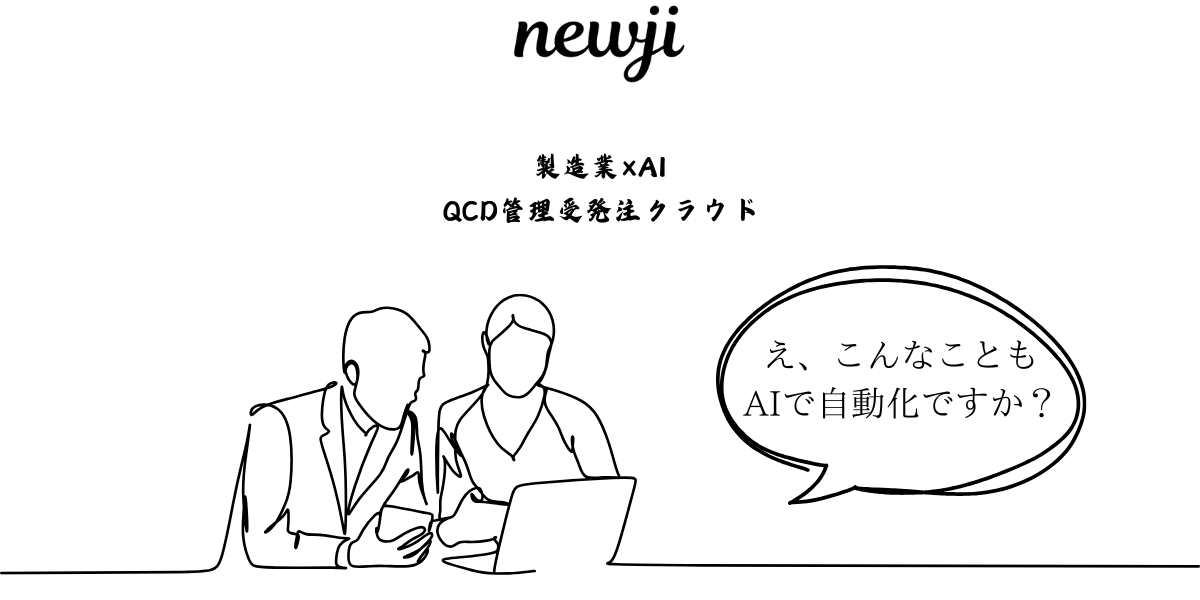
Model selection from measurement data using Bayesian inference
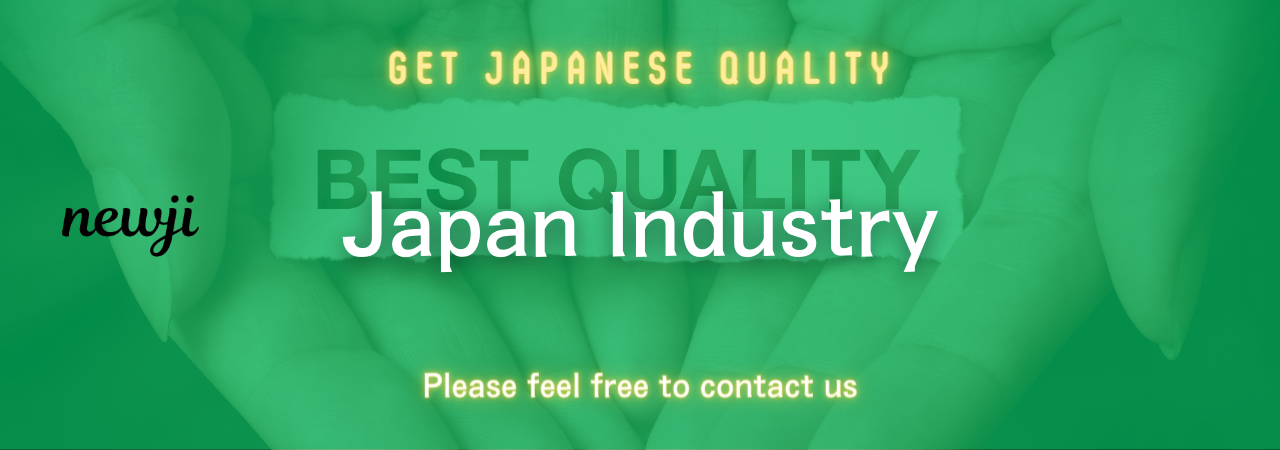
目次
Understanding Bayesian Inference
Bayesian inference is a statistical method that allows us to update our beliefs or models based on new evidence or data.
This method combines prior knowledge with current observations to make informed decisions.
It’s an approach widely used in various fields including science, engineering, and finance to tackle problems with uncertainty and incomplete information.
The key concept in Bayesian inference is the use of probabilities to represent uncertainty about model parameters.
The process starts by defining what we already know through a prior distribution.
Then, as new data becomes available, a likelihood function is used to measure the compatibility of the data under different model assumptions.
The result of Bayesian inference is the posterior distribution, which represents the updated beliefs after considering both the prior information and the new data.
Importance of Model Selection
In practice, model selection is a crucial aspect of data analysis, as choosing the right model can significantly influence the conclusions drawn from data.
Model selection involves identifying the model that best explains the observed data among a set of candidate models.
The aim is to balance the trade-off between model complexity and goodness of fit to the data.
Selecting an overly complex model might lead to overfitting, where the model captures noise rather than the underlying trend.
Conversely, a too-simple model may fail to capture important patterns in the data, leading to underfitting.
Bayesian inference provides a robust framework for model selection by evaluating models based on both their ability to fit the data and their complexity.
Using Bayesian Inference for Model Selection
Bayesian inference is particularly powerful for model selection due to its capacity to incorporate prior beliefs and its flexibility in comparing different models.
Several criteria within the Bayesian framework help implement model selection effectively, including the computation of the posterior probability of each model given the data.
An essential tool in Bayesian model selection is the Bayes factor.
The Bayes factor compares the predictive power of two models by looking at how well each model predicts the observed data.
Mathematically, it is the ratio of the marginal likelihoods of the models.
A higher Bayes factor indicates a preference for one model over another.
Bayes factors can also be interpreted qualitatively to guide model choice, often categorized by thresholds indicating evidence strength from weak to strong.
Another approach is the Bayesian Information Criterion (BIC), which approximates the Bayes factor.
The BIC introduces a penalty for the number of model parameters, thus discouraging overfitting by favoring simpler models with fewer parameters when the fit to the data is equivalent.
Priors and Their Role in Model Selection
The choice of priors is a critical aspect of Bayesian inference, particularly in the context of model selection.
Priors are mathematical expressions of what is known or assumed about the parameters before observing the data.
Carefully chosen priors can enhance the model’s performance by integrating relevant background knowledge and preventing overfitting, thus leading to more reliable model selection.
In contrast, improperly chosen priors may skew results and undermine the inference process.
In many cases, objective or non-informative priors are used when little prior information is available.
Such priors minimize the influence of previous assumptions to let the data speak for itself.
However, when meaningful prior information is at hand, informative priors can significantly improve the model selection process by guiding the analysis towards plausible parameter values.
Evaluating Model Performance
Once a model is selected using Bayesian inference, it’s important to evaluate its performance to ensure its suitability for making predictions or drawing conclusions.
Posterior predictive checks are a useful technique in this process, involving the simulation of new data from the posterior distribution and comparing it to the observed data.
These checks help to diagnose any discrepancies between the model and the data, indicating potential model misfit areas.
Cross-validation is another commonly used method for assessing model performance.
By partitioning data into training and testing sets, cross-validation evaluates the model’s ability to generalize to new, unseen data.
This step is crucial for models intended for predictive tasks, as it provides a safeguard against overfitting, ensuring the model’s predictions remain accurate outside the original dataset.
Benefits and Applications of Bayesian Model Selection
The advantages of Bayesian model selection lie in its transparency and interpretability.
By incorporating prior beliefs and quantifying uncertainty, it delivers more robust and comprehensive results than some traditional methods.
Moreover, Bayesian inference’s adaptability to handle various data types and structures makes it an attractive approach for a wide range of applications.
In medicine, Bayesian methods are used to select models for predicting patient outcomes based on historical data and clinical trials.
In finance, it assists in evaluating asset pricing models, considering both historical price movements and market assumptions.
In environmental science, Bayesian model selection helps in understanding complex systems, such as climate models, where data may be sparse or highly uncertain.
As more data becomes available, the reliance on sophisticated techniques like Bayesian inference continues to grow.
The approach not only equips analysts with powerful tools for selecting models but also provides a systematic way to manage uncertainty and integrate diverse types of information into the decision-making process.
In conclusion, using Bayesian inference for model selection offers a comprehensive and nuanced approach to understanding complex datasets.
By seamlessly integrating old knowledge with new data, it empowers researchers and professionals to make well-informed, defensible choices in model selection that are critical for accurate prediction and insight extraction.
資料ダウンロード
QCD管理受発注クラウド「newji」は、受発注部門で必要なQCD管理全てを備えた、現場特化型兼クラウド型の今世紀最高の受発注管理システムとなります。
ユーザー登録
受発注業務の効率化だけでなく、システムを導入することで、コスト削減や製品・資材のステータス可視化のほか、属人化していた受発注情報の共有化による内部不正防止や統制にも役立ちます。
NEWJI DX
製造業に特化したデジタルトランスフォーメーション(DX)の実現を目指す請負開発型のコンサルティングサービスです。AI、iPaaS、および先端の技術を駆使して、製造プロセスの効率化、業務効率化、チームワーク強化、コスト削減、品質向上を実現します。このサービスは、製造業の課題を深く理解し、それに対する最適なデジタルソリューションを提供することで、企業が持続的な成長とイノベーションを達成できるようサポートします。
製造業ニュース解説
製造業、主に購買・調達部門にお勤めの方々に向けた情報を配信しております。
新任の方やベテランの方、管理職を対象とした幅広いコンテンツをご用意しております。
お問い合わせ
コストダウンが利益に直結する術だと理解していても、なかなか前に進めることができない状況。そんな時は、newjiのコストダウン自動化機能で大きく利益貢献しよう!
(β版非公開)