- お役立ち記事
- Application to fluid performance optimization by combining the basics of fluid analysis/fluid simulation with machine learning
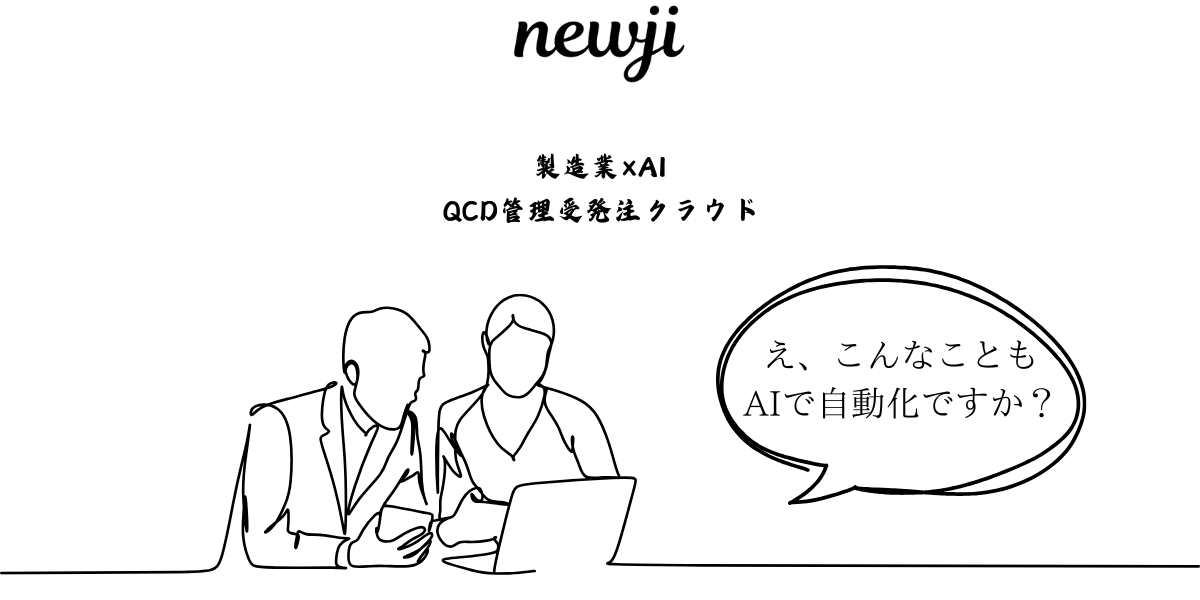
Application to fluid performance optimization by combining the basics of fluid analysis/fluid simulation with machine learning
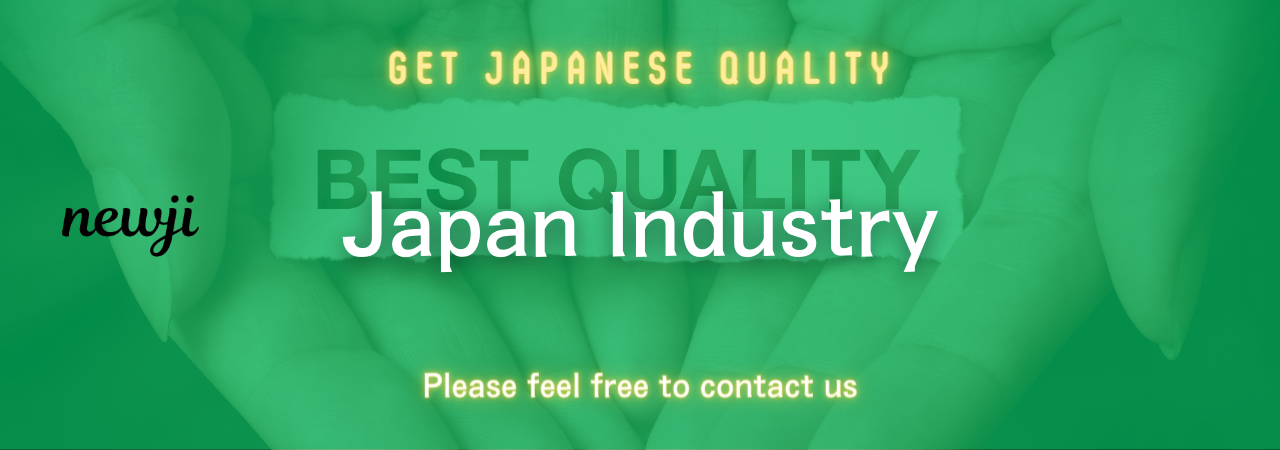
目次
Understanding Fluid Analysis and Simulation
Fluid analysis and fluid simulation are vital components in various engineering fields.
These techniques are used to predict how fluids (liquids and gases) behave under different conditions, which is crucial for designing efficient systems and devices.
The Basics of Fluid Analysis
Fluid analysis involves studying the behavior and properties of fluids in motion or at rest.
It helps in understanding how fluids interact with solid surfaces, how they transfer heat, and how they respond to various forces.
This analysis is essential in industries like aerospace, automotive, and chemical engineering, where fluid behavior impacts performance and safety.
Key Concepts in Fluid Simulation
Fluid simulation, on the other hand, uses mathematical models and computational tools to recreate fluid motions virtually.
This is done using complex equations like the Navier-Stokes equations, which describe how fluids move.
Simulations allow engineers to visualize and predict fluid behavior without needing to conduct costly and time-consuming physical experiments.
The Role of Machine Learning in Fluid Dynamics
Machine learning, a subset of artificial intelligence, has revolutionized how we approach fluid dynamics.
By training algorithms on large datasets, machine learning models can learn patterns and make predictions about fluid behavior more quickly and accurately than traditional methods.
Benefits of Combining Machine Learning with Fluid Simulation
Integrating machine learning with fluid simulation offers several advantages:
1. **Increased Efficiency**: Machine learning models can process vast amounts of data quickly, providing simulations results in a fraction of the time it would take using conventional methods.
2. **Improved Accuracy**: With continuous learning, these models can refine their predictions, leading to more accurate simulations that better reflect real-world scenarios.
3. **Cost-Effectiveness**: Reducing the need for physical prototypes and experiments lowers costs significantly, making it easier for companies to innovate.
4. **Scalability**: Machine learning algorithms can be scaled to handle more complex systems, making them versatile tools in fluid dynamics research.
Applications in Various Industries
The synergy between fluid simulation and machine learning extends across multiple industries.
In aerospace, for instance, it assists in designing more aerodynamic aircraft, reducing fuel consumption and emissions.
In the automotive sector, it aids in optimizing engine performance and improving the efficiency of cooling systems.
The maritime industry uses these advancements to design more efficient hulls, enabling ships to move faster and consume less energy.
Even in the medical field, machine learning-enhanced fluid simulations play a critical role in understanding blood flow through the human body, aiding in the development of better diagnostic and treatment techniques.
The Process of Optimizing Fluid Performance
Optimizing fluid performance means making systems more efficient, reducing energy consumption, and enhancing overall productivity.
This process involves several key steps.
Data Collection and Preprocessing
The first step involves gathering data from real-world experiments or existing simulations.
This data is then cleaned and preprocessed to ensure it is suitable for training machine learning models.
This step is crucial as the quality of data directly impacts the accuracy of predictions.
Model Selection and Training
Selecting the right machine learning model is fundamental to the success of the optimization process.
Models like neural networks, decision trees, or support vector machines may be used, depending on the complexity and nature of the fluid dynamics problem.
The model is then trained on the preprocessed data to learn the intricate patterns and relationships within the fluid dynamics system.
Validation and Testing
Once the model is trained, it must be validated and tested against new datasets to ensure its accuracy and reliability.
This phase helps in identifying any discrepancies and fine-tuning the model for improved performance.
Implementation and Continuous Improvement
Finally, the optimized simulation is implemented in the real-world application, where it is continuously monitored and updated.
Machine learning algorithms continue to learn and improve, adapting to changes and maintaining optimal performance over time.
Challenges and Future Prospects
Despite its advantages, the integration of machine learning with fluid dynamics also presents challenges.
The complexity of fluid behavior requires sophisticated algorithms, and ensuring the availability of high-quality data can be a hurdle.
Moreover, interpreting the outcomes of machine learning models can be difficult, necessitating specialized knowledge.
The Future of Fluid Dynamics and Machine Learning
As technology advances, the fusion of fluid dynamics and machine learning holds promise for even greater innovations.
Enhanced computational power and deeper understanding of machine learning models will likely lead to breakthroughs in both predictive accuracy and efficiency.
New applications, such as real-time simulations for autonomous vehicles or adaptive systems for climate control, are expected to emerge, further expanding the scope and impact of these technologies.
In conclusion, the convergence of fluid analysis, simulation, and machine learning is creating new paradigms in optimizing fluid performance.
This interdisciplinary approach is paving the way for more efficient, sustainable, and innovative solutions across various industries, holding the potential to transform how we understand and interact with fluid dynamics.
資料ダウンロード
QCD調達購買管理クラウド「newji」は、調達購買部門で必要なQCD管理全てを備えた、現場特化型兼クラウド型の今世紀最高の購買管理システムとなります。
ユーザー登録
調達購買業務の効率化だけでなく、システムを導入することで、コスト削減や製品・資材のステータス可視化のほか、属人化していた購買情報の共有化による内部不正防止や統制にも役立ちます。
NEWJI DX
製造業に特化したデジタルトランスフォーメーション(DX)の実現を目指す請負開発型のコンサルティングサービスです。AI、iPaaS、および先端の技術を駆使して、製造プロセスの効率化、業務効率化、チームワーク強化、コスト削減、品質向上を実現します。このサービスは、製造業の課題を深く理解し、それに対する最適なデジタルソリューションを提供することで、企業が持続的な成長とイノベーションを達成できるようサポートします。
オンライン講座
製造業、主に購買・調達部門にお勤めの方々に向けた情報を配信しております。
新任の方やベテランの方、管理職を対象とした幅広いコンテンツをご用意しております。
お問い合わせ
コストダウンが利益に直結する術だと理解していても、なかなか前に進めることができない状況。そんな時は、newjiのコストダウン自動化機能で大きく利益貢献しよう!
(Β版非公開)