- お役立ち記事
- Surrounding environment recognition technology, state estimation technology and their applications in autonomous driving
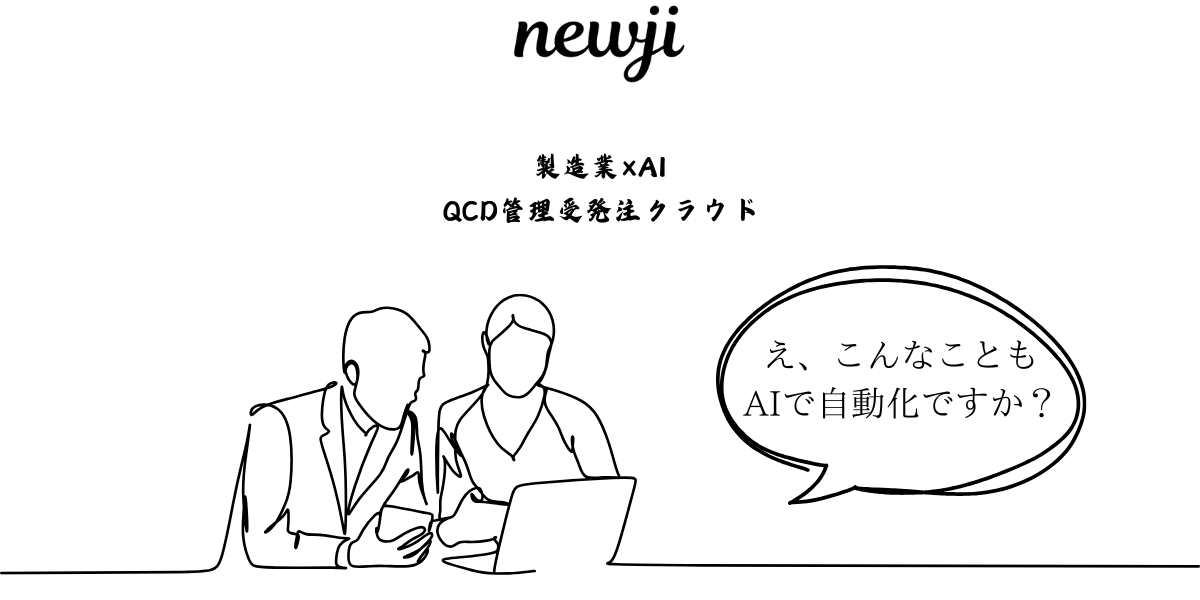
Surrounding environment recognition technology, state estimation technology and their applications in autonomous driving
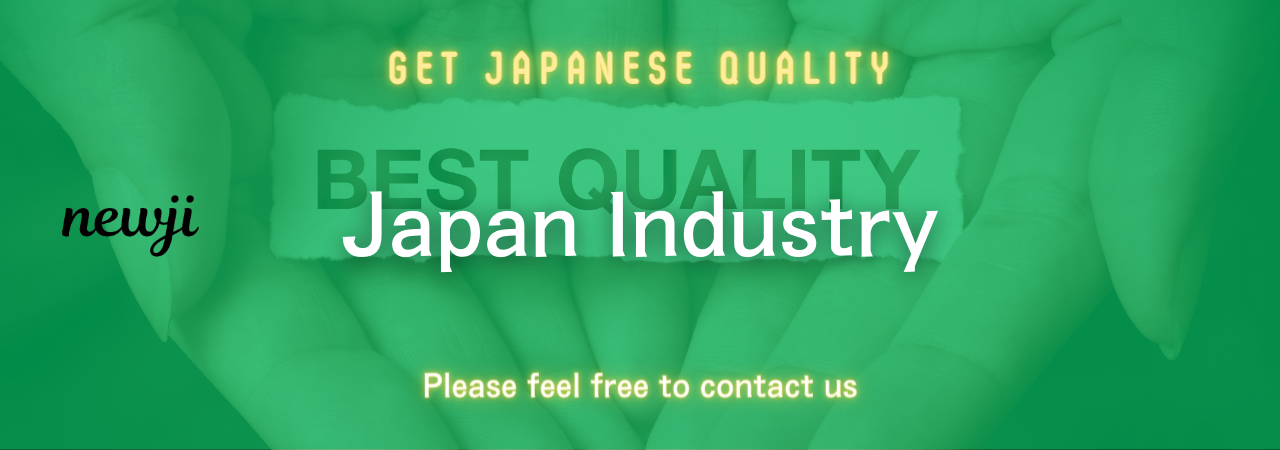
目次
Understanding Surrounding Environment Recognition
Surrounding environment recognition technology plays a crucial role in the development of autonomous driving systems.
This technology allows vehicles to perceive their surroundings through a combination of sensors and advanced algorithms.
Typically, a range of sensors such as cameras, LiDAR, radar, and ultrasonic sensors are used to collect data about the vehicle’s environment.
These sensors work in unison to detect and classify objects, assess distances, and identify potential road hazards.
One of the key tasks of environment recognition is object detection, which involves identifying and classifying other vehicles, pedestrians, cyclists, and obstacles in the vehicle’s path.
Modern autonomous systems employ machine learning algorithms to enhance the accuracy and robustness of object detection processes.
Deep learning models, especially convolutional neural networks, are commonly used to process enormous amounts of visual data, allowing the vehicle to recognize objects with precision and reliability.
Mapping and Localization
In addition to object detection, mapping and localization are fundamental aspects of surrounding environment recognition.
Autonomous vehicles must create a detailed map of their surroundings and constantly update it to reflect changes in the environment.
Mapping helps the vehicle understand its position relative to the world around it.
Localization, on the other hand, is the process of determining the vehicle’s exact position within the pre-determined map.
This typically relies on GPS data, but also integrates information from on-board sensors to improve accuracy.
Techniques such as simultaneous localization and mapping (SLAM) help vehicles construct maps of unknown environments while keeping track of their location.
Localization ensures that the vehicle can navigate efficiently and make informed decisions based on real-time environmental data.
Fusion of Sensor Data
A critical aspect of surroundings recognition in autonomous cars is the fusion of data from multiple sensors.
Each type of sensor provides unique information, and combining this data improves the reliability and accuracy of the system.
For instance, cameras can provide visual detail, while LiDAR offers precise distance measurements.
Data fusion algorithms process inputs from various sensors to create a comprehensive view of the environment.
By merging data from multiple sources, vehicles can overcome the limitations of individual sensors and make more informed driving decisions.
Sensor fusion not only enhances object detection and localization but also improves the vehicle’s ability to operate safely in challenging conditions, such as poor weather or low visibility.
The Role of State Estimation Technology
State estimation technology is another essential component in the realm of autonomous driving.
It involves predicting and estimating the internal state of the vehicle and its environment over time.
Understanding the state of a vehicle includes determining its speed, position, orientation, and acceleration.
Vehicle dynamics models are often employed to predict future states based on current inputs and environmental conditions.
Kalman filters, a common tool in state estimation, utilize mathematical models to provide real-time estimates of the vehicle’s state.
These filters continuously process sensor data and update predictions, ensuring the vehicle responds accurately to changes in the environment.
Path Planning and Decision Making
State estimation data feeds into the path planning and decision-making processes of autonomous vehicles.
Once a vehicle’s state is accurately determined, the system can plan a safe and efficient path through its surroundings.
Path planning involves finding the best route while avoiding obstacles, adhering to traffic rules, and ensuring passenger safety and comfort.
Sophisticated algorithms run simulations to evaluate different route options and choose the optimal path based on state estimation data.
Decision-making in autonomous driving is inherently complex, as it requires balancing multiple objectives, including safety, efficiency, and passenger experience.
Artificial intelligence and machine learning techniques often underlie these decision-making processes, allowing autonomous vehicles to adapt to real-time road conditions and make sound judgments.
Applications in Autonomous Driving
Surrounding environment recognition and state estimation technologies have wide-ranging applications in autonomous driving, contributing to safer and more efficient vehicle operations.
Enhancing Safety
By accurately recognizing their environment, autonomous vehicles are better equipped to avoid collisions with other vehicles, pedestrians, and obstacles.
Real-time data processing ensures that the vehicle can react instantly to sudden changes in the driving environment, significantly reducing the likelihood of accidents.
These technologies also play an important role in developing advanced driver-assistance systems (ADAS), which offer features like adaptive cruise control and emergency braking.
Improving Traffic Flow and Efficiency
Autonomous vehicles have the potential to enhance overall traffic flow and efficiency on roads.
By optimizing path planning and making data-driven decisions, these vehicles can reduce congestion and improve fuel efficiency.
Advanced state estimation and environment recognition enable vehicles to anticipate and adjust to traffic conditions, resulting in a smoother and more efficient transportation system.
Facilitating Autonomous Fleet Operations
Environment recognition and state estimation technologies are vital for the successful deployment of autonomous vehicle fleets.
These systems ensure that vehicles in a fleet can communicate and coordinate with one another, improving overall performance and efficiency.
Autonomous delivery services, ride-sharing, and public transportation can benefit immensely from these technologies by minimizing operational costs and maximizing service coverage.
Conclusion
Surrounding environment recognition and state estimation technologies are indispensable to the progress of autonomous driving.
By enabling vehicles to understand their surroundings and predict future states, these technologies pave the way for safer, more efficient, and reliable autonomous vehicles.
As advancements continue, we can expect significant improvements in traffic flow, safety, and the overall user experience in the world of autonomous driving.
資料ダウンロード
QCD調達購買管理クラウド「newji」は、調達購買部門で必要なQCD管理全てを備えた、現場特化型兼クラウド型の今世紀最高の購買管理システムとなります。
ユーザー登録
調達購買業務の効率化だけでなく、システムを導入することで、コスト削減や製品・資材のステータス可視化のほか、属人化していた購買情報の共有化による内部不正防止や統制にも役立ちます。
NEWJI DX
製造業に特化したデジタルトランスフォーメーション(DX)の実現を目指す請負開発型のコンサルティングサービスです。AI、iPaaS、および先端の技術を駆使して、製造プロセスの効率化、業務効率化、チームワーク強化、コスト削減、品質向上を実現します。このサービスは、製造業の課題を深く理解し、それに対する最適なデジタルソリューションを提供することで、企業が持続的な成長とイノベーションを達成できるようサポートします。
オンライン講座
製造業、主に購買・調達部門にお勤めの方々に向けた情報を配信しております。
新任の方やベテランの方、管理職を対象とした幅広いコンテンツをご用意しております。
お問い合わせ
コストダウンが利益に直結する術だと理解していても、なかなか前に進めることができない状況。そんな時は、newjiのコストダウン自動化機能で大きく利益貢献しよう!
(Β版非公開)