- お役立ち記事
- Fundamentals of hybrid AI and application to autonomous driving systems
月間77,185名の
製造業ご担当者様が閲覧しています*
*2025年2月28日現在のGoogle Analyticsのデータより
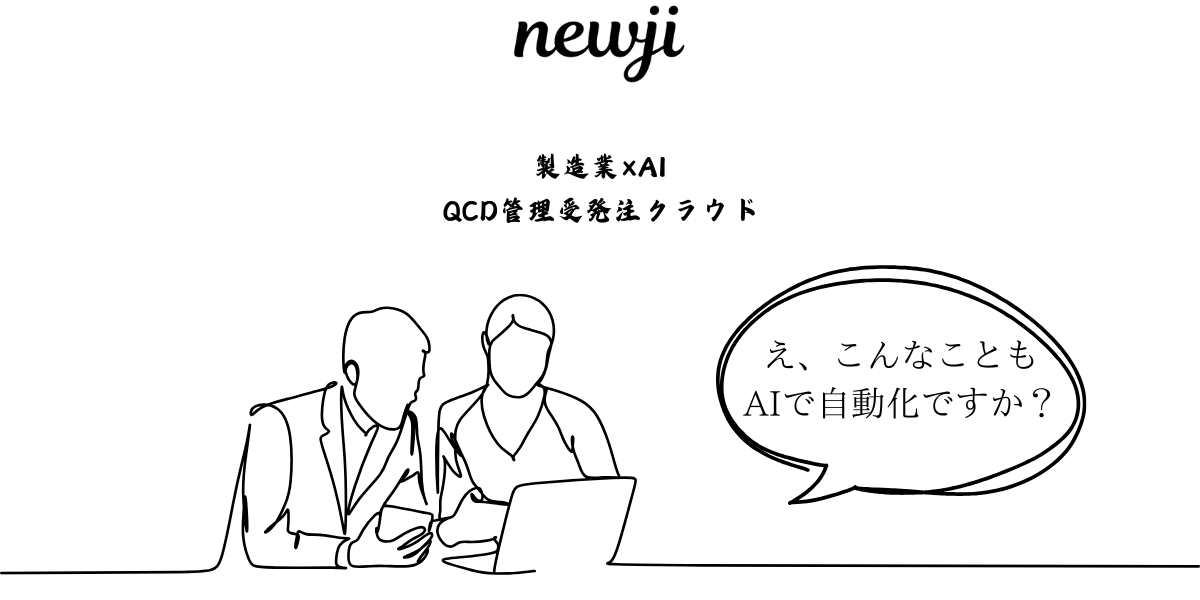
Fundamentals of hybrid AI and application to autonomous driving systems
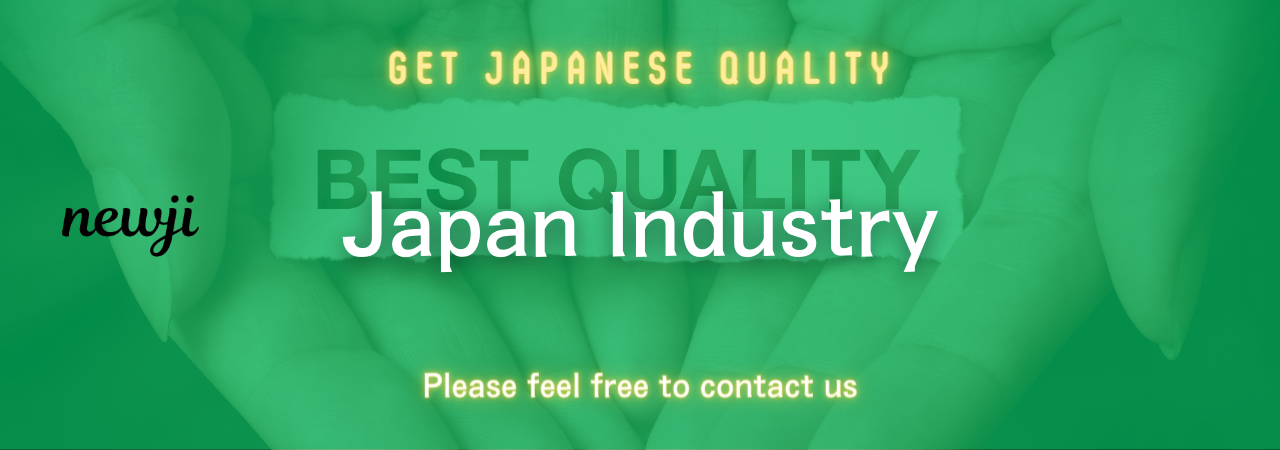
目次
Introduction to Hybrid AI
Hybrid AI, a sophisticated combination of different artificial intelligence methodologies, is paving the way for breakthroughs in technology and its applications, particularly in the field of autonomous driving systems.
Understanding the fundamentals of hybrid AI allows us to appreciate how it synthesizes various AI approaches to deliver more robust, efficient, and effective solutions.
Hybrid AI models integrate techniques from symbolic reasoning, machine learning, neural networks, genetic algorithms, and more to create systems that can adapt and learn in complex environments.
This type of AI is essential in systems where flexibility, real-time decision-making, and understanding of dynamic environments are crucial.
Components of Hybrid AI
To fully grasp hybrid AI, it is important to break down its core components and understand how they complement each other.
Symbolic AI
Symbolic AI, one of the earliest forms of artificial intelligence, is grounded in logic and rule-based systems.
These systems encode human expertise through predefined rules and logic statements.
Symbolic AI excels in tasks involving structured information and where explicit rules and representations are necessary.
Machine Learning
Machine Learning (ML), another pillar of hybrid AI, involves algorithms that enable systems to learn from data and make predictions or decisions without being explicitly programmed.
ML is particularly good at handling large volumes of data to identify patterns and correlations.
It is the backbone of many modern AI applications, contributing immensely to the adaptability and learning ability of hybrid AI.
Neural Networks
Neural Networks mimic the structure and functioning of the human brain, consisting of layers of interconnected nodes or “neurons.”
These networks are adept at handling complex, unstructured data and are a crucial component of deep learning frameworks, which drive advancements in image recognition, natural language processing, and more.
In hybrid AI, neural networks provide the capability to process and learn from vast and diverse datasets.
Integrating Components
The true power of hybrid AI lies in its ability to integrate these various components into a cohesive unit.
By combining symbolic reasoning with the data-oriented approaches of neural networks and machine learning, hybrid AI systems can leverage the strengths of each to overcome traditional AI limitations.
For instance, symbolic AI is deterministic and explainable, providing transparency in decision-making processes.
However, it struggles with scalability and adapting to new, unforeseen situations.
Machine learning and neural networks offer adaptability and are excellent at handling large, unstructured datasets but can lack transparency.
Hybrid AI models can therefore combine these traits to create a system that is both adaptable and explainable, a critical combination for applications such as autonomous driving.
Hybrid AI in Autonomous Driving Systems
Autonomous driving systems are complex environments where vehicles must interpret a wide array of data from sensors, maps, and onboard technology to navigate safely.
The blend of symbolic AI with neural networks and machine learning allows these systems to reason and make decisions like expert human drivers while learning from new experiences to improve over time.
Real-Time Decision Making
One of the most critical aspects of autonomous driving is real-time decision-making.
Vehicles must make split-second decisions based on a constant stream of data from various sensors, such as cameras, LiDAR, and radar.
Hybrid AI systems excel in this area by merging fast-processing neural networks with the precision and rule-bound nature of symbolic AI, ensuring that decisions are both quick and correct.
Obstacle Detection and Avoidance
Hybrid AI enhances obstacle detection and avoidance by combining pattern recognition capabilities of neural networks with logical reasoning from symbolic AI.
This combination allows vehicles to better understand complex scenes and make safe navigation decisions.
By interpreting sensor data in real-time, vehicles can recognize obstacles, predict their trajectory, and plan safe maneuvers to avoid them.
Route Planning and Optimization
Effective route planning in autonomous vehicles requires handling a vast array of data including traffic conditions, road types, weather, and more.
Hybrid AI systems employ machine learning algorithms to analyze real-time data and learn optimal travel routes, while symbolic AI ensures adherence to traffic rules and safety regulations.
This integration allows autonomous vehicles to efficiently plan routes that minimize time, fuel consumption, and risks.
Challenges and Future Directions
Despite its potential, implementing hybrid AI in autonomous driving systems faces several challenges.
These include ensuring data privacy and security, enhancing the interpretability of AI decisions, and resolving ethical concerns about decision-making in critical scenarios.
Ongoing research aims to address these issues, focusing on improving the interpretability and reliability of hybrid AI systems.
As technology advances, hybrid AI is expected to integrate more sophisticated algorithms and sensor technologies, improving autonomous systems’ ability to handle ever-changing environments.
Conclusion
Hybrid AI represents a significant leap forward in the development of adaptive, smart systems capable of handling the complex demands of autonomous driving.
By integrating the structure of symbolic AI with the learning capabilities of neural networks and machine learning, these systems achieve remarkable adaptability and reliability.
As the industry progresses, hybrid AI will undoubtedly continue to play a pivotal role in pushing the boundaries of what’s possible, building a future where autonomous vehicles are a common, safe, and trusted part of everyday life.
資料ダウンロード
QCD管理受発注クラウド「newji」は、受発注部門で必要なQCD管理全てを備えた、現場特化型兼クラウド型の今世紀最高の受発注管理システムとなります。
ユーザー登録
受発注業務の効率化だけでなく、システムを導入することで、コスト削減や製品・資材のステータス可視化のほか、属人化していた受発注情報の共有化による内部不正防止や統制にも役立ちます。
NEWJI DX
製造業に特化したデジタルトランスフォーメーション(DX)の実現を目指す請負開発型のコンサルティングサービスです。AI、iPaaS、および先端の技術を駆使して、製造プロセスの効率化、業務効率化、チームワーク強化、コスト削減、品質向上を実現します。このサービスは、製造業の課題を深く理解し、それに対する最適なデジタルソリューションを提供することで、企業が持続的な成長とイノベーションを達成できるようサポートします。
製造業ニュース解説
製造業、主に購買・調達部門にお勤めの方々に向けた情報を配信しております。
新任の方やベテランの方、管理職を対象とした幅広いコンテンツをご用意しております。
お問い合わせ
コストダウンが利益に直結する術だと理解していても、なかなか前に進めることができない状況。そんな時は、newjiのコストダウン自動化機能で大きく利益貢献しよう!
(β版非公開)